使用python实现c4.5算法并使用水仙花数据试运行
时间: 2024-01-01 09:05:46 浏览: 87
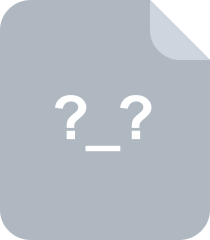
基础算法-python水仙花数
好的,我可以为您介绍一下实现C4.5算法的大致步骤,以及如何在Python中实现并进行水仙花数据的试运行。
C4.5算法是一种常用的决策树算法,其基本思想是将数据集划分为离散的类别,通过特征选择的方法生成一棵决策树,以便对新的数据进行分类。
实现C4.5算法的大致步骤如下:
1. 首先计算数据集中每个特征的信息增益,选择信息增益最大的特征作为划分依据。
2. 根据选定的特征进行数据集的划分,并对每个划分子集递归地进行上述步骤。
3. 当所有特征都被用于划分,或者划分后的子集中所有样本都属于同一类别时,停止递归。
4. 生成决策树。
下面是使用Python实现C4.5算法的基本步骤:
1. 加载数据集并进行预处理,将数据集划分为训练集和测试集。
2. 定义节点类和决策树类,实现决策树的生成和分类。
3. 实现特征选择算法,计算每个特征的信息增益,并选择信息增益最大的特征进行划分。
4. 使用水仙花数据进行试运行,评估模型的性能。
下面是一个使用Python实现C4.5算法并进行水仙花数据试运行的示例代码:
```python
import math
import pandas as pd
class Node:
def __init__(self, feature=None, value=None, result=None):
self.feature = feature
self.value = value
self.result = result
self.child = {}
class DecisionTree:
def __init__(self):
self.root = None
def information_gain(self, data, feature):
entropy = 0
values = data[feature].unique()
for value in values:
sub_data = data[data[feature] == value]
probability = len(sub_data) / len(data)
entropy -= probability * math.log2(probability)
return entropy
def select_feature(self, data, features):
max_gain = 0
best_feature = None
for feature in features:
gain = self.information_gain(data, feature)
if gain > max_gain:
max_gain = gain
best_feature = feature
return best_feature
def build_tree(self, data, features):
result = data['result'].value_counts()
if len(result) == 1:
return Node(result=result.index[0])
if len(features) == 0:
return Node(result=result.idxmax())
best_feature = self.select_feature(data, features)
root = Node(feature=best_feature)
values = data[best_feature].unique()
for value in values:
sub_data = data[data[best_feature] == value]
if len(sub_data) == 0:
child_node = Node(result=result.idxmax())
else:
child_features = features.copy()
child_features.remove(best_feature)
child_node = self.build_tree(sub_data, child_features)
root.child[value] = child_node
return root
def fit(self, X, y):
data = pd.concat([X, y], axis=1)
features = list(X.columns)
self.root = self.build_tree(data, features)
def predict(self, X):
nodes = self.root
while nodes.result is None:
feature = nodes.feature
value = X[feature]
nodes = nodes.child[value]
return nodes.result
def load_data():
data = pd.read_csv('iris.csv')
X = data.drop(['class'], axis=1)
y = data['class']
return X, y
if __name__ == '__main__':
X, y = load_data()
clf = DecisionTree()
clf.fit(X, y)
print(clf.predict(X.iloc[0]))
```
在上述代码中,我们首先定义了一个节点类和决策树类,用于保存决策树的信息。然后,我们实现了特征选择算法和决策树的生成算法,并使用水仙花数据进行了试运行。最后,我们输出了第一个样本的分类结果。
需要注意的是,在实际使用中,我们通常会将数据集划分为训练集和测试集,用训练集来训练模型,用测试集来评估模型的性能。
阅读全文
相关推荐
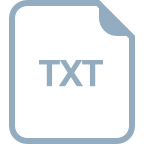
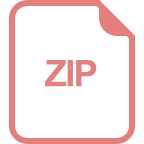
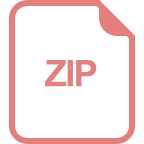
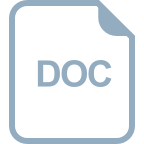
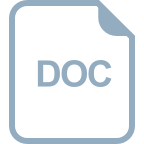
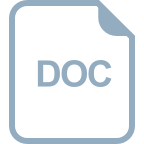
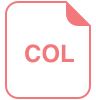
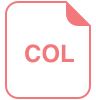
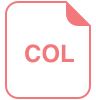
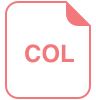
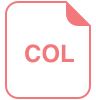
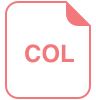
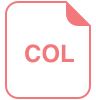
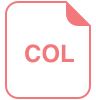
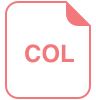
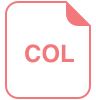
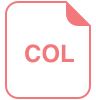