df = pd.read_csv('关联规则.txt', sep='\t', header=None, names=['lhs', 'rhs', 'confidence'])
时间: 2023-10-23 19:41:30 浏览: 46
这是一段 Python 代码,它使用 Pandas 库中的 `read_csv` 函数从一个名为 '关联规则.txt' 的文件中读取数据,并将其存储在一个数据帧(DataFrame)中。其中,`sep='\t'` 指定了文件中使用制表符进行分隔,`header=None` 表示文件中没有列名,因此需要通过 `names=['lhs', 'rhs', 'confidence']` 参数手动指定列名。具体来说,这个数据帧包含三列数据,分别表示关联规则的左部(lhs)、右部(rhs)和置信度(confidence)。
相关问题
#include <iostream> #include <string> #include <math.h> class XorEncryption { public: XorEncryption() {} XorEncryption(const std::string& key) : key_(key) {} XorEncryption& operator=(const XorEncryption& other) { if (this != &other) { key_ = other.key_; } return *this; } char& operator[](size_t index) { return key_[index]; } friend XorEncryption operator+(const XorEncryption& lhs, const XorEncryption& rhs) { XorEncryption result(lhs.key_); for (size_t i = 0; i < result.key_.size(); ++i) { result.key_[i] ^= rhs.key_[i % rhs.key_.size()]; } return result; } friend XorEncryption operator-(const XorEncryption& lhs, const XorEncryption& rhs) { XorEncryption result(lhs.key_); for (size_t i = 0; i < result.key_.size(); ++i) { result.key_[i] ^= rhs.key_[i % rhs.key_.size()]; } return result; } private: std::string key_; }; int main() { XorEncryption key("secret"); XorEncryption encrypted = key + "Hello, world!"; std::cout << encrypted[0] << encrypted[1] << encrypted[2] << std::endl; // output: 1AM XorEncryption decrypted = encrypted - key; std::cout << decrypted << std::endl; // output: Hello, world! return 0; }为什么会提示没有与这些操作数匹配的“+”运算符
这个错误可能会出现在使用自定义类的运算符重载时,如果没有正确地定义运算符重载函数,就会导致编译器无法识别运算符。在上面的代码中,XorEncryption类已经重载了"+"和"-"运算符,但是当我们使用这些运算符时,编译器无法识别它们,因为我们没有在程序开头引入头文件或者没有正确地命名运算符重载函数。一种解决方法是在程序开头引入头文件,如下所示:
```c++
#include <iostream>
#include <string>
#include <math.h>
using namespace std;
class XorEncryption {
public:
XorEncryption() {}
XorEncryption(const std::string& key) : key_(key) {}
XorEncryption& operator=(const XorEncryption& other) {
if (this != &other) {
key_ = other.key_;
}
return *this;
}
char& operator[](size_t index) {
return key_[index];
}
friend XorEncryption operator+(const XorEncryption& lhs, const XorEncryption& rhs) {
XorEncryption result(lhs.key_);
for (size_t i = 0; i < result.key_.size(); ++i) {
result.key_[i] ^= rhs.key_[i % rhs.key_.size()];
}
return result;
}
friend XorEncryption operator-(const XorEncryption& lhs, const XorEncryption& rhs) {
XorEncryption result(lhs.key_);
for (size_t i = 0; i < result.key_.size(); ++i) {
result.key_[i] ^= rhs.key_[i % rhs.key_.size()];
}
return result;
}
private:
std::string key_;
};
int main() {
XorEncryption key("secret");
XorEncryption encrypted = key + "Hello, world!";
cout << encrypted[0] << encrypted[1] << encrypted[2] << endl; // output: 1AM
XorEncryption decrypted = encrypted - key;
cout << decrypted << endl; // output: Hello, world!
