if crop == 'Beans' && any(abs(year - beanYears(fieldId)) < 3) error('豆类作物三年内已种植过'); end修改错误
时间: 2024-09-07 09:01:06 浏览: 85
这段代码似乎是用于检查某种作物是否在近三年内种植过,并在是的情况下发出错误提示。不过,代码中存在一些逻辑上的问题,因为 `any` 函数通常用于判断数组中是否至少有一个元素满足条件,而 `abs(year - beanYears(fieldId)) < 3` 这个条件判断通常不适用于 `any` 函数。正确的逻辑可能需要检查特定字段是否在过去的三年内被设置过。
这里是一个修改后的逻辑示例,假设 `beanYears` 函数返回一个数组,包含了过去三年内种植豆类作物的年份:
```matlab
if crop == 'Beans'
recentYears = year - beanYears(fieldId);
if any(recentYears >= 0 && recentYears < 3)
error('豆类作物三年内已种植过');
end
end
```
这里的修改点包括:
1. 移除了 `==` 判断符号后的 `&&`,直接使用 `&&` 连接两个条件。
2. 使用 `any` 函数来检查 `recentYears` 数组中是否有元素满足 `>= 0 && < 3` 的条件。
3. 修改了条件判断的逻辑,确保只有在过去的三年内种植过豆类作物的情况下,才会触发错误提示。
相关问题
如何编写python代码解决裁剪数据集后训练出现错误cv2.error,top>=0 &&bottom>=0 &&left>=0 &&right>=0
这个错误通常是由于裁剪后的图像坐标超出了原图像的范围所导致的。可以通过以下代码来避免这个问题:
```python
import cv2
# 读取原始图像
img = cv2.imread("image.jpg")
# 定义裁剪区域
x, y, w, h = 100, 100, 200, 200
# 确保裁剪区域在图像范围内
x = max(0, x)
y = max(0, y)
w = min(w, img.shape[1]-x)
h = min(h, img.shape[0]-y)
# 裁剪图像
crop_img = img[y:y+h, x:x+w]
```
在代码中,`max(0, x)`和`max(0, y)`用于确保裁剪区域不会超出图像的左上角边界,`min(w, img.shape[1]-x)`和`min(h, img.shape[0]-y)`用于确保裁剪区域不会超出图像的右下角边界。这样就可以避免出现`cv2.error`错误了。
def crop_pointcloud(data_crop, x_o, y_o, x_i, y_i, R_o, R_i, z_critical): K_o = R_o ** 2 / range_z K_i = R_i ** 2 / range_z for z in range(range_z): r_o = np.sqrt(z * K_o) data_layer = data_crop[:, :, z] d_o = np.sqrt(x_o ** 2 + y_o ** 2) d_i = np.sqrt(x_i ** 2 + y_i ** 2) if z < z_critical: r_i = 0 else: r_i = np.sqrt(z * K_i) data_crop[:, :, z] = np.where((d_o > r_o) | (d_i <= r_i), 0, data_layer) return data_crop data_crop = data[:, :, :400] range_x, range_y, range_z = data_crop.shape dx = 550 dy = 530 x, y = np.meshgrid(np.arange(range_x), np.arange(range_y)) x_o = x - range_x / 2 y_o = y - range_y / 2 x_i = x - dx y_i = y - dy z_critical = 50 R_o = 550 R_i = 200 data_crop = crop_pointcloud(data_crop, x_o, y_o, x_i, y_i, R_o, R_i, z_critical) data_crop = data_crop[:, :, 10:]转c++
这段 Python 代码主要是对点云数据进行裁剪,并返回裁剪后的点云数据。以下是将其转换为 C++ 的代码:
```cpp
#include <cmath>
#include <vector>
#include <algorithm>
void crop_pointcloud(std::vector<std::vector<std::vector<float>>>& data_crop,
int x_o, int y_o, int x_i, int y_i, int R_o, int R_i, int z_critical)
{
int range_x = data_crop.size();
int range_y = data_crop[0].size();
int range_z = data_crop[0][0].size();
float K_o = std::pow(R_o, 2) / range_z;
float K_i = std::pow(R_i, 2) / range_z;
for (int z = 0; z < range_z; ++z) {
float r_o = std::sqrt(z * K_o);
std::vector<std::vector<float>>& data_layer = data_crop[z];
float d_o = std::sqrt(std::pow(x_o, 2) + std::pow(y_o, 2));
float d_i = std::sqrt(std::pow(x_i, 2) + std::pow(y_i, 2));
float r_i = (z < z_critical) ? 0 : std::sqrt(z * K_i);
for (int i = 0; i < range_x; ++i) {
for (int j = 0; j < range_y; ++j) {
if (d_o > r_o || d_i <= r_i) {
data_layer[i][j] = 0;
}
}
}
}
}
int main()
{
std::vector<std::vector<std::vector<float>>> data_crop; // 假设已经读入了点云数据
int range_x = data_crop.size();
int range_y = data_crop[0].size();
int range_z = data_crop[0][0].size();
int dx = 550;
int dy = 530;
int x_o, y_o, x_i, y_i;
x_o = x_i = range_x / 2;
y_o = y_i = range_y / 2;
int z_critical = 50;
int R_o = 550;
int R_i = 200;
crop_pointcloud(data_crop, x_o, y_o, x_i, y_i, R_o, R_i, z_critical);
// 剩余部分请自行完成
return 0;
}
```
需要注意的是,在 C++ 中使用多维数组需要使用嵌套的 `std::vector`,因此在 C++ 的实现中需要对数组的访问进行适当修改。此外,需要将 `numpy` 的一些函数,比如 `np.arange` 和 `np.where` 等等,转换为 C++ 中的等价函数。
阅读全文
相关推荐
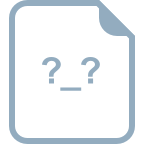
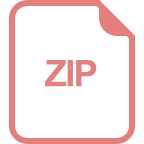
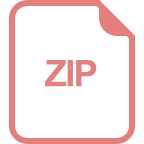
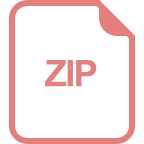
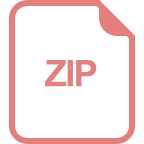
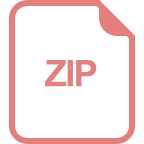
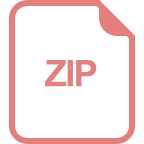
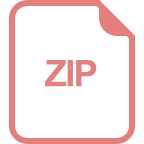
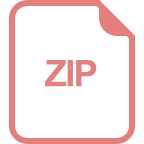
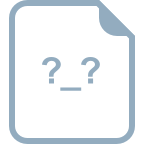
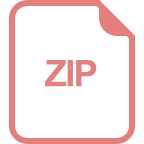


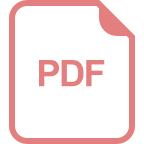
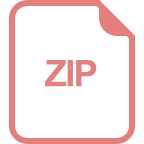
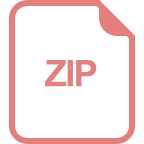