又显示”错误使用 plot 向量长度必须相同。 出错 exe3_7 (第 12 行) pic= plot(axes1, linspace(0, timeLength, samples), y); % 初始化音频波形图“
时间: 2024-04-12 15:29:33 浏览: 62
这个错误是由于 `linspace` 函数生成的时间点和音频数据 `y` 的长度不匹配引起的。`linspace` 函数生成的时间点数量应该与音频数据 `y` 的长度相同。
你可以尝试修改代码,使用 `linspace` 函数生成与音频数据 `y` 长度相同的时间点,如下所示:
```matlab
t = linspace(0, timeLength, samples); % 生成与音频数据 y 长度相同的时间点
pic = plot(axes1, t, y); % 初始化音频波形图
```
通过这样的修改,你将使用正确长度的时间点来初始化音频波形图,避免了长度不匹配的错误。
请注意,`linspace` 函数的第三个参数是生成的等间隔点的数量,应与音频数据 `y` 的长度一致。在修改代码时,请确保 `samples` 变量的值与音频数据 `y` 的长度一致,并根据需要进行调整。
相关问题
rf = RandomForestClassifier(n_estimators=90, max_depth=) train_sizes, train_scores, cv_scores = learning_curve(rf,x_train,y_train,cv=5,train_sizes=np.linspace(0.01,1,100)) train_scores_mean = np.mean(train_scores, axis=1) train_scores_std = np.std(train_scores, axis=1) cv_scores_mean = np.mean(cv_scores, axis=1) cv_scores_std = np.std(cv_scores, axis=1) fig = plt.figure(figsize=(8,6), dpi=200) ax = fig.add_axes([0.1, 0.1, 0.8, 0.8]) ax.plot(train_sizes, train_scores_mean, color='dodgerblue', alpha=0.8) ax.plot(train_sizes, cv_scores_mean, color='g', alpha=0.8) ax.fill_between(train_sizes, train_scores_mean - train_scores_std, train_scores_mean + train_scores_std, alpha=0.1, color="dodgerblue") ax.fill_between(train_sizes, cv_scores_mean - cv_scores_std, cv_scores_mean + cv_scores_std, alpha=0.1, color="g") ax.legend(labels=['train_set_scores', 'cross_val_scores'], loc='best') ax.set_title('Learning curve of the random forests') ax.grid(True) ax.set_xlabel('The number of training samples') ax.set_ylabel('Model score') plt.savefig('Learning curve of the random forests.jpg') plt.show()
这段代码使用了随机森林分类器(RandomForestClassifier)进行训练,并使用学习曲线(learning_curve)来评估模型的性能。具体来说,它通过在训练集上逐渐增加样本数量,并在交叉验证集上计算模型得分,来绘制训练集和交叉验证集得分随训练集样本数量变化的曲线。这可以帮助我们判断模型是否存在过拟合或欠拟合的问题。
其中,n_estimators是指随机森林中树的个数,max_depth是指每棵树的最大深度。learning_curve函数中的train_sizes参数指定了训练集的大小范围,np.linspace(0.01,1,100)表示从0.01到1均匀取100个数,即训练集大小从1%到100%。cv参数指定了交叉验证的折数,这里设置为5。最后,绘制了训练集得分和交叉验证集得分随训练集样本数量变化的曲线,并保存了图片。
def draw(x, y): y = y[0] x_po = x[np.where(y == 1)] x_ne = x[np.where(y == 0)] #绘制散点图 ax = plt.axes(projection = "3d") x_1 = x_po[0,:] y_1 = x_po[1,:] z_1 = x_po[2,:] x_2 = x_ne[0,:] y_2 = x_ne[1,:] z_2 = x_ne[2,:] #p = 0.5的面 a,b,c,d = w x = np.linspace(-3, 3, 3) y = np.linspace(-3, 3, 3) x_3, y_3 = np.meshgrid(x, y) z_3 = -(a * x_3 + b * y_3 +d) / c ax.scatter(x_1, y_1, z_1, c = "r", label = "Positive") ax.scatter(x_2, y_2, z_2, c = "b", label = "Negative") ax.plot_surface(x_3, y_3, z_3, alpha = 0.5) plt.legend() plt.show() draw(X_train, y_train)
这是一个Python函数,名为draw,它有两个参数x和y。函数的作用是将数据x和标签y中的正例和负例分别提取出来,用于可视化或其他操作。具体来说,函数首先将标签y的第一个元素赋值给变量y,然后使用numpy库中的where函数,找出标签y中等于1的位置,将对应位置的数据x提取出来,存储在变量x_po中;同样地,找出标签y中等于0的位置,将对应位置的数据x提取出来,存储在变量x_ne中。最后,函数返回x_po和x_ne两个变量。
阅读全文
相关推荐
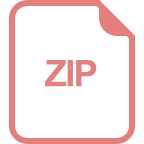
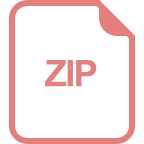













