解释代码并讲解上下文关系import kmeans.utils.CentersOperation; import org.apache.hadoop.io.LongWritable; import org.apache.hadoop.io.Text; import org.apache.hadoop.mapreduce.Mapper; import java.io.IOException; import java.util.ArrayList; import java.util.List; public class KMeansMapper extends Mapper<LongWritable, Text, Text, Text> { private List<List<Double>> centers = new ArrayList<>(); @Override protected void map(LongWritable key, Text value, Context context) throws IOException, InterruptedException { String[] dimensions; List<Double> point = new ArrayList<>(); double centerIndex = 1; double minDistance = Double.MAX_VALUE; int iteration = context.getConfiguration().getInt(KMeans.ITERATION, 0); if (centers.size() == 0) { String centersPath = context.getCacheFiles()[0].toString(); centers = CentersOperation.getCenters(centersPath, true); } dimensions = value.toString().split("[,\\t]"); for (int i = 0; i < dimensions.length - 1; i++) { point.add(Double.parseDouble(dimensions[i])); } for (int i = 0; i < centers.size(); i++) { double distance = 0; List<Double> center = centers.get(i); for (int j = 0; j < center.size(); j++) { distance += Math.pow((point.get(j) - center.get(j)), 2); } distance = Math.sqrt(distance); if (distance < minDistance) { minDistance = distance; centerIndex = i + 1; } } String pointData = value.toString().split("\t")[0]; if (iteration == (KMeans.MAX_ITERATION - 1)) { context.write(new Text(pointData), new Text(String.valueOf(centerIndex))); } else { context.write(new Text(String.valueOf(centerIndex)), new Text(pointData)); } } }
时间: 2024-01-03 16:03:32 浏览: 78
这段代码是KMeans算法的Mapper阶段实现,主要作用是将输入的数据集中的每个数据点与已知的聚类中心进行距离计算,找到距离最小的聚类中心,输出键为聚类中心的向量,值为数据点的向量。
具体解释如下:
1. 导入必要的类:
```
import kmeans.utils.CentersOperation;
import org.apache.hadoop.io.LongWritable;
import org.apache.hadoop.io.Text;
import org.apache.hadoop.mapreduce.Mapper;
import java.io.IOException;
import java.util.ArrayList;
import java.util.List;
```
2. 定义KMeansMapper类,并继承Mapper类,并设置泛型类型:
```
public class KMeansMapper extends Mapper<LongWritable, Text, Text, Text> {
```
3. 定义一个列表存储已知的聚类中心:
```
private List<List<Double>> centers = new ArrayList<>();
```
4. 在map方法中实现KMeans算法的Mapper阶段:
```
@Override
protected void map(LongWritable key, Text value, Context context) throws IOException, InterruptedException {
// 读取输入数据
String[] dimensions;
List<Double> point = new ArrayList<>();
double centerIndex = 1;
double minDistance = Double.MAX_VALUE;
int iteration = context.getConfiguration().getInt(KMeans.ITERATION, 0);
// 如果centers列表为空,则读取聚类中心
if (centers.size() == 0) {
String centersPath = context.getCacheFiles()[0].toString();
centers = CentersOperation.getCenters(centersPath, true);
}
// 将数据点转换为List<Double>类型
dimensions = value.toString().split("[,\\t]");
for (int i = 0; i < dimensions.length - 1; i++) {
point.add(Double.parseDouble(dimensions[i]));
}
// 计算数据点与每个聚类中心的距离并找到距离最小的聚类中心
for (int i = 0; i < centers.size(); i++) {
double distance = 0;
List<Double> center = centers.get(i);
for (int j = 0; j < center.size(); j++) {
distance += Math.pow((point.get(j) - center.get(j)), 2);
}
distance = Math.sqrt(distance);
if (distance < minDistance) {
minDistance = distance;
centerIndex = i + 1;
}
}
// 根据迭代次数输出结果
String pointData = value.toString().split("\t")[0];
if (iteration == (KMeans.MAX_ITERATION - 1)) {
context.write(new Text(pointData), new Text(String.valueOf(centerIndex)));
} else {
context.write(new Text(String.valueOf(centerIndex)), new Text(pointData));
}
}
```
5. 解释map方法中的实现:
- 读取输入数据点,并将其转换为List<Double>类型;
- 如果centers列表为空,则读取聚类中心;
- 计算数据点与每个聚类中心的距离并找到距离最小的聚类中心;
- 根据迭代次数输出结果。
6. 其中,`CentersOperation.getCenters(centersPath, true)`方法是从文件中读取聚类中心的方法,`context.getCacheFiles()[0].toString()`是获取DistributedCache中的文件路径的方法。
需要注意的是,这段代码需要和其他KMeans算法的代码一起使用,才能实现完整的KMeans算法的功能。
阅读全文
相关推荐
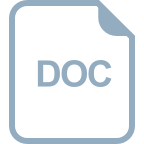
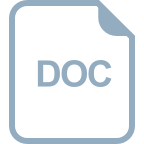
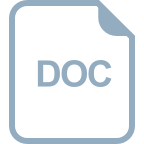
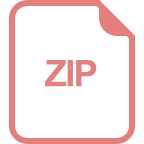
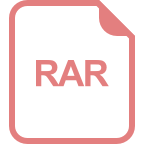
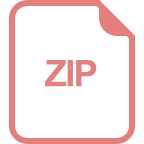
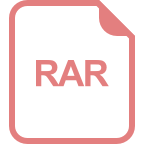
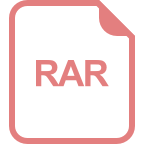
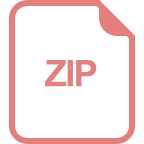
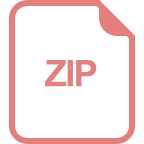
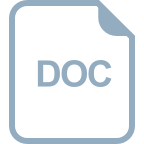
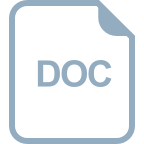