NBC算法的python伪代码
时间: 2023-10-26 15:42:56 浏览: 37
以下是NBC算法的python伪代码:
#导入必要的库
import pandas as pd
import numpy as np
from sklearn.naive_bayes import GaussianNB
from sklearn.model_selection import train_test_split
#读取数据集
data=pd.read_csv('dataset.csv')
#分离特征和目标
X=data.iloc[:,:-1]
y=data.iloc[:,-1]
#将数据集分为训练集和测试集
X_train, X_test, y_train, y_test = train_test_split(X, y, test_size=0.3, random_state=42)
#定义NBC模型
model=GaussianNB()
#训练模型
model.fit(X_train,y_train)
#预测测试集结果
y_pred=model.predict(X_test)
#计算模型的准确率
accuracy=np.sum(y_pred==y_test)/len(y_test)
print('Accuracy:',accuracy)
相关问题
matlab 多重信号分类算法
MATLAB中有许多多重信号分类算法可用于信号处理和模式识别领域。
一种常用的多重信号分类算法是支持向量机(SVM)。SVM是一种机器学习算法,可以用于将多重信号分类为两个或多个类别。它以非线性分类器的形式运行,通过在输入空间中构建一个超平面来最大化类别间的间距。
另一种常用的算法是人工神经网络(ANN),也称为深度学习。ANN模拟人脑中的神经网络,可以通过训练来识别和分类多重信号。ANN可以处理非线性关系,并且在训练数据中有较强的记忆能力。
还有一种常用的算法是朴素贝叶斯分类器(NBC)。NBC基于贝叶斯定理,通过计算输入信号的属性和类别之间的概率来进行分类。NBC假设输入信号的属性之间是独立的,并对数据进行概率密度估计。
此外,还有许多其他的多重信号分类算法可用于不同的场景和问题。例如,决策树、随机森林、梯度提升机等。这些算法在MATLAB中都有相应的实现函数和工具箱,可以根据具体问题和需求进行选择和使用。
总而言之,MATLAB提供了丰富的多重信号分类算法,可以帮助处理和解决各种信号分类问题。研究人员和工程师可以根据问题的特点和要求选择适当的算法,并使用MATLAB进行实现和分析。
请列出R语言Nbclust包所有代码
### 回答1:
Nbclust包的代码包括:
1. nbc_search:用于搜索最佳聚类参数组合的函数;
2. nbc_extract:用于从搜索结果中提取最佳参数组合的函数;
3. nbc_evaluate:用于评估聚类模型的函数;
4. nbc_plot:用于绘制聚类模型的函数;
5. nbc_select:用于从多个聚类模型中选择最佳模型的函数;
6. nbc_predict:用于预测样本属于哪个聚类的函数;
7. nbc_compare:用于比较不同聚类模型的函数;
8. nbc_hclust:用于层次聚类的函数;
9. nbc_kmeans:用于K-means聚类的函数;
10. nbc_gmm:用于Gaussian Mixture Model聚类的函数;
11. nbc_pam:用于Partitioning Around Medoids聚类的函数;
12. nbc_xmeans:用于X-means聚类的函数;
13. nbc_fanny:用于FANNY聚类的函数;
14. nbc_clara:用于CLARA聚类的函数;
15. nbc_diana:用于DIANA聚类的函数;
16. nbc_monocrit:用于Monocrit聚类的函数;
17. nbc_agnes:用于AGNES聚类的函数;
18. nbc_som:用于Self-Organizing Map聚类的函数;
19. nbc_dbscan:用于DBSCAN聚类的函数;
20. nbc_optics:用于OPTICS聚类的函数;
21. nbc_hdbscan:用于HDBSCAN聚类的函数;
22. nbc_cobweb:用于Cobweb聚类的函数;
23. nbc_em:用于Expectation-Maximization聚类的函数;
24. nbc_clustree:用于Clustree聚类的函数。
### 回答2:
R语言中的Nbclust包是一个用于进行聚类分析和判别分析的工具包。它提供了一系列函数和方法来评估聚类的质量,并为用户提供了选择适当聚类数的建议。
以下是使用Nbclust包的完整示例代码:
```R
# 1. 安装和加载Nbclust包
install.packages("Nbclust")
library(Nbclust)
# 2. 准备数据
data <- iris[, 1:4] # 使用鸢尾花数据集的前4列作为示例数据
# 3. 运行Nbclust函数
# 以下是Nbclust函数中的参数选项,根据需要进行调整
# data:输入的数据对象
# diss:距离矩阵或相似度矩阵
# distance:用于计算距离矩阵的距离度量方法(默认是欧氏距离)
# min.nc:最小聚类数
# max.nc:最大聚类数
# method:用于生成聚类索引的方法(默认是"ward.D")
# index:用于评估聚类质量的指标集合(默认包括30个指标)
# nfactors:主成分分析的因子数(默认为5)
# alpha:主成分分析的显著性水平(默认为0.05)
result <- NbClust(data, diss = NULL, distance = "euclidean", min.nc = 2,
max.nc = 10, method = "ward.D", index = "all")
# 4. 查看聚类评估结果
print(result$Best.nc) # 最佳聚类数
print(result$All.index) # 所有选择的指标结果
print(result$Best.partition) # 最佳聚类结果
```
以上是使用Nbclust包进行聚类分析的完整代码示例。用户可以根据自己的数据和需求调整数据准备和函数参数。
### 回答3:
Nbclust包是R语言中用于集群分析的一个包。下面是列出了Nbclust包中的主要代码:
1. 安装Nbclust包:
```R
install.packages("Nbclust")
```
2. 载入Nbclust包:
```R
library(Nbclust)
```
3. 使用Nbclust进行集群分析:
```R
data <- read.csv("data.csv") # 读取数据文件,将数据保存在data变量中
# 指定要进行聚类的数据列
data <- data[, c("col1", "col2", "col3")]
# 设置参数
nb <- NbClust(data, distance = "euclidean", min.nc = 2, max.nc = 10,
method = "kmeans", index = "all")
# 查看评价指标值和最佳聚类数
print(nb$All.max)
```
上述代码中,首先安装Nbclust包,然后加载该包。接下来,读取要进行集群分析的数据,并指定要进行聚类的数据列。然后,设置NbClust函数的参数,其中distance设置为"euclidean"表示使用欧氏距离计算相似性,min.nc和max.nc指定了聚类数的范围,method设为"kmeans"表示使用k均值聚类算法,index设为"all"表示计算所有可用的评价指标。最后,通过print语句打印出评价指标值和最佳聚类数。
希望以上回答能够帮到你!
相关推荐
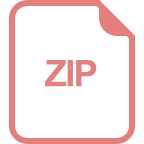
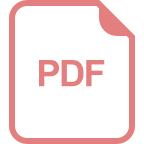
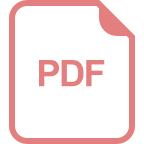









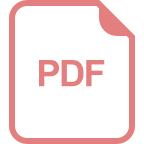