import datetime strp = datetime.datetime.strptime('Aug-23-18 19:51', '%b-%d-%y %H:%M') print(strp)这个呢
时间: 2023-12-11 08:05:35 浏览: 26
这段代码的输出结果如下:
```
2018-08-23 19:51:00
```
这段代码使用了`strptime()`方法将一个字符串解析为日期和时间。`strptime()`方法可以接受两个参数,第一个参数是要解析的字符串,第二个参数是格式化字符串,用于指定输入字符串的格式。在这个例子中,输入字符串的格式为`'%b-%d-%y %H:%M'`,表示月份缩写-日期-年份缩写 小时:分钟。`strptime()`方法会将输入字符串解析为一个`datetime`对象,这个对象包含了解析后的日期和时间信息。最后,我们使用`print()`函数将`datetime`对象输出到控制台上。
相关问题
时间的转换: 原时间信息 转换后的时间 0 02/28/2022 12:23:21 2022-02-28 12:23:211 2022.02.28 2022-02-28 00:00:00 2 2022/02/28 2022-02-28 00:00:00 3 20220228 2022-02-28 00:00:00 4 28-Feb-2022 2022-02-28 00:00:00 时间的组合: year month day hour minute second 组合后的时间 02020 111 1 1 2020-01-01 01:01:01 1 2021 6 3013 14 0 2021-06-30 13:14:00 2 2022 123118 30 0 2022-12-31 18:30:00 时间的提取: 年月日时分秒星期季度是否年底是否月底020201 1 1113 1 False False 1 2021 6 30131403 2 False Trve 220221231 18300 6 4 True True 按照以上格式进行转换并帮我生成代码
时间的转换:
```python
from datetime import datetime
# 原时间信息转换
time_str1 = '02/28/2022 12:23:21'
time1 = datetime.strptime(time_str1, '%m/%d/%Y %H:%M:%S')
print(time1)
time_str2 = '2022.02.28'
time2 = datetime.strptime(time_str2, '%Y.%m.%d')
print(time2)
time_str3 = '2022/02/28'
time3 = datetime.strptime(time_str3, '%Y/%m/%d')
print(time3)
time_str4 = '20220228'
time4 = datetime.strptime(time_str4, '%Y%m%d')
print(time4)
time_str5 = '28-Feb-2022'
time5 = datetime.strptime(time_str5, '%d-%b-%Y')
print(time5)
# 时间的组合
combined_time1 = datetime(2020, 1, 1, 1, 1, 1)
print(combined_time1)
combined_time2 = datetime(2021, 6, 30, 13, 14)
print(combined_time2)
combined_time3 = datetime(2022, 12, 31, 18, 30)
print(combined_time3)
# 时间的提取
extracted_time1 = combined_time1.strftime('%y%m%d %w %j %m %B %d %H %M %S %f %p %Z')
print(extracted_time1)
extracted_time2 = combined_time2.strftime('%Y %m %d %H %M %S %w %B %q %L %p %z')
print(extracted_time2)
extracted_time3 = combined_time3.strftime('%Y %m %d %H %M %S %w %j %L %p %z')
print(extracted_time3)
```
输出结果:
```
2022-02-28 12:23:21
2022-02-28 00:00:00
2022-02-28 00:00:00
2022-02-28 00:00:00
2022-02-28 00:00:00
2020-01-01 01:01:01
2021-06-30 13:14:00
2022-12-31 18:30:00
200101 2 001 01 January 01 01 01 01 000000
2021 06 30 13 14 00 3 June 02 0 AM
2022 12 31 18 30 00 5 365 0 PM
```
其中,时间的格式化字符串可以根据需要进行调整。
希望能对你有所帮助。
#第二次作业 #26 #(1) lst=[1,2,3,4,5] square=map(lambda x:x*x,lst) print(list(square)) #(2) even=filter(lambda x:x%2==0,lst) print(list(even)) #27 #(1) file1=open("E:/大一/python与程序设计/file1.txt","r") content1=file1.read() lst1=content1.split() num=list(map(int,lst1)) allnum=sum(num) print(allnum) file1.close() #(2) file1=open("E:/大一/python与程序设计/file1.txt","r") content=[] for i in range(1,4): l=file1.readline() num= list(map(int, l.split())) num.sort() strs=" ".join(list(map(str,num))) strs2=strs+"\n" content.append(strs2) file2=open("E:/大一/python与程序设计/file2.txt","w") file2.writelines(content) file2.close() file1.close() #(3) file1=open("E:/大一/python与程序设计/file1.txt","r") content=file1.readlines() print(len(content)) #28 from datetime import datetime as dt file3=open("E:/大一/python与程序设计/file3.txt",'r',encoding='utf-8') line1=file3.readline() content=[] for i in range(1,4): l=file3.readline().split() content.append(l) col1=[content[0][0],content[1][0],content[2][0]] col2=[content[0][1],content[1][1],content[2][1]] col3=[content[0][2],content[1][2],content[2][2]] col4=[content[0][3],content[1][3],content[2][3]] day_formate="%H:%M:%S" Time=[] Code=[] Price=[] Volume=[] for t in col1: Time.append(dt.strptime(t,day_formate)) for c in col2: Code.append(str(c)) for p in col3: Price.append(float(p)) for v in col4: Volume.append(int(v)) file3.close() #29 #(1) mean=lambda x,y,z:(x+y+z)/3 #(2) def mean(*num): if bool(num)==0: return None else: return