LSTM预测未来的预测部分代码与代码解释
时间: 2024-10-25 16:02:53 浏览: 17
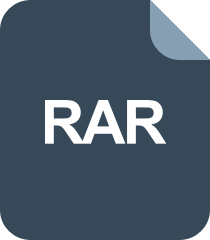
LSTM预测,lstm预测未来多天数据,matlab

LSTM(长短时记忆网络)是一种特殊的循环神经网络,用于处理序列数据,特别是时间序列预测。在预测未来值时,通常会采用以下步骤:
```python
import tensorflow as tf
from tensorflow.keras.layers import LSTM, Dense
# 假设我们有一个时间序列模型,其中包含一个LSTM层
model = tf.keras.Sequential([
LSTM(units=64, input_shape=(input_seq_length, num_features), return_sequences=True), # 输入层、LSTM单元数和输入长度
LSTM(units=32, return_sequences=False), # 下一层LSTM,不返回序列结果
Dense(units=num_features) # 输出层,对应原始特征的数量
])
# 编译模型
model.compile(optimizer='adam', loss='mean_squared_error')
# 训练模型(假设X_train和y_train是训练数据)
history = model.fit(X_train, y_train, epochs=50, validation_data=(X_val, y_val))
# 预测未来值
# 假设你想预测n步未来
future_steps = 10
last_observed_sequence = X_train[-1:] # 最近的一次观测值作为初始输入
predictions = []
for _ in range(future_steps):
output = model(last_observed_sequence)
predictions.append(output)
last_observed_sequence = np.concatenate((last_observed_sequence[1:], [output])) # 使用上一步的预测作为新的输入
# 合并预测结果
predicted_future_sequence = np.array(predictions).flatten()
# 对于实际应用,你可以将predicted_future_sequence和真实的未来值对比来评估模型性能
```
在这个代码中:
1. `LSTM` 层定义了长期记忆单元,它们可以记住先前的信息并传递给下一层。
2. `Dense` 层是全连接层,用于从LSTM输出转换到预测的数值。
3. `fit` 函数用于训练模型,通过最小化损失函数调整权重。
4. `predict` 用于基于最后一个观测值生成未来预测。
5. 预测过程中,每次迭代使用上一次预测值作为新的输入,逐步向前推进。
阅读全文
相关推荐
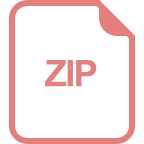
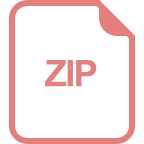















