Python实现DeepAR多步预测以及误差分析和可视化代码
时间: 2024-02-22 12:57:11 浏览: 25
DeepAR是一种基于深度学习的时间序列测模型,它可以用于多步预测。下面是Python实现DeepAR多步预测以及误差分析和可视化代码的示例:
首先,我们需要安装gluonts和mxnet库:
```python
!pip install gluonts
!pip install mxnet
```
接着,我们需要导入必要的库:
```python
import numpy as np
import pandas as pd
from gluonts.dataset.common import ListDataset
from gluonts.model.deepar import DeepAREstimator
from gluonts.trainer import Trainer
from gluonts.evaluation.backtest import make_evaluation_predictions
from gluonts.evaluation import Evaluator
import matplotlib.pyplot as plt
```
接下来,我们需要准备数据。这里我们使用一个示例数据集,它包含了200个时间序列,每个时间序列包含了100个数据点。我们将使用前80个数据点作为训练数据,后20个数据点作为测试数据。
```python
# 生成示例数据集
data = np.random.normal(size=(200, 100))
start = pd.Timestamp("01-01-2019", freq="1D")
train_ds = ListDataset(
[
{"start": start, "target": data[i, :80]}
for i in range(data.shape[0])
],
freq="1D",
)
test_ds = ListDataset(
[
{"start": start, "target": data[i, :]}
for i in range(data.shape[0])
],
freq="1D",
)
```
接下来,我们需要定义DeepAR模型的参数。这里我们将使用默认参数。
```python
# 定义DeepAR模型
estimator = DeepAREstimator(
prediction_length=20,
context_length=80,
freq="1D",
num_layers=2,
num_cells=40,
cell_type="lstm",
trainer=Trainer(epochs=10),
)
```
接着,我们需要训练模型,并使用测试数据进行预测。这里我们使用make_evaluation_predictions函数来生成预测结果。
```python
# 训练模型并进行预测
predictor = estimator.train(train_ds)
forecast_it, ts_it = make_evaluation_predictions(
dataset=test_ds, predictor=predictor, num_samples=100
)
forecasts = list(forecast_it)
```
最后,我们可以使用Evaluator来计算模型的误差,并可视化预测结果。
```python
# 计算误差
evaluator = Evaluator(quantiles=[0.1, 0.5, 0.9])
agg_metrics, item_metrics = evaluator(iter(ts_it), iter(forecast_it), num_series=len(test_ds))
# 可视化预测结果
for i in range(3):
ts_entry = next(iter(test_ds))
ts = ts_entry["target"]
forecast_entry = forecasts[i]
forecast = forecast_entry.mean
plt.plot(np.arange(len(ts)), ts, label="target")
plt.plot(np.arange(len(ts), len(ts) + len(forecast)), forecast, label="forecast")
plt.axvline(len(ts), linestyle="dashed", color="black")
plt.legend()
plt.show()
```
以上就是Python实现DeepAR多步预测以及误差分析和可视化代码的示例。
相关推荐
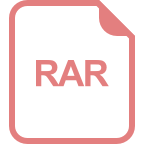
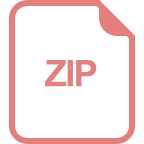














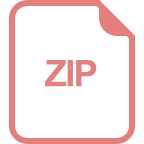