网格搜索优化CNN-LSTM模型参数python代码
时间: 2023-10-11 12:06:54 浏览: 373
下面是一个使用网格搜索优化CNN-LSTM模型参数的Python代码示例:
```python
from sklearn.model_selection import GridSearchCV
from keras.wrappers.scikit_learn import KerasClassifier
from keras.models import Sequential
from keras.layers import Conv2D, MaxPooling2D, LSTM, Dense, Flatten
# 定义CNN-LSTM模型
def create_model(filters, kernel_size, pool_size, lstm_units, learning_rate):
model = Sequential()
model.add(Conv2D(filters=filters, kernel_size=kernel_size, activation='relu', input_shape=(width, height, channels)))
model.add(MaxPooling2D(pool_size=pool_size))
model.add(Flatten())
model.add(LSTM(units=lstm_units))
model.add(Dense(units=1, activation='sigmoid'))
model.compile(optimizer=Adam(learning_rate=learning_rate), loss='binary_crossentropy', metrics=['accuracy'])
return model
# 创建Keras分类器
model = KerasClassifier(build_fn=create_model)
# 定义参数网格
param_grid = {
'filters': [32, 64],
'kernel_size': [(3, 3), (5, 5)],
'pool_size': [(2, 2), (3, 3)],
'lstm_units': [64, 128],
'learning_rate': [0.001, 0.01]
}
# 创建网格搜索对象
grid_search = GridSearchCV(estimator=model, param_grid=param_grid, cv=3)
# 执行网格搜索
grid_search_result = grid_search.fit(X_train, y_train)
# 输出最佳参数组合和评分
print("Best Parameters: ", grid_search_result.best_params_)
print("Best Score: ", grid_search_result.best_score_)
# 使用最佳参数组合训练模型并进行最终评估
best_model = grid_search_result.best_estimator_
best_model.fit(X_train, y_train)
test_loss, test_accuracy = best_model.evaluate(X_test, y_test)
print("Test Loss: ", test_loss)
print("Test Accuracy: ", test_accuracy)
```
在这个示例中,我们使用`GridSearchCV`来执行网格搜索。我们首先定义了一个函数`create_model`来创建CNN-LSTM模型,并使用`KerasClassifier`将其包装为一个可用于网格搜索的Keras分类器。
然后,我们定义了参数网格`param_grid`,其中包含了我们想要优化的参数范围。
接下来,我们创建了一个`GridSearchCV`对象,并传入模型、参数网格和交叉验证的折数。
最后,我们调用`fit`方法来执行网格搜索。执行完毕后,我们可以通过`best_params_`属性获取最佳参数组合,并通过`best_score_`属性获取最佳模型的评分。
最后,我们使用最佳参数组合训练最佳模型,并在测试集上进行最终评估。
阅读全文
相关推荐
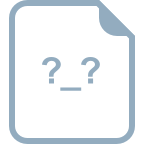
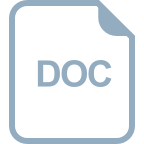
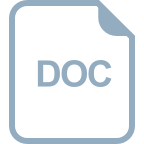
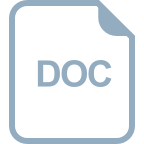
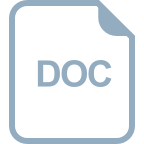
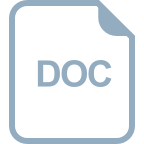
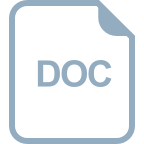
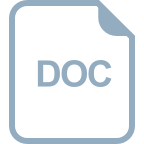

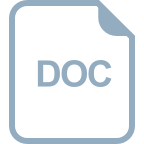
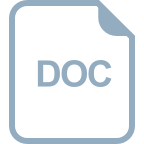
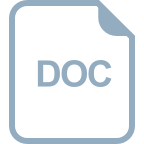
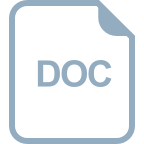
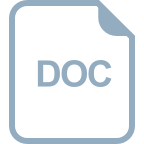
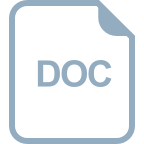
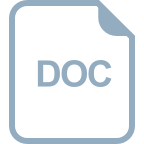
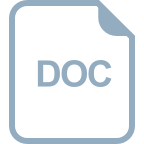