解释CV_Assert(size.width>0 && size.height>0);
时间: 2023-12-10 12:04:24 浏览: 995
这是OpenCV库中的一个宏定义,用于检查图像的宽度是否大于0,如果不满足条件,会报出错误并终止程序运行。CV_Assert的作用类似于assert,但它是OpenCV库中的特定实现。在这里,如果图像的宽度为0或负数,则无法对其进行任何有效操作,因此需要使用CV_Assert来检查图像的宽度是否合法。
相关问题
void dis_homo_to_maps(const cv::Mat &perspective_mat, const cv::Size img_size, cv::Mat distortion_map1, cv::Mat distortion_map2, cv::Mat &map1, cv::Mat &map2) { cv::Mat inv_perspective(perspective_mat.inv()); inv_perspective.convertTo(inv_perspective, CV_32FC1); cv::Mat xy(img_size, CV_32FC2); float *pxy = (float*)xy.data; for (int y = 0; y < img_size.height; y++) for (int x = 0; x < img_size.width; x++) { *pxy++ = x; *pxy++ = y; } cv::Mat xy_transformed; cv::perspectiveTransform(xy, xy_transformed, inv_perspective); float *pmytest = (float*)xy_transformed.data; for (int y = 0; y < xy_transformed.rows; y++) for (int x = 0; x < xy_transformed.cols; x++) { if (abs(*pmytest) > 5000) *pmytest = 5000.00; pmytest++; if (abs(*pmytest) > 5000) *pmytest = 5000.00; pmytest++; } assert(xy_transformed.channels() == 2); cv::Mat maps[2]; // map_x, map_y cv::split(xy_transformed, maps); cv::convertMaps(maps[0], maps[1], map1, map2, CV_32FC1); short int *pt = (short int *)map1.data; short int *dispt = (short int *)distortion_map1.data; for (int i = 0; i < map1.rows; i++) { for (int j = 0; j < map1.cols; j++) { cv::Point tem1; tem1.x = *pt++; tem1.y = *pt++; if ((tem1.x<0) || (tem1.x>distortion_map1.cols - 1) || (tem1.y<0) || (tem1.y>distortion_map1.rows - 1)) continue; int tem2 = (tem1.y*distortion_map1.cols + tem1.x) * 2; dispt += tem2; cv::Point tem3; tem3.x = *dispt++; tem3.y = *dispt++; dispt -= tem2+2; *(--pt) = tem3.y; *(--pt) = tem3.x; pt++; pt++; } } }解释一下这段代码
这段代码是一个函数,实现了将透视变换后的图像映射到畸变校正的图像上。接受的输入包括透视矩阵、图像大小、畸变校正的映射关系等。具体实现过程如下:
1. 将透视矩阵取逆,并将其转换为单精度浮点数类型。
2. 生成一个二维的浮点数类型的矩阵,其中存储了图像上每个像素的坐标。
3. 将图像上的像素坐标通过透视变换,映射到畸变校正后的图像上。
4. 检查映射后的坐标是否超出图像边界,如果超出则将其限制在图像范围内。
5. 将映射后的坐标通过 convertMaps 函数转换为两个映射关系 map1 和 map2。
6. 将 map1 和 distortion_map1 中的像素一一对应,得到映射后的坐标点。
值得注意的是,这段代码中涉及到了指针操作和矩阵的操作,需要对 C++ 的相关知识有一定的了解。
float* get_hog_feature(cv::Mat img) { cv::HOGDescriptor hog = cv::HOGDescriptor(cvSize(20, 20), cvSize(10, 10), cvSize(5, 5), cvSize(5, 5), 9); cv::resize(img, img, cv::Size(30, 30), (0, 0), (0, 0), cv::INTER_LINEAR); std::vector<float> descriptors; // float *descriptors; hog.compute(img, descriptors, cv::Size(20, 20), cv::Size(0, 0)); float *feature_float = (float*)malloc(descriptors.size() * sizeof(float)); assert(feature_float); for (int i = 0; i < 128; i++) { feature_float[i] = descriptors[i * 2]; } return feature_float; } bool getRectsHogFeature(const cv::Mat& img) { std::vector<cv::Mat> mats; int feature_dim = 128; for (DETECTION_ROW& dbox : d) { cv::Rect rc = cv::Rect(int(dbox.tlwh(0)), int(dbox.tlwh(1)), int(dbox.tlwh(2)), int(dbox.tlwh(3))); rc.x = (rc.x >= 0 ? rc.x : 0); rc.y = (rc.y >= 0 ? rc.y : 0); rc.width = (rc.x + rc.width <= img.cols ? rc.width : (img.cols - rc.x)); rc.height = (rc.y + rc.height <= img.rows ? rc.height : (img.rows - rc.y)); cv::Mat mattmp = img(rc).clone(); //cv::resize(mattmp, mattmp, cv::Size(64, 128)); float *feature_float = get_hog_feature(mattmp); for (int i=0;i<feature_dim;i++) { dbox.feature[i] = feature_float[i]; } } return true; } 请解析这段代码
这段代码主要是关于计算图像的HOG特征。下面是对代码的解析:
1. `get_hog_feature` 函数是用来计算输入图像的HOG特征的。它接受一个 `cv::Mat` 类型的图像作为输入参数,返回一个 `float*` 类型的特征数组。
2. 在函数内部,首先创建了一个 `cv::HOGDescriptor` 对象 `hog`,并指定了它的参数,包括窗口大小、块大小、块步长和直方图的箱数。
3. 然后,将输入图像调整为指定的大小(30x30)。
4. 接下来,创建一个空的 `std::vector<float>` 类型的 `descriptors`,用于存储计算得到的特征。
5. 使用 `hog.compute` 函数计算图像的HOG特征,并将结果存储在 `descriptors` 中。
6. 为了方便后续操作,动态分配了一个 `float*` 类型的特征数组 `feature_float`,并将 `descriptors` 中的部分数据复制到该数组中。
7. 最后,返回特征数组 `feature_float`。
另外,还有一个函数 `getRectsHogFeature` 用于将计算得到的HOG特征存储到一个名为 `dbox.feature` 的数组中。该函数接受一个 `cv::Mat` 类型的图像作为输入参数,并遍历一个名为 `d` 的容器,其中存储了一些矩形框的信息。
在每次迭代中,首先根据矩形框的位置和图像的大小,截取对应位置的图像区域。然后,调用 `get_hog_feature` 函数计算该区域的HOG特征,并将特征数据复制到 `dbox.feature` 数组中。
总的来说,这段代码实现了计算图像的HOG特征,并将特征存储到相应的数据结构中,以供后续使用。
阅读全文
相关推荐
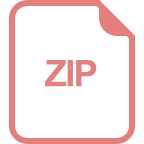
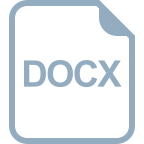
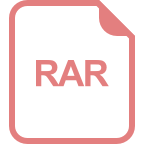


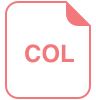
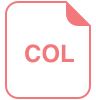
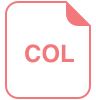






