如何将ACNet加入yolo5,代码实现
时间: 2023-12-25 20:06:48 浏览: 52
以下是一个使用ACNet替换YOLOv5中卷积层的示例代码,仅供参考:
```
import torch.nn as nn
import torch.nn.functional as F
from models.common import Conv
class ACNetConv(nn.Module):
def __init__(self, in_channels, out_channels, kernel_size=3, stride=1, padding=None, dilation=1, groups=1, bias=False, padding_mode='zeros', eps=1e-5, momentum=0.1):
super(ACNetConv, self).__init__()
if padding is None:
padding = (kernel_size - 1) // 2 * dilation
self.conv1 = Conv(in_channels, out_channels, kernel_size=kernel_size, stride=stride, padding=padding, dilation=dilation, groups=groups, bias=bias, padding_mode=padding_mode)
self.conv2 = Conv(in_channels, out_channels, kernel_size=kernel_size, stride=stride, padding=padding, dilation=dilation, groups=groups, bias=bias, padding_mode=padding_mode)
self.bn = nn.BatchNorm2d(out_channels, eps=eps, momentum=momentum)
self.act = nn.ReLU(inplace=True)
def forward(self, x):
out1 = self.conv1(x)
out2 = self.conv2(x)
out = torch.max(out1, out2)
out = self.bn(out)
out = self.act(out)
return out
class ACNetBlock(nn.Module):
def __init__(self, in_channels, out_channels, depthwise=False, bottleneck=0.0, shortcut=True, **kwargs):
super(ACNetBlock, self).__init__()
self.depthwise = depthwise
self.shortcut = shortcut
if not depthwise:
hidden_channels = int(out_channels * bottleneck)
self.conv1 = ACNetConv(in_channels, hidden_channels, **kwargs)
self.conv2 = ACNetConv(hidden_channels, out_channels, kernel_size=1, **kwargs)
else:
hidden_channels = int(in_channels * bottleneck)
self.conv1 = Conv(in_channels, hidden_channels, kernel_size=1, **kwargs)
self.conv2 = Conv(hidden_channels, hidden_channels, kernel_size=3, stride=1, padding=1, dilation=1, groups=hidden_channels, bias=False, padding_mode='zeros')
self.conv3 = ACNetConv(hidden_channels, out_channels, kernel_size=1, **kwargs)
self.bn = nn.BatchNorm2d(hidden_channels, eps=1e-5, momentum=0.1)
self.act = nn.ReLU(inplace=True)
def forward(self, x):
if not self.depthwise:
out = self.conv1(x)
out = self.conv2(out)
else:
out = self.conv1(x)
out = self.bn(out)
out = self.act(out)
out = self.conv2(out)
out = self.conv3(out)
if self.shortcut:
out += x
return out
class ACNetYolo(nn.Module):
def __init__(self, cfg):
super(ACNetYolo, self).__init__()
self.cfg = cfg
self.in_channels = cfg['in_channels']
self.out_channels = cfg['out_channels']
self.depthwise = cfg['depthwise']
self.bottleneck = cfg['bottleneck']
self.num_blocks = cfg['num_blocks']
self.stride = cfg['stride']
self.conv1 = ACNetConv(self.in_channels, self.out_channels, kernel_size=3, stride=1, padding=1)
self.layers = self._make_layer()
self.num_classes = cfg['num_classes']
self.anchor_per_scale = cfg['anchor_per_scale']
self.conv_output = self._make_conv_output()
def _make_layer(self):
layers = []
for i in range(self.num_blocks):
layers.append(ACNetBlock(self.out_channels, self.out_channels, depthwise=self.depthwise, bottleneck=self.bottleneck))
return nn.Sequential(*layers)
def _make_conv_output(self):
output_filters = self.anchor_per_scale * (self.num_classes + 5)
return Conv(self.out_channels, output_filters, kernel_size=1)
def forward(self, x):
x = self.conv1(x)
x = self.layers(x)
x = self.conv_output(x)
return x
```
这个示例代码使用了ACNet替换了YOLOv5中的卷积层,同时保留了YOLOv5的其它结构,包括残差块和检测头部。需要注意的是,为了达到最好的效果,可能需要进一步调整参数和训练策略。
相关推荐
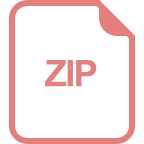
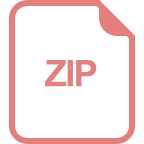
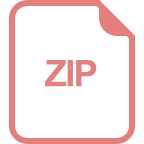