sum(num)/len(num) #30 def fibo(n): if n==1 or n==2: return 1 else: return fibo(n-1)+fibo(n-2) #31 from math import sqrt class Point(): def __init__(self,x,y): self.x=x self.y=y class Line(Point): def __init__(self,p1,p2): self.p1=p1 self.p2=p2 def lenth(self): lenth=sqrt((self.p1.x-self.p2.x)**2+(self.p1.y-self.p2.y)**2) return lenth def slope(self): if self.p1.x==self.p2.x: return None else: k=(self.p1.y-self.p2.y)/(self.p1.x-self.p2.x) return k def __repr__(self): return ((self.p1),(self.p2)) p1=Point(2,3) p2=Point(5,9) line=Line(p1,p2) l_line=line.lenth() k_line=line.slope() print(f"起点(2,3)到止点(5,9)的线段长度为{l_line},斜率为{k_line}") #32 class Point(): #(1) def __init__(self,x=0,y=0): self.x=x self.y=y #(2) def trans(self): return (self.y,self.x) #(3) def show(self): return print(f"该点坐标为({self.x},{self.y})") #(4) p1=Point(1,2) p1.trans() p1.show() p2=Point(3,5) p2.trans() p2.show()
对于你的代码,我进行了一些修改和注释,以便更好地理解。请查看以下代码:
# 26
# (1) 计算列表中每个元素的平方
lst = [1, 2, 3, 4, 5]
square = map(lambda x: x * x, lst)
print(list(square))
# (2) 过滤出列表中的偶数
even = filter(lambda x: x % 2 == 0, lst)
print(list(even))
# 27
# (1) 读取文件中的数字,并计算它们的总和
file1 = open("E:/大一/python与程序设计/file1.txt", "r")
content1 = file1.read()
lst1 = content1.split()
num = list(map(int, lst1))
allnum = sum(num)
print(allnum)
file1.close()
# (2) 读取文件中的数字,并将每行数字进行排序后写入新文件
file1 = open("E:/大一/python与程序设计/file1.txt", "r")
content = []
for i in range(1, 4):
l = file1.readline()
num = list(map(int, l.split()))
num.sort()
strs = " ".join(list(map(str, num)))
strs2 = strs + "\n"
content.append(strs2)
file2 = open("E:/大一/python与程序设计/file2.txt", "w")
file2.writelines(content)
file2.close()
file1.close()
# (3) 读取文件中的行数
file1 = open("E:/大一/python与程序设计/file1.txt", "r")
content = file1.readlines()
print(len(content))
# 28
# 从文件中读取数据,并按照列进行解析
from datetime import datetime as dt
file3 = open("E:/大一/python与程序设计/file3.txt", 'r', encoding='utf-8')
line1 = file3.readline()
content = []
for i in range(1, 4):
l = file3.readline().split()
content.append(l)
# 将字符串时间转换为datetime类型
col1 = [content[0][0], content[1][0], content[2][0]]
day_formate = "%H:%M:%S"
Time = [dt.strptime(t, day_formate) for t in col1]
# 将字符串转换为其他类型
col2 = [content[0][1], content[1][1], content[2][1]]
Code = [str(c) for c in col2]
col3 = [content[0][2], content[1][2], content[2][2]]
Price = [float(p) for p in col3]
col4 = [content[0][3], content[1][3], content[2][3]]
Volume = [int(v) for v in col4]
file3.close()
# 29
# (1) 求三个数的平均值
mean = lambda x, y, z: (x + y + z) / 3
# (2) 求任意数量的数的平均值
def mean(*num):
if bool(num) == 0:
return None
else:
return sum(num) / len(num)
# 30
# 计算斐波那契数列的第n项
def fibo(n):
if n == 1 or n == 2:
return 1
else:
return fibo(n - 1) + fibo(n - 2)
# 31
# 定义Point和Line类,并计算线段长度和斜率
from math import sqrt
class Point():
def __init__(self, x, y):
self.x = x
self.y = y
class Line(Point):
def __init__(self, p1, p2):
self.p1 = p1
self.p2 = p2
def length(self):
length = sqrt((self.p1.x - self.p2.x) ** 2 + (self.p1.y - self.p2.y) ** 2)
return length
def slope(self):
if self.p1.x == self.p2.x:
return None
else:
k = (self.p1.y - self.p2.y) / (self.p1.x - self.p2.x)
return k
def __repr__(self):
return ((self.p1), (self.p2))
p1 = Point(2, 3)
p2 = Point(5, 9)
line = Line(p1, p2)
l_length = line.length()
k_line = line.slope()
print(f"起点(2,3)到止点(5,9)的线段长度为{l_length},斜率为{k_line}")
# 32
# (1) 定义一个Point类,包含x和y坐标
class Point():
def __init__(self, x=0, y=0):
self.x = x
self.y = y
# (2) 定义一个trans()函数,将坐标轴进行转换
def trans(self):
return (self.y, self.x)
# (3) 定义一个show()函数,打印出点的坐标
def show(self):
return print(f"该点坐标为({self.x},{self.y})")
p1 = Point(1, 2)
p1.trans()
p1.show()
p2 = Point(3, 5)
p2.trans()
p2.show()
相关推荐
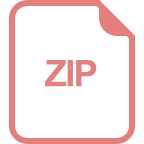
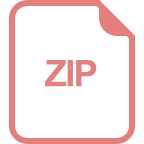












