如何用python实现基于概率分布的超声图像与自然图像性质差异分析这一课题,需要分析大量图像以确保结果的真实性,需要对比两种图像的特征必须有颜色,纹理,形状,差异性分析方法也需要多种,包括完整详细复杂代码
时间: 2023-08-07 07:03:56 浏览: 45
这个课题需要用到图像处理和机器学习相关的知识,下面是一个基本的思路:
1. 数据收集:收集大量的超声图像和自然图像,可以使用公开的数据集或者自己采集。
2. 数据预处理:对于每个图像,需要先进行预处理,包括图像缩放、裁剪、灰度化、归一化等。
3. 特征提取:使用常用的特征提取方法,如局部二值模式(LBP)、灰度共生矩阵(GLCM)、方向梯度直方图(HOG)等,提取颜色、纹理、形状等特征。
4. 模型训练:将提取出的特征作为训练集,使用机器学习算法进行训练,如支持向量机(SVM)、决策树(Decision Tree)、深度学习等。
5. 模型测试:用测试集对模型进行测试,计算准确率、召回率、F1值等指标,评估模型的性能。
6. 差异性分析:通过比较两种图像的特征,计算它们之间的相似性或差异性,可以使用欧氏距离、余弦相似度等方法进行分析。可以将差异性分析结果可视化,辅助研究者理解数据。
以下是一个简单的代码示例,供参考:
```python
import cv2
import numpy as np
from skimage.feature import local_binary_pattern, greycomatrix, greycoprops
from sklearn import svm
# 数据预处理
def preprocess(image):
image = cv2.resize(image, (256, 256)) # 缩放为256x256大小
image = cv2.cvtColor(image, cv2.COLOR_BGR2GRAY) # 灰度化
image = cv2.normalize(image, None, 0, 255, cv2.NORM_MINMAX) # 归一化
return image
# 特征提取 - 颜色特征
def color_feature(image):
mean = cv2.mean(image) # 计算均值
return mean
# 特征提取 - 纹理特征
def texture_feature(image):
lbp = local_binary_pattern(image, 8, 1) # 计算LBP特征
glcm = greycomatrix(lbp, [1], [0, np.pi/4, np.pi/2, 3*np.pi/4]) # 计算GLCM矩阵
contrast = greycoprops(glcm, 'contrast').ravel() # 提取对比度特征
homogeneity = greycoprops(glcm, 'homogeneity').ravel() # 提取一致性特征
return np.hstack([contrast, homogeneity])
# 特征提取 - 形状特征
def shape_feature(image):
contours, hierarchy = cv2.findContours(image, cv2.RETR_EXTERNAL, cv2.CHAIN_APPROX_SIMPLE) # 提取轮廓
area = 0
perimeter = 0
for cnt in contours:
area += cv2.contourArea(cnt) # 计算面积
perimeter += cv2.arcLength(cnt, True) # 计算周长
return np.array([area, perimeter])
# 训练模型
def train_model(X, y):
clf = svm.SVC(kernel='linear', C=1.0) # 创建SVM分类器
clf.fit(X, y) # 训练模型
return clf
# 测试模型
def test_model(clf, X, y):
y_pred = clf.predict(X) # 预测标签
acc = np.sum(y_pred == y) / len(y) # 计算准确率
return acc
# 差异性分析
def diff_analysis(X1, X2):
dist = np.linalg.norm(X1 - X2) # 计算欧氏距离
sim = np.dot(X1, X2) / (np.linalg.norm(X1) * np.linalg.norm(X2)) # 计算余弦相似度
return dist, sim
# 主函数
if __name__ == '__main__':
# 数据收集
ultrasound_images = [...] # 超声图像数据集
natural_images = [...] # 自然图像数据集
labels = [...] # 数据标签
# 特征提取
X = []
for image in ultrasound_images:
image = preprocess(image)
color = color_feature(image)
texture = texture_feature(image)
shape = shape_feature(image)
feature = np.hstack([color, texture, shape])
X.append(feature)
for image in natural_images:
image = preprocess(image)
color = color_feature(image)
texture = texture_feature(image)
shape = shape_feature(image)
feature = np.hstack([color, texture, shape])
X.append(feature)
X = np.array(X)
y = np.array(labels)
# 模型训练
clf = train_model(X, y)
# 模型测试
acc = test_model(clf, X, y)
print('Accuracy:', acc)
# 差异性分析
image1 = ultrasound_images[0]
image2 = natural_images[0]
feature1 = preprocess(image1)
feature2 = preprocess(image2)
diff_dist, diff_sim = diff_analysis(feature1, feature2)
print('Difference Distance:', diff_dist)
print('Difference Similarity:', diff_sim)
```
这只是一个简单的示例,具体实现还需要根据具体情况进行调整和优化。
相关推荐
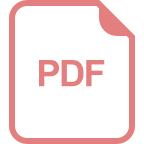
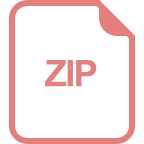
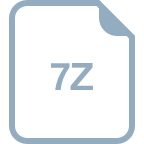







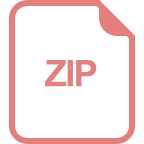
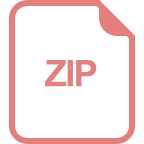
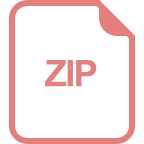
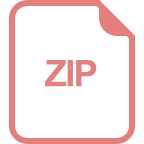
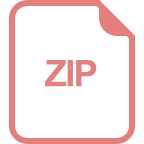
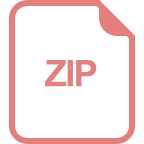
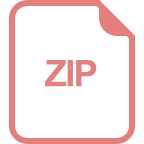
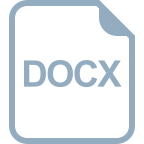
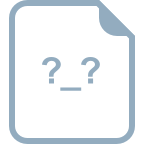