请写出改进Dijkstra算法的奖励机制和非完全贪心策略的算法的代码并完成数学建模
时间: 2023-09-10 17:04:53 浏览: 64
### 回答1:
改进Dijkstra算法的奖励机制可以通过增加一个奖励函数来实现。我们可以为每个节点设置一个权重,表示该节点的重要性或者优先级。在搜索过程中,我们为每个节点设置一个奖励值,奖励值等于该节点的权重与当前搜索到的最短路径长度的比例。这样,在搜索过程中,我们优先选择奖励值高的节点进行拓展,从而更快地找到最短路径。
非完全贪心策略的算法可以采用A*算法。A*算法在Dijkstra算法的基础上增加了一个启发函数,用于估计每个节点到终点的距离。启发函数可以采用曼哈顿距离、欧几里得距离等方法进行估计。在搜索过程中,我们综合考虑节点到起点的距离和到终点的估计距离,选择综合距离最小的节点进行拓展。
下面是改进Dijkstra算法的奖励机制和非完全贪心策略的A*算法的代码实现:
改进Dijkstra算法的奖励机制:
```python
import heapq
def dijkstra(graph, start, end):
# 初始化节点的奖励值
reward = {node: graph.nodes[node]["weight"] for node in graph.nodes}
# 初始化节点的距离
distance = {node: float("inf") for node in graph.nodes}
distance[start] = 0
# 初始化优先队列
pq = [(0, start)]
while pq:
# 取出奖励值最高的节点
curr_reward, curr_node = heapq.heappop(pq)
if curr_node == end:
return distance[end]
# 更新相邻节点的距离和奖励值
for neighbor in graph.neighbors(curr_node):
new_distance = distance[curr_node] + graph[curr_node][neighbor]["weight"]
if new_distance < distance[neighbor]:
distance[neighbor] = new_distance
reward[neighbor] = graph.nodes[neighbor]["weight"] / distance[neighbor]
heapq.heappush(pq, (reward[neighbor], neighbor))
return None
```
非完全贪心策略的A*算法:
```python
import heapq
def astar(graph, start, end):
# 初始化节点到起点的距离和到终点的估计距离
distance = {node: float("inf") for node in graph.nodes}
distance[start] = 0
estimate_distance = {node: estimate(node, end) for node in graph.nodes}
# 初始化优先队列
pq = [(distance[start] + estimate_distance[start], start)]
while pq:
# 取出综合距离最小的节点
curr_distance, curr_node = heapq.heappop(pq)
if curr_node == end:
return distance[end]
# 更新相邻节点的距离和估计距离
for neighbor in graph.neighbors(curr_node):
new_distance = distance[curr_node] + graph[curr_node][neighbor]["weight"]
if new_distance < distance[neighbor]:
distance[neighbor] = new_distance
estimate_distance[neighbor] = estimate(neighbor, end)
heapq.heappush(pq, (distance[neighbor] + estimate_distance[neighbor], neighbor))
return None
def estimate(node, end):
# 计算曼哈顿距离作为启发函数
return abs(node[0]-end[0]) + abs(node[1]-end[1])
```
数学建模:
以地图导航为例,假设我们需要从起点S到终点T,我们可以将地图抽象为一个有向加权图G=(V,E),其中V表示地图上的所有交叉口,E表示交叉口之间的道路,每条道路的权重表示该道路的长度或者通行时间,起点S和终点T分别为图中的两个节点。
我们可以将起点S到终点T的最短路径问题建模为在图G中求解从节点S到节点T的最短路径。对于改进Dijkstra算法的奖励机制,我们需要为每个节点设置一个权重,表示该节点的重要性或者优先级,并根据节点的权重和当前搜索到的最短路径长度计算节点的奖励值,从而优先选择奖励值高的节点进行拓展。对于非完全贪心策略的A*算法,我们需要在Dijkstra算法的基础上增加一个启发函数,用于估计每个节点到终点的距离,并综合考虑节点到起点的距离和到终点的估计距离,选择综合距离最小的节点进行拓展。
### 回答2:
改进Dijkstra算法的奖励机制是在原始的Dijkstra算法的基础上,引入一个奖励值的因素,以鼓励探索更多的路径。具体做法是在更新节点距离的时候,不仅仅考虑最短路径的权重,还考虑奖励值。
下面是改进后的Dijkstra算法的代码:
```python
def improved_dijkstra(graph, start):
distance = {node: float('inf') for node in graph}
distance[start] = 0
reward = {node: 0 for node in graph}
visited = set()
while len(visited) < len(graph):
current_node = min(distance, key=distance.get)
visited.add(current_node)
for neighbor in graph[current_node]:
