python读mat转成矩阵,使用h5py打开Mat文件并将数据转换为numpy矩阵
时间: 2024-02-25 14:51:36 浏览: 469
可以使用h5py库来打开Mat文件,并将数据转换为numpy矩阵。下面是一个示例代码:
```python
import h5py
import numpy as np
# 打开Mat文件
mat_file = h5py.File('filename.mat', 'r')
# 获取数据
data = mat_file['data_name']
# 将数据转换为numpy矩阵
matrix = np.array(data)
```
在上面的代码中,将Mat文件名和数据名称替换为实际的文件名和数据名称即可。此外,如果Mat文件中有多个数据,您可以使用`keys()`方法来获取所有数据的名称列表。
相关问题
python 读取mat文件 可以存储到什么地方
Python 读取 .mat 文件可以将数据存储到多种数据类型中,包括:
1. numpy.ndarray:可以将.mat 文件中的矩阵或多维数组读取为 numpy 数组,并进行进一步处理和分析。
2. pandas.DataFrame:可以将.mat 文件中的数据读取为 pandas DataFrame,方便进行数据分析和处理。
3. python 原生数据类型:可以将.mat 文件中的数据读取为 Python 中的原生数据类型,如字典、列表、元组等。
读取和存储 .mat 文件的 Python 库有很多,其中比较常用的有 scipy.io、h5py、pytables 等。这些库都提供了方便的 API,可以轻松地读取和存储 .mat 文件中的数据。
例如,使用 scipy.io 库读取 .mat 文件中的数据并保存到 numpy 数组中,可以按照以下代码进行操作:
```python
import scipy.io as sio
# 读取 .mat 文件中的数据
data = sio.loadmat('data.mat')
# 将数据存储到 numpy 数组中
array_data = data['array']
```
当然,您也可以根据需要选择其他的库和数据类型来进行读取和存储。
Traceback (most recent call last): File "D:\python\code\阈值处理20221227\robustness.py", line 7, in <module> data = scipy.io.loadmat('D:/Network-Data-master/01_Jazz.mat') File "D:\python\code\venv\lib\site-packages\scipy\io\matlab\_mio.py", line 226, in loadmat MR, _ = mat_reader_factory(f, **kwargs) File "D:\python\code\venv\lib\site-packages\scipy\io\matlab\_mio.py", line 80, in mat_reader_factory raise NotImplementedError('Please use HDF reader for matlab v7.3 ' NotImplementedError: Please use HDF reader for matlab v7.3 files, e.g. h5py
这个错误说明你正在尝试加载一个 Matlab v7.3 文件,而 Scipy 的 loadmat 函数不支持直接读取这种格式的文件。你可以使用 h5py 库来读取这种文件格式。以下是可能的实现方法:
```python
import h5py
import networkx as nx
import numpy as np
# 加载真实网络的jazz.mat文件
with h5py.File('D:/Network-Data-master/01_Jazz.mat', 'r') as f:
adjacency_matrix = np.array(f['Problem']['A'])
G = nx.from_numpy_matrix(adjacency_matrix)
# 根据节点度数、介数、局部聚类系数、中介中心度、pangerank大小降序排序,burt约束系数大小升序排序,得到每种方法节点顺序表
degree = dict(G.degree())
degree_seq = [node for node, deg in sorted(degree.items(), key=lambda x: x[1], reverse=True)]
betweenness = nx.betweenness_centrality(G)
betweenness_seq = [node for node, btwn in sorted(betweenness.items(), key=lambda x: x[1], reverse=True)]
clustering = nx.clustering(G)
clustering_seq = [node for node, clust in sorted(clustering.items(), key=lambda x: x[1], reverse=True)]
centrality = nx.eigenvector_centrality(G)
centrality_seq = [node for node, cent in sorted(centrality.items(), key=lambda x: x[1], reverse=True)]
pagerank = nx.pagerank(G)
pagerank_seq = [node for node, pr in sorted(pagerank.items(), key=lambda x: x[1], reverse=True)]
burt = nx.constraint(G)
burt_seq = [node for node, con in sorted(burt.items(), key=lambda x: x[1])]
# 每次累计删除10%节点,计算网络的连通性、网络效率、平均最短路径长度,并加入判断,避免出现网络不连通而报错,记录结果
num_nodes = len(G.nodes())
num_steps = 10
results = []
for i in range(num_steps):
percent_deleted = (i+1) * 0.1
nodes_to_delete = degree_seq[:int(percent_deleted*num_nodes)]
G_copy = G.copy()
G_copy.remove_nodes_from(nodes_to_delete)
if nx.is_connected(G_copy):
results.append((percent_deleted, nx.average_shortest_path_length(G_copy), nx.global_efficiency(G_copy)))
else:
results.append((percent_deleted, np.nan, np.nan))
# 将节点删除过程和结果绘制成图表,观察网络鲁棒性下降的情况
fig, ax = plt.subplots(1, 3)
fig.set_size_inches(18, 6)
ax[0].set_title('Degree')
ax[0].plot([degree[n] for n in degree_seq])
ax[0].set_xlabel('Node rank')
ax[0].set_ylabel('Degree')
ax[1].set_title('Efficiency and path length')
ax[1].plot([r[0] for r in results], [r[1] for r in results], label='Average shortest path length')
ax[1].plot([r[0] for r in results], [r[2] for r in results], label='Global efficiency')
ax[1].set_xlabel('Percent nodes deleted')
ax[1].set_ylabel('Metric value')
ax[1].legend()
ax[2].set_title('Burt constraint')
ax[2].plot([burt[n] for n in burt_seq])
ax[2].set_xlabel('Node rank')
ax[2].set_ylabel('Burt constraint')
plt.show()
```
这个代码和之前的代码非常相似,主要区别在于加载和处理 Matlab v7.3 文件的部分。现在使用 h5py 库来读取文件,然后将邻接矩阵转换为 NetworkX 图。
阅读全文
相关推荐
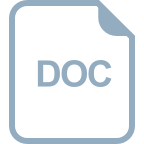
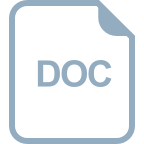
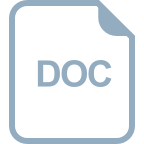
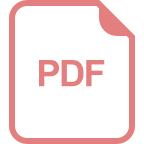
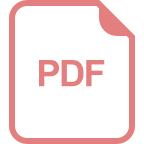
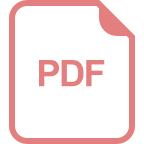
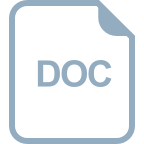
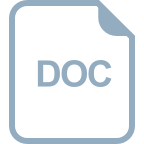
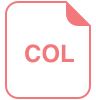
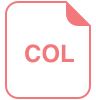
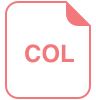
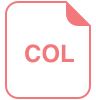
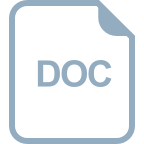
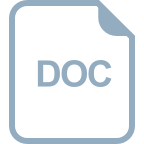
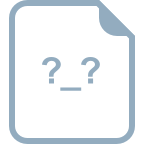
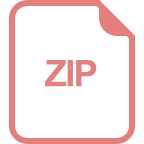
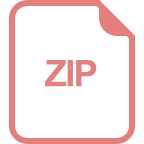