import tensorflow as tf import numpy as np from keras import Model in_flow= np.load("X_in_30od.npy") out_flow= np.load("X_out_30od.npy") c1 = np.load("X_30od.npy") D1 = np.load("Y_30od.npy") print(c1.shape) print(D1.shape) max=np.max(out_flow) train_in_flow=in_flow[0:200]/max val_in_flow=in_flow[200:260]/max test_in_flow=out_flow[260:]/max train_out_flow=out_flow[0:200]/max val_out_flow=out_flow[200:260]/max test_out_flow=out_flow[260:]/max train_c1=c1[0:200]/max val_c1=c1[200:260]/max test_c1=c1[260:]/max train_D1=D1[0:200]/max val_D1=D1[200:260]/max test_D1=D1[260:]/max print(train_c1.shape, train_in_flow.shape, train_in_flow.shape, train_D1.shape) from keras.layers import * input_od=Input(shape=(5,109,109)) x1=Reshape((5,109,109,1),input_shape=(5,109,109))(input_od) x1=ConvLSTM2D(filters=64,kernel_size=(3,3),activation='relu',padding='same',input_shape=(5,109,109,1))(x1) x1=Dropout(0.2)(x1) x1=Dense(1)(x1) x1=Reshape((109,109))(x1) input_inflow=Input(shape=(5,109)) x2=Permute((2,1))(input_inflow) x2=LSTM(109,return_sequences=True,activation='sigmoid')(x2) x2=Dense(109,activation='sigmoid')(x2) x2=tf.multiply(x1,x2) x2=Dense(109,activation='sigmoid')(x2) input_inflow2=Input(shape=(5,109)) x3=Permute([2,1])(input_inflow2) x3=LSTM(109,return_sequences=True,activation='sigmoid')(x3) x3=Dense(109,activation='sigmoid')(x3) x3 = Reshape((109, 109))(x3) x3=tf.multiply(x1,x3) x3=Dense(109,activation='sigmoid')(x3) mix=Add()([x2,x3]) mix=Bidirectional(LSTM(109,return_sequences=True,activation='sigmoid'))(mix) mix=Dense(109,activation='sigmoid')(mix) model= Model(inputs=[input_od,input_inflow,input_inflow2],outputs=[mix]) model.compile(optimizer='adam', loss='mean_squared_error') history = model.fit([train_c1, train_in_flow,train_in_flow ],train_D1, validation_data=([val_c1,val_out_flow, val_in_flow], val_D1), epochs=50, batch_size=32) model.save("my_model.h5032") 结果显示WARNING:absl:Found untraced functions such as _update_step_xla while saving (showing 1 of 1). These functions will not be directly callable after loading. 怎么保存函数或者保存参数
时间: 2023-12-03 15:44:02 浏览: 153
这个警告是因为使用了 XLA (Accelerated Linear Algebra) 编译器来加速计算,而该编译器的运行方式使得一些函数的跟踪信息无法被保存在模型中。这并不会影响模型的性能或正确性,但可能会影响模型导出后的可用性。
如果你想要保存模型参数,可以使用 `model.save_weights()` 函数来保存训练好的参数。例如:
```
model.save_weights("my_model_weights.h5")
```
如果你想要保存整个模型,包括模型结构和参数,可以使用 `model.save()` 函数。例如:
```
model.save("my_model.h5")
```
注意,这里使用的文件扩展名是 `.h5`,这是因为 Keras 早期版本使用 HDF5 格式来保存模型。如果你使用的是较新的 Keras 版本,也可以使用其他格式,如 `.hdf5` 或 `.keras`。
相关问题
import tensorflow as tf import numpy as np from keras import Model in_flow= np.load("X_in_30od.npy") out_flow= np.load("X_out_30od.npy") c1 = np.load("X_30od.npy") D1 = np.load("Y_30od.npy") print(c1.shape) print(D1.shape) max=np.max(out_flow) train_in_flow=in_flow[0:200]/max val_in_flow=in_flow[200:260]/max test_in_flow=out_flow[260:]/max train_out_flow=out_flow[0:200]/max val_out_flow=out_flow[200:260]/max test_out_flow=out_flow[260:]/max train_c1=c1[0:200]/max val_c1=c1[200:260]/max test_c1=c1[260:]/max train_D1=D1[0:200]/max val_D1=D1[200:260]/max test_D1=D1[260:]/max print(train_c1.shape, train_in_flow.shape, train_in_flow.shape, train_D1.shape) from keras.layers import * input_od=Input(shape=(5,109,109)) x1=Reshape((5,109,109,1),input_shape=(5,109,109))(input_od) x1=ConvLSTM2D(filters=64,kernel_size=(3,3),activation='relu',padding='same',input_shape=(5,109,109,1))(x1) x1=Dropout(0.2)(x1) x1=Dense(1)(x1) x1=Reshape((109,109))(x1) input_inflow=Input(shape=(5,109)) x2=Permute((2,1))(input_inflow) x2=LSTM(109,return_sequences=True,activation='sigmoid')(x2) x2=Dense(109,activation='sigmoid')(x2) x2=tf.multiply(x1,x2) x2=Dense(109,activation='sigmoid')(x2) input_inflow2=Input(shape=(5,109)) x3=Permute([2,1])(input_inflow2) x3=LSTM(109,return_sequences=True,activation='sigmoid')(x3) x3=Dense(109,activation='sigmoid')(x3) x3 = Reshape((109, 109))(x3) x3=tf.multiply(x1,x3) x3=Dense(109,activation='sigmoid')(x3) mix=Add()([x2,x3]) mix=Bidirectional(LSTM(109,return_sequences=True,activation='sigmoid'))(mix) mix=Dense(109,activation='sigmoid')(mix) model= Model(inputs=[input_od,input_inflow,input_inflow2],outputs=[mix]) model.compile(optimizer='adam', loss='mean_squared_error') history = model.fit([train_c1, train_in_flow,train_in_flow ],train_D1, validation_data=([val_c1,val_out_flow, val_in_flow], val_D1), epochs=100, batch_size=32) model.save("my_model.h10032") model.save_weights("my_model_weights.h10032") 根据上述程序利用保持好的模型预测并将预测结果可视化输出
以下是根据您提供的代码进行预测和可视化输出的示例代码:
```
import tensorflow as tf
import numpy as np
from keras import Model
from keras.models import load_model
import matplotlib.pyplot as plt
# 加载模型
model = load_model("my_model.h10032")
# 加载数据
in_flow = np.load("X_in_30od.npy")
out_flow = np.load("X_out_30od.npy")
c1 = np.load("X_30od.npy")
D1 = np.load("Y_30od.npy")
max_val = np.max(out_flow)
# 对输入数据进行预处理
test_in_flow = in_flow[260:] / max_val
test_out_flow = out_flow[260:] / max_val
test_c1 = c1[260:] / max_val
# 进行预测
pred_D1 = model.predict([test_c1, test_out_flow, test_in_flow])
# 可视化输出
plt.figure(figsize=(10, 10))
for i in range(5):
plt.subplot(3, 2, i+1)
plt.imshow(pred_D1[i], cmap='jet')
plt.title("Prediction of OD flow at time step " + str(i+1))
plt.colorbar()
plt.show()
```
注意,这里只输出了前5个时间步的预测结果,您可以根据需要进行修改。另外,这里使用了jet配色方案进行可视化,您可以根据需要进行修改。
下述代码缺少什么import tensorflow as tf import numpy as np from keras import Model in_flow= np.load("X_in_30od.npy") out_flow= np.load("X_out_30od.npy") c1 = np.load("X_30od.npy") D1 = np.load("Y_30od.npy") print(train_in_flow.shape) print(val_in_flow.shape) print(test_in_flow.shape) from keras.layers import * input_od=Input(shape=(5,109,109)) x1=Reshape((5,109,109,1),input_shape=(5,109,109))(input_od) x1=ConvLSTM2D(filters=64,kernel_size=(3,3),activation='relu',padding='same',input_shape=(5,109,109,1))(x1) x1=Dropout(0.2)(x1) x1=Dense(1)(x1) x1=Reshape((109,109))(x1) input_inflow=Input(shape=(5,109)) x2=Permute((2,1))(input_inflow) x2=LSTM(109,return_sequences=True,activation='sigmoid')(x2) x2=Dense(109,activation='sigmoid')(x2) x2=tf.multiply(x1,x2) x2=Dense(109,activation='sigmoid')(x2) input_inflow2=Input(shape=(5,109)) x3=Permute([2,1])(input_inflow2) x3=LSTM(109,return_sequences=True,activation='sigmoid')(x3) x3=Dense(109,activation='sigmoid')(x3) x3 = Reshape((109, 109))(x3) x3=tf.multiply(x1,x3) x3=Dense(109,activation='sigmoid')(x3) mix=Add()([x2,x3]) mix=Bidirectional(LSTM(109,return_sequences=True,activation='sigmoid'))(mix) mix=Dense(109,activation='sigmoid')(mix) model= Model(inputs=[input_od,input_inflow,input_inflow2],outputs=[mix]) model.compile(optimizer='adam', loss='mean_squared_error')
The code is missing the following imports:
```
from keras.layers import Input, ConvLSTM2D, Dropout, Dense, Permute, LSTM, Multiply, Reshape, Add, Bidirectional
```
These are necessary for defining the model architecture.
阅读全文
相关推荐















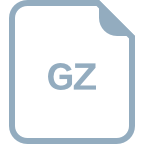