在部分类别数量稀少的情况下,进行14分类的算法,并可视化预测结果以及图Python实现完成代码
时间: 2024-09-24 09:09:00 浏览: 43
在数据类别相对较少的情况下,对于14个类别的分类任务,可以考虑使用简单的监督学习模型,如决策树、KNN(k-近邻)或者朴素贝叶斯等。如果你想要可视化预测结果,常用的库有Matplotlib用于绘制图表,比如混淆矩阵或ROC曲线,而Scikit-learn库中有现成的函数可以帮助生成这些图形。
首先,你需要导入必要的库:
```python
import numpy as np
import pandas as pd
import matplotlib.pyplot as plt
from sklearn.model_selection import train_test_split
from sklearn.neighbors import KNeighborsClassifier
from sklearn.metrics import confusion_matrix, classification_report, plot_confusion_matrix
from sklearn.naive_bayes import GaussianNB
```
假设你已经有了训练集数据`X_train`和`y_train`,测试集数据`X_test`和`y_test`:
```python
# 分割数据
X_train, X_test, y_train, y_test = train_test_split(X, y, test_size=0.2, random_state=42)
# 选择模型,这里以KNN为例
model = KNeighborsClassifier(n_neighbors=5) # 或者用GaussianNB()
model.fit(X_train, y_train)
# 预测
y_pred = model.predict(X_test)
# 可视化混淆矩阵
plot_confusion_matrix(model, X_test, y_test, cmap=plt.cm.Blues)
plt.title('Confusion Matrix')
plt.show()
# 可视化分类报告
print(classification_report(y_test, y_pred))
```
这段代码会先训练一个KNN模型,然后对测试集进行预测,最后显示混淆矩阵和分类报告以评估模型性能。注意,实际应用中你可能需要尝试调整K值或其他超参数来优化模型效果。
阅读全文
相关推荐
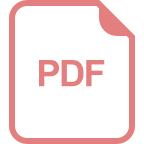
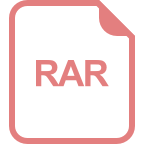
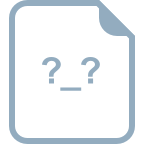
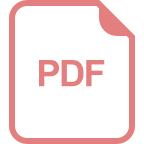
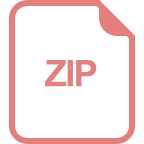
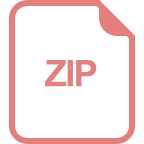
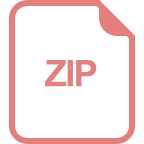
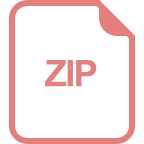
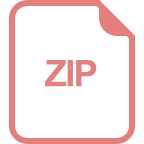
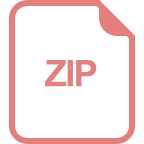
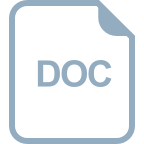
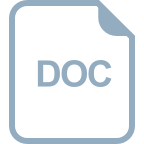
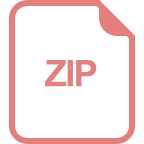
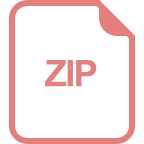
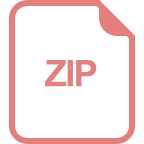