b=[2,1,5,4,3] H=4 dp = [0]*5 if H<normal_mean[0]: dp[0]=0 else: dp[0]=1 f = dp*b print(f)这段代码有什么问题报错TypeError: can't multiply sequence by non-int of type 'list'
时间: 2024-04-18 11:26:11 浏览: 85
这段代码的问题是在执行`f = dp*b`时,尝试将列表`b`乘以列表`dp`。然而,乘法操作符在这里无法直接应用于列表类型。
要解决这个问题,您可以使用循环遍历列表`dp`和`b`,并逐个相乘。这是代码的修正版本:
```python
b = [2, 1, 5, 4, 3]
H = 4
dp = [0] * 5
if H < normal_mean[0]:
dp[0] = 0
else:
dp[0] = 1
f = []
for i in range(len(dp)):
f.append(dp[i] * b[i])
print(f)
```
这样,乘法操作符将逐个相乘列表中的元素,得到一个新的列表`f`。
阅读全文
相关推荐
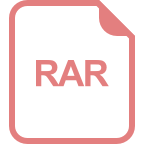
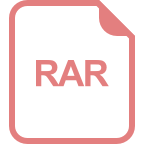
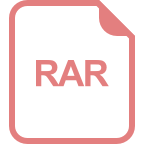
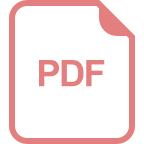
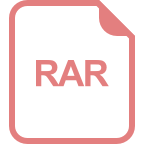
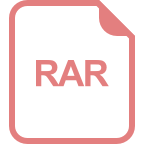
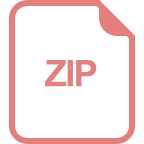
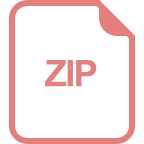
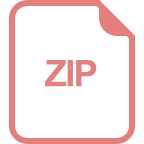
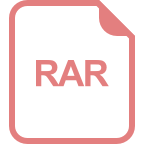
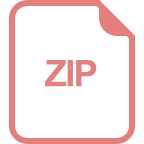
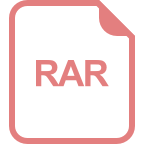
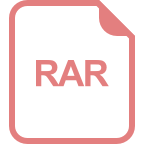
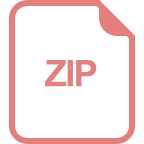
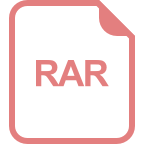
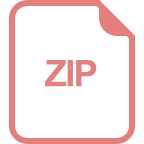
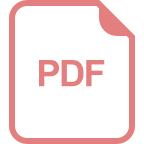
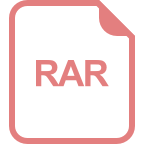