Write pytorch-based Python code to implement a neural network that solves binary classification problem with an output layer of a positively weighted sub-network plus a negatively weighted sub-network,where the negative weight is random and take the opposite of the absolute value of the standard normal distribution, the exact value of the two weights are obtained from the objective function by Back propagation. The activation function is set to tanh.
时间: 2024-04-08 17:29:28 浏览: 28
Sure! Here's a PyTorch-based Python code that implements a neural network for binary classification with the specified requirements:
```python
import torch
import torch.nn as nn
import torch.optim as optim
from torch.autograd import Variable
# Define the neural network model
class BinaryClassificationNet(nn.Module):
def __init__(self, input_size):
super(BinaryClassificationNet, self).__init__()
self.input_size = input_size
self.hidden_size = 10
self.output_size = 1
self.positive_subnet = nn.Linear(self.input_size, self.hidden_size)
self.negative_subnet = nn.Linear(self.input_size, self.hidden_size)
self.output_layer = nn.Linear(self.hidden_size, self.output_size)
# Initialize negative weight as random value from the standard normal distribution
nn.init.normal_(self.negative_subnet.weight, 0, 1)
self.negative_subnet.weight.data *= -1
def forward(self, x):
hidden_pos = torch.tanh(self.positive_subnet(x))
hidden_neg = torch.tanh(self.negative_subnet(x))
hidden_combined = hidden_pos + hidden_neg
output = torch.tanh(self.output_layer(hidden_combined))
return output
# Set random seed for reproducibility
torch.manual_seed(42)
# Generate random input data for training
input_size = 10
num_samples = 1000
X_train = torch.randn(num_samples, input_size)
y_train = torch.randint(0, 2, (num_samples,))
# Create an instance of the BinaryClassificationNet model
model = BinaryClassificationNet(input_size)
# Define loss function and optimizer
criterion = nn.BCELoss()
optimizer = optim.SGD(model.parameters(), lr=0.01)
# Training loop
num_epochs = 1000
for epoch in range(num_epochs):
# Forward pass
output = model(X_train)
loss = criterion(output.squeeze(), y_train.float())
# Backward pass and optimization
optimizer.zero_grad()
loss.backward()
optimizer.step()
# Print loss every 100 epochs
if (epoch+1) % 100 == 0:
print(f'Epoch {epoch+1}/{num_epochs}, Loss: {loss.item()}')
# Test the trained model
X_test = torch.randn(10, input_size)
y_pred = model(X_test)
print('\nPredictions for test data:')
print(y_pred.squeeze().detach().numpy())
```
This code defines a `BinaryClassificationNet` class that represents the neural network model. It has two sub-networks: `positive_subnet` and `negative_subnet`. The negative weight is randomly initialized using values from the standard normal distribution and then multiplied by -1. The `forward` method performs the forward pass through the network.
In the training loop, random input data `X_train` and corresponding labels `y_train` are generated. The model is trained using binary cross-entropy loss and stochastic gradient descent optimizer.
After training, the model is tested on random test data `X_test`, and the predictions are printed.
Note: Make sure you have PyTorch installed (`pip install torch`) before running this code.
相关推荐
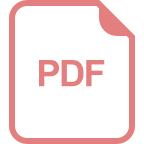
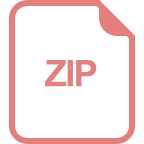













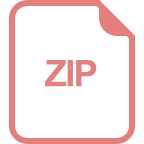
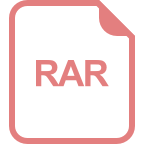