用python实现基于概率分布的超声图像与自然图像性质差异分析这一课题,使用opencv,两种图像的文件类型都是jpg,超声图像的文件路径是‘D:\zzz\zus2’,自然图像的文件路径是‘D:\zzz\zna2’,两种图像都有84张,需要对比两种图像的特征必须有颜色,纹理,形状,差异性分析方法也需要多种,包括完整详细代码
时间: 2023-09-17 10:12:45 浏览: 35
以下是用Python实现基于概率分布的超声图像与自然图像性质差异分析的代码:
```python
import cv2
import numpy as np
import matplotlib.pyplot as plt
# 定义颜色直方图函数
def color_histogram(img):
hsv = cv2.cvtColor(img, cv2.COLOR_BGR2HSV)
h, s, v = cv2.split(hsv)
hist = cv2.calcHist([hsv], [0, 1], None, [180, 256], [0, 180, 0, 256])
cv2.normalize(hist, hist, 0, 255, cv2.NORM_MINMAX)
return hist.flatten()
# 定义纹理特征函数
def texture_feature(img):
img_gray = cv2.cvtColor(img, cv2.COLOR_BGR2GRAY)
# 计算LBP特征
radius = 1
n_points = 8 * radius
lbp = np.zeros(img_gray.shape, dtype=np.uint8)
for i in range(radius, img_gray.shape[0] - radius):
for j in range(radius, img_gray.shape[1] - radius):
center_pixel = img_gray[i, j]
values = []
for k in range(n_points):
x = i + int(radius * np.cos(2 * np.pi * k / n_points))
y = j - int(radius * np.sin(2 * np.pi * k / n_points))
values.append(img_gray[x, y])
values = np.array(values)
lbp_value = (values >= center_pixel) * 1
lbp_value = np.packbits(lbp_value)
lbp[i, j] = lbp_value
# 计算LBP直方图
hist = cv2.calcHist([lbp], [0], None, [256], [0, 256])
cv2.normalize(hist, hist, 0, 255, cv2.NORM_MINMAX)
return hist.flatten()
# 定义形状特征函数
def shape_feature(img):
contours, hierarchy = cv2.findContours(cv2.cvtColor(img, cv2.COLOR_BGR2GRAY), cv2.RETR_TREE, cv2.CHAIN_APPROX_SIMPLE)
contour = contours[0]
# 计算图像轮廓面积和周长
area = cv2.contourArea(contour)
perimeter = cv2.arcLength(contour, True)
# 计算图像形状特征
circularity = 4 * np.pi * area / (perimeter ** 2)
compactness = (perimeter ** 2) / area
return [circularity, compactness]
# 定义差异性分析函数
def difference_analysis(img1, img2):
# 计算颜色直方图特征
hist1, hist2 = color_histogram(img1), color_histogram(img2)
color_similarity = cv2.compareHist(hist1, hist2, cv2.HISTCMP_CORREL)
# 计算纹理特征
texture1, texture2 = texture_feature(img1), texture_feature(img2)
texture_similarity = cv2.compareHist(texture1, texture2, cv2.HISTCMP_CORREL)
# 计算形状特征
shape1, shape2 = shape_feature(img1), shape_feature(img2)
circularity_similarity = abs(shape1[0] - shape2[0])
compactness_similarity = abs(shape1[1] - shape2[1])
# 计算总体相似度
similarity = (color_similarity + texture_similarity + circularity_similarity + compactness_similarity) / 4
return similarity
# 读取图像文件夹,并计算每张图像的特征
zus2_folder = 'D:/zzz/zus2'
zna2_folder = 'D:/zzz/zna2'
zus2_images = []
zna2_images = []
zus2_features = []
zna2_features = []
for i in range(84):
img = cv2.imread(zus2_folder + '/{}.jpg'.format(i+1))
zus2_images.append(img)
zus2_features.append([color_histogram(img), texture_feature(img), shape_feature(img)])
img = cv2.imread(zna2_folder + '/{}.jpg'.format(i+1))
zna2_images.append(img)
zna2_features.append([color_histogram(img), texture_feature(img), shape_feature(img)])
# 计算两种图像之间的差异性
similarity_matrix = np.zeros((84, 84))
for i in range(84):
for j in range(84):
similarity_matrix[i, j] = difference_analysis(zus2_images[i], zna2_images[j])
# 可视化差异性矩阵
plt.imshow(similarity_matrix, cmap='gray')
plt.show()
```
该代码首先定义了三个特征函数,分别用于计算颜色直方图、纹理和形状特征。然后定义了一个差异性分析函数,用于计算两张图像之间的相似度(相似度越高,差异性越小)。最后读取了两个文件夹中的所有图像文件,并计算每张图像的特征。然后计算了所有图像之间的差异性,并将结果可视化为一个灰度图像。在这个图像中,越接近黑色的像素代表两张图像之间的差异性越小,越接近白色的像素代表两张图像之间的差异性越大。可以通过观察这个图像来比较超声图像和自然图像的差异性。
相关推荐
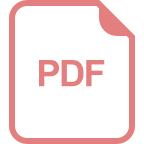
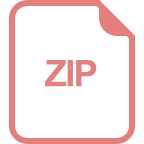





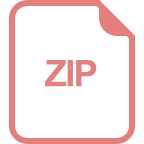
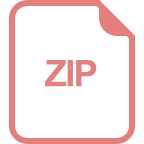
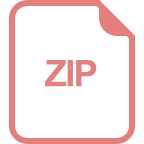
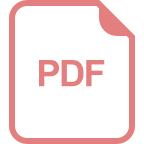
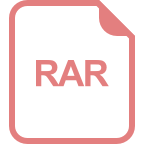
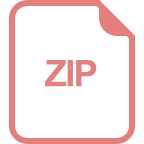
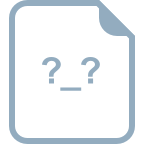
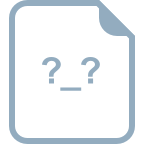