基于opencv对指定图像进行预处理操作,包括图像增强、平滑、二值化、腐蚀、膨胀等处理,完成车牌识别(代码请详细注释)
时间: 2024-10-20 07:13:15 浏览: 40
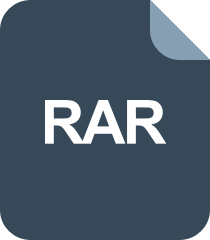
图像黑白化处理_图像二值化_
在OpenCV中进行图像预处理并进行车牌识别通常涉及以下几个步骤。这里我会给出一个Python示例,使用OpenCV库及其相关的函数:
```python
# 导入所需的库
import cv2
import numpy as np
# 加载图像
image = cv2.imread('your_image_path.jpg', 0) # 读取灰度图,0表示只加载灰度通道
# 图像增强(提高对比度)
# 可能使用的函数如 adaptiveThreshold 或者 clahe (自适应直方图均衡化)
clahe = cv2.createCLAHE(clipLimit=2.0, tileGridSize=(8, 8))
enhanced_image = clahe.apply(image)
# 平滑 - 使用高斯滤波器去除噪声
blur_image = cv2.GaussianBlur(enhanced_image, (5, 5), 0)
# 二值化 - 将图像转换为黑白,常用的是Otsu's thresholding
_, binary_image = cv2.threshold(blur_image, 0, 255, cv2.THRESH_BINARY + cv2.THRESH_OTSU)
# 腐蚀和膨胀操作 - 形态学操作用于消除小斑点,保持轮廓连续性
kernel = np.ones((3, 3), np.uint8) # 生成一个3x3的结构元素
eroded_image = cv2.erode(binary_image, kernel, iterations=1)
dilated_image = cv2.dilate(eroded_image, kernel, iterations=1)
# 进一步细化边缘 - Canny算子可以检测边缘
canny_edges = cv2.Canny(dilated_image, low_threshold=50, high_threshold=150)
# 轮廓检测 - 查找可能的车牌区域
contours, _ = cv2.findContours(canny_edges.copy(), cv2.RETR_EXTERNAL, cv2.CHAIN_APPROX_SIMPLE)
# 遍历轮廓,找到最大面积的候选区域作为车牌
max_contour = max(contours, key=cv2.contourArea)
approximation = cv2.approxPolyDP(max_contour, 0.01 * cv2.arcLength(max_contour, True), True)
if len(approximation) == 4: # 检查是否接近四边形
plate_cropped = image[max_contour[:, :, 1].min():max_contour[:, :, 1].max(),
max_contour[:, :, 0].min():max_contour[:, :, 0].max()]
# 对车牌区域进行进一步的文字识别,这一步通常需要OCR工具如Tesseract
# plate_text = pytesseract.image_to_string(plate_cropped)
```
**
阅读全文
相关推荐
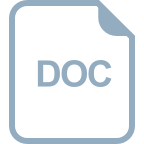
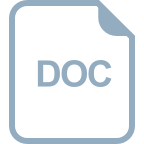
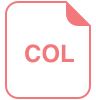
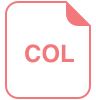
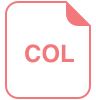
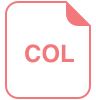
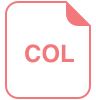
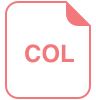
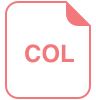
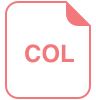







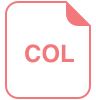