tensorflow遥感图像分类
时间: 2023-09-18 18:11:10 浏览: 119
要使用TensorFlow进行遥感图像分类,一般需要以下步骤:
1. 数据准备:收集并准备用于训练和测试的遥感图像数据集。数据集应该包含图像和其对应的标签,标签指示图像所属的类别。
2. 数据预处理:对数据进行预处理,包括图像增强、图像缩放和图像标准化等操作。
3. 构建模型:使用TensorFlow建立一个卷积神经网络(CNN),并根据数据集进行训练,以便模型能够识别遥感图像。
4. 模型评估:使用测试集评估模型的性能,了解模型的准确性和召回率。
5. 模型优化:根据评估结果,对模型进行优化,以提高其准确性和召回率。
6. 模型应用:将模型应用到实际的遥感图像分类任务中,进行分类预测。
以上是一般的流程,具体实现细节需要根据具体情况进行调整。
相关问题
tensorflow遥感图像分类代码
以下是使用 TensorFlow 进行遥感图像分类的示例代码:
```python
import tensorflow as tf
import numpy as np
import os
# 定义超参数
learning_rate = 0.001
training_epochs = 50
batch_size = 128
display_step = 1
# 定义网络参数
n_input = 784 # MNIST 数据输入(图像尺寸: 28*28)
n_classes = 6 # 总共有 6 类遥感图像
# 加载数据集
def load_data():
x_train = np.load('x_train.npy') # 训练集数据
y_train = np.load('y_train.npy') # 训练集标签
x_test = np.load('x_test.npy') # 测试集数据
y_test = np.load('y_test.npy') # 测试集标签
return x_train, y_train, x_test, y_test
# 创建模型
def neural_net(x):
# 定义权重和偏置
weights = {
'h1': tf.Variable(tf.random_normal([n_input, 256])),
'h2': tf.Variable(tf.random_normal([256, 256])),
'out': tf.Variable(tf.random_normal([256, n_classes]))
}
biases = {
'b1': tf.Variable(tf.random_normal([256])),
'b2': tf.Variable(tf.random_normal([256])),
'out': tf.Variable(tf.random_normal([n_classes]))
}
# 创建网络模型
layer_1 = tf.add(tf.matmul(x, weights['h1']), biases['b1'])
layer_1 = tf.nn.relu(layer_1)
layer_2 = tf.add(tf.matmul(layer_1, weights['h2']), biases['b2'])
layer_2 = tf.nn.relu(layer_2)
out_layer = tf.matmul(layer_2, weights['out']) + biases['out']
return out_layer
# 创建损失函数和优化器
def train_neural_net(x_train, y_train, x_test, y_test):
x = tf.placeholder(tf.float32, [None, n_input])
y = tf.placeholder(tf.float32, [None, n_classes])
# 创建模型
logits = neural_net(x)
# 定义损失函数和优化器
loss_op = tf.reduce_mean(tf.nn.softmax_cross_entropy_with_logits(logits=logits, labels=y))
optimizer = tf.train.AdamOptimizer(learning_rate=learning_rate)
train_op = optimizer.minimize(loss_op)
# 定义评估模型的准确率的操作
correct_pred = tf.equal(tf.argmax(logits, 1), tf.argmax(y, 1))
accuracy = tf.reduce_mean(tf.cast(correct_pred, tf.float32))
# 初始化变量
init = tf.global_variables_initializer()
with tf.Session() as sess:
sess.run(init)
for epoch in range(training_epochs):
total_batch = int(len(x_train) / batch_size)
for i in range(total_batch):
batch_x = x_train[i*batch_size:(i+1)*batch_size]
batch_y = y_train[i*batch_size:(i+1)*batch_size]
# 训练模型
sess.run(train_op, feed_dict={x: batch_x, y: batch_y})
# 每一轮结束后计算训练集和测试集上的准确率
train_acc = sess.run(accuracy, feed_dict={x: x_train, y: y_train})
test_acc = sess.run(accuracy, feed_dict={x: x_test, y: y_test})
# 输出训练过程
if epoch % display_step == 0:
print("Epoch:", epoch+1, "Train acc:", train_acc, "Test acc:", test_acc)
print("Optimization Finished!")