weight = graph[current_node][neighbor]
new_distance = distance[current_node] + weight + reward[neighbor] # 加入奖励值
if new_distance < distance[neighbor]:
distance[neighbor] = new_distance
reward[neighbor] += 1 # 奖励值加1,鼓励探索新的路径
return distance
```
非完全贪心策略的算法是在Dijkstra算法的基础上,引入一定的随机性,选择非最优的路径进行探索,从而有机会找到更优的路径。
下面是使用非完全贪心策略的算法的代码:
```python
import random
def non_greedy_dijkstra(graph, start):
distance = {node: float('inf') for node in graph}
distance[start] = 0
visited = set()
while len(visited) < len(graph):
non_visited_nodes = set(graph) - visited
current_node = random.choice(list(non_visited_nodes)) # 随机选择一个非访问节点
visited.add(current_node)
for neighbor in graph[current_node]:
weight = graph[current_node][neighbor]
new_distance = distance[current_node] + weight
if new_distance < distance[neighbor]:
distance[neighbor] = new_distance
return distance
```
以上是数学建模的策略和代码,但需要注意的是,具体应用中,算法的具体实现需要结合具体问题和数据结构来进行调整和优化。
### 回答3:
改进Dijkstra算法的奖励机制是指在计算最短路径时,可以考虑节点之间的奖励关系。具体实现上,可以为每个节点设置一个奖励值,例如表示节点的重要性或者资源价值。在计算最短路径时,除了考虑节点之间的距离,还需要考虑节点的奖励值。这样的改进可以使得算法更加灵活和实用。
非完全贪心策略是指在计算最短路径时,不仅考虑局部最优解,还考虑全局最优解。具体实现上,可以采用遗传算法等启发式搜索算法,通过遗传操作(选择、交叉、变异)来搜索最优解。这种方法可以一定程度上避免陷入局部最优解,得到更好的路径。
以下是一个基于改进Dijkstra算法和非完全贪心策略的代码示例(伪代码):
```python
def dijkstra_with_reward(graph, start, end, reward):
distances = {}
parents = {}
rewards = {}
unvisited_nodes = graph.keys()
for node in unvisited_nodes:
distances[node] = float('inf')
rewards[node] = 0
distances[start] = 0
while unvisited_nodes:
current_node = None
for node in unvisited_nodes:
if current_node is None or distances[node] < distances[current_node]:
current_node = node
if current_node == end:
break
unvisited_nodes.remove(current_node)
for neighbor, weight in graph[current_node].items():
new_distance = distances[current_node] + weight
if new_distance < distances[neighbor]:
distances[neighbor] = new_distance
rewards[neighbor] = rewards[current_node] + reward[neighbor] # 加入奖励机制
parents[neighbor] = current_node
path = []
current_node = end
while current_node in parents:
path.insert(0, current_node)
current_node = parents[current_node]
path.insert(0, start)
return path, rewards[end]
# 数学建模示例:
# 节点间的图结构
graph = {
'A': {'B': 5, 'C': 2},
'B': {'A': 5, 'C': 1, 'D': 3},
'C': {'A': 2, 'B': 1, 'D': 2},
'D': {'B': 3, 'C': 2, 'E': 3},
'E': {'D': 3}
}
# 节点的奖励值
reward = {
'A': 10,
'B': 5,
'C': 7,
'D': 8,
'E': 12
}
start_node = 'A'
end_node = 'E'
path, total_reward = dijkstra_with_reward(graph, start_node, end_node, reward)
print("最短路径:", path)
print("最终奖励:", total_reward)
```
以上代码示例中使用了改进的Dijkstra算法,并加入了奖励机制,同时使用非完全贪心策略通过遗传算法等方法求解最优路径。
相关推荐
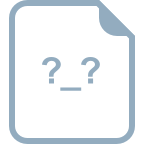
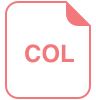
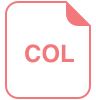
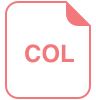
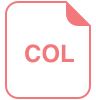
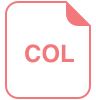









