使用tensorflow框架运行facenet模型
时间: 2024-06-15 12:03:00 浏览: 16
Facenet是一个用于人脸识别和人脸验证的深度学习模型,它基于TensorFlow框架实现。下面是使用TensorFlow框架运行Facenet模型的步骤:
1. 安装TensorFlow:首先,你需要安装TensorFlow框架。你可以通过pip命令来安装TensorFlow,例如:`pip install tensorflow`。
2. 下载Facenet模型:你可以从Facenet的GitHub仓库中下载预训练的模型。在GitHub仓库的[releases页面](https://github.com/davidsandberg/facenet/releases)中,你可以找到不同版本的预训练模型。选择一个适合你的需求的模型,并下载它。
3. 加载模型:使用TensorFlow加载Facenet模型。你可以使用TensorFlow提供的`tf.saved_model.load()`函数来加载模型。例如:
```python
import tensorflow as tf
model = tf.saved_model.load('path/to/saved_model')
```
这将加载Facenet模型,并将其存储在`model`变量中。
4. 预处理输入图像:在将图像输入Facenet模型之前,你需要对图像进行预处理。通常,这包括将图像调整为模型所需的大小,并进行归一化处理。你可以使用TensorFlow提供的函数来完成这些操作。例如:
```python
import tensorflow as tf
from PIL import Image
def preprocess_image(image_path):
image = Image.open(image_path)
image = image.resize((160, 160)) # 调整图像大小为160x160
image = tf.keras.preprocessing.image.img_to_array(image)
image = (image - 127.5) / 128.0 # 归一化处理
return image
image = preprocess_image('path/to/image.jpg')
```
这将加载图像,并将其调整为160x160的大小,并进行归一化处理。
5. 运行模型:使用加载的模型对预处理后的图像进行推理。你可以使用TensorFlow提供的`model.predict()`函数来运行模型。例如:
```python
import tensorflow as tf
embedding = model.predict(tf.expand_dims(image, axis=0))
```
这将对图像进行推理,并生成一个表示人脸特征的嵌入向量。
以上是使用TensorFlow框架运行Facenet模型的基本步骤。你可以根据自己的需求进行进一步的调整和优化。
相关推荐
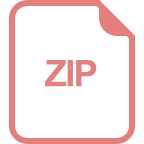
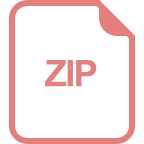
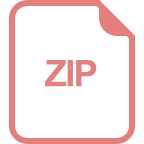














