Robbins-Monro setting
时间: 2024-06-03 10:07:31 浏览: 87
The Robbins-Monro setting is a theoretical framework for stochastic optimization problems. It is named after Herbert Robbins and Sutton Monro, who first introduced it in a paper published in 1951. The setting is used to find the solution to an optimization problem where the objective function is unknown or difficult to compute.
In the Robbins-Monro setting, the objective function is modeled as a random variable that depends on a set of parameters. The goal is to find the optimal values of these parameters that minimize the objective function. The setting assumes that the objective function can be estimated using noisy or incomplete information, and that the parameters can be updated iteratively using a set of stochastic rules.
The Robbins-Monro setting is commonly used in machine learning and statistics to solve problems such as stochastic gradient descent, maximum likelihood estimation, and Bayesian inference. It has also been applied in fields such as finance, engineering, and operations research.
阅读全文
相关推荐




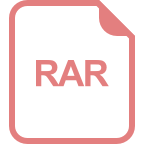