# 计算最终模型在测试集上的准确率
test_acc = sess.run(accuracy, feed_dict={x: x_test, y: y_test})
print("Test Accuracy:", test_acc)
if __name__ == '__main__':
x_train, y_train, x_test, y_test = load_data()
train_neural_net(x_train, y_train, x_test, y_test)
```
在这个示例代码中,我们使用了一个基于多层感知器的神经网络来进行遥感图像分类。数据集是一个由 Numpy 数组保存的遥感图像数据集,其中训练集和测试集的数据和标签分别保存在 `x_train.npy`、`y_train.npy`、`x_test.npy` 和 `y_test.npy` 文件中。
代码首先定义了模型的超参数和网络参数,然后定义了一个函数 `load_data()` 来加载数据集。接着,代码定义了神经网络模型的结构,包括输入层、两个隐藏层和输出层。然后,我们定义了损失函数和优化器,并使用训练集来训练模型,并在每一轮结束后计算训练集和测试集上的准确率。最后,我们计算最终模型在测试集上的准确率。
遥感图像分类 python
关于遥感图像分类,Python提供了很多工具和库,比如Scikit-learn、TensorFlow等。其中,Scikit-learn是一个非常流行的机器学习库,可以用于分类、聚类、回归等任务。而TensorFlow则是一个深度学习框架,可以用于构建神经网络模型进行图像分类。
具体来说,遥感图像分类可以分为以下几个步骤:
1. 数据预处理:包括数据读取、数据增强、数据归一化等。
2. 特征提取:从图像中提取出有用的特征,比如纹理、形状、颜色等。
3. 特征选择:选择最具有代表性的特征。
4. 模型训练:使用机器学习或深度学习算法训练模型。
5. 模型评估:评估模型的性能,比如准确率、召回率等。
以下是一个简单的遥感图像分类的Python代码示例:
```python
import numpy as np
from sklearn.model_selection import train_test_split
from sklearn.preprocessing import StandardScaler
from sklearn.svm import SVC
# 读取数据
data = np.loadtxt('data.txt', delimiter=',')
X = data[:, :-1]
y = data[:, -1]
# 数据归一化
scaler = StandardScaler()
X = scaler.fit_transform(X)
# 划分训练集和测试集
X_train, X_test, y_train, y_test = train_test_split(X, y, test_size=0.3, random_state=42)
# 训练模型
clf = SVC(kernel='linear')
clf.fit(X_train, y_train)
# 评估模型
score = clf.score(X_test, y_test)
print('Accuracy:', score)
```
阅读全文
相关推荐
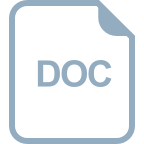
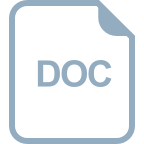
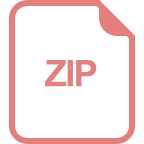
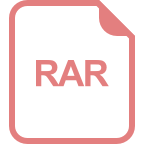
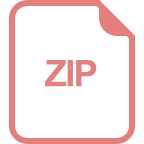
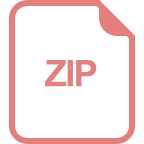
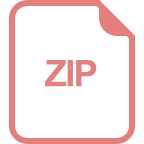
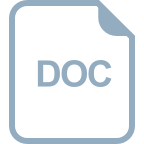
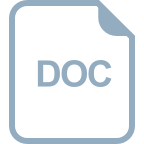
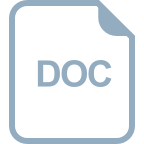
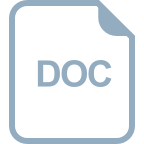