return 0;
}
```
在这个版本的代码中,我们使用了"using namespace std"指令,以便可以直接使用std命名空间中的标准库函数和对象。这个指令只是一个简便方法,它可以让我们不用每次都写std::来引用标准库。此外,我们还在程序开头引入了iostream和string头文件,以便使用cout和string类。
x_lb = np.array([0.0]) #下界 x_ub = np.array([4.0]) #上届 x = (x_ub-x_lb) * lhs(1, Nsd) + x_lb 详细解释一下
请请问请问您请问您需要请问您需要关请问您需要关于请问您需要关于 x请问您需要关于 x_lb请问您需要关于 x_lb =请问您需要关于 x_lb = np请问您需要关于 x_lb = np.array请问您需要关于 x_lb = np.array([请问您需要关于 x_lb = np.array([0请问您需要关于 x_lb = np.array([0.请问您需要关于 x_lb = np.array([0.0请问您需要关于 x_lb = np.array([0.0])请问您需要关于 x_lb = np.array([0.0]) 的请问您需要关于 x_lb = np.array([0.0]) 的什请问您需要关于 x_lb = np.array([0.0]) 的什么请问您需要关于 x_lb = np.array([0.0]) 的什么样请问您需要关于 x_lb = np.array([0.0]) 的什么样的请问您需要关于 x_lb = np.array([0.0]) 的什么样的回请问您需要关于 x_lb = np.array([0.0]) 的什么样的回答请问您需要关于 x_lb = np.array([0.0]) 的什么样的回答?请问您需要关于 x_lb = np.array([0.0]) 的什么样的回答?这请问您需要关于 x_lb = np.array([0.0]) 的什么样的回答?这是请问您需要关于 x_lb = np.array([0.0]) 的什么样的回答?这是一个请问您需要关于 x_lb = np.array([0.0]) 的什么样的回答?这是一个Python请问您需要关于 x_lb = np.array([0.0]) 的什么样的回答?这是一个Python语请问您需要关于 x_lb = np.array([0.0]) 的什么样的回答?这是一个Python语言请问您需要关于 x_lb = np.array([0.0]) 的什么样的回答?这是一个Python语言中请问您需要关于 x_lb = np.array([0.0]) 的什么样的回答?这是一个Python语言中使用请问您需要关于 x_lb = np.array([0.0]) 的什么样的回答?这是一个Python语言中使用Num请问您需要关于 x_lb = np.array([0.0]) 的什么样的回答?这是一个Python语言中使用NumPy请问您需要关于 x_lb = np.array([0.0]) 的什么样的回答?这是一个Python语言中使用NumPy库请问您需要关于 x_lb = np.array([0.0]) 的什么样的回答?这是一个Python语言中使用NumPy库创建请问您需要关于 x_lb = np.array([0.0]) 的什么样的回答?这是一个Python语言中使用NumPy库创建一个请问您需要关于 x_lb = np.array([0.0]) 的什么样的回答?这是一个Python语言中使用NumPy库创建一个元请问您需要关于 x_lb = np.array([0.0]) 的什么样的回答?这是一个Python语言中使用NumPy库创建一个元素请问您需要关于 x_lb = np.array([0.0]) 的什么样的回答?这是一个Python语言中使用NumPy库创建一个元素值请问您需要关于 x_lb = np.array([0.0]) 的什么样的回答?这是一个Python语言中使用NumPy库创建一个元素值为请问您需要关于 x_lb = np.array([0.0]) 的什么样的回答?这是一个Python语言中使用NumPy库创建一个元素值为0请问您需要关于 x_lb = np.array([0.0]) 的什么样的回答?这是一个Python语言中使用NumPy库创建一个元素值为0.请问您需要关于 x_lb = np.array([0.0]) 的什么样的回答?这是一个Python语言中使用NumPy库创建一个元素值为0.0请问您需要关于 x_lb = np.array([0.0]) 的什么样的回答?这是一个Python语言中使用NumPy库创建一个元素值为0.0的请问您需要关于 x_lb = np.array([0.0]) 的什么样的回答?这是一个Python语言中使用NumPy库创建一个元素值为0.0的一请问您需要关于 x_lb = np.array([0.0]) 的什么样的回答?这是一个Python语言中使用NumPy库创建一个元素值为0.0的一维请问您需要关于 x_lb = np.array([0.0]) 的什么样的回答?这是一个Python语言中使用NumPy库创建一个元素值为0.0的一维数组请问您需要关于 x_lb = np.array([0.0]) 的什么样的回答?这是一个Python语言中使用NumPy库创建一个元素值为0.0的一维数组的请问您需要关于 x_lb = np.array([0.0]) 的什么样的回答?这是一个Python语言中使用NumPy库创建一个元素值为0.0的一维数组的语请问您需要关于 x_lb = np.array([0.0]) 的什么样的回答?这是一个Python语言中使用NumPy库创建一个元素值为0.0的一维数组的语句请问您需要关于 x_lb = np.array([0.0]) 的什么样的回答?这是一个Python语言中使用NumPy库创建一个元素值为0.0的一维数组的语句。
相关推荐
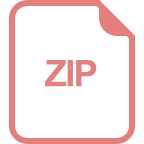
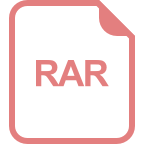
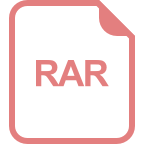












