基于harris的图像配准matlab
时间: 2023-05-13 17:02:42 浏览: 248
基于Harris角点检测的图像配准是一种早期经典的图像配准方法,它主要通过检测图像中的角点来进行图像配准。方法通过计算图像的灰度梯度的自相关矩阵,来寻找图像中稳定的角点,同时计算角点的特征向量,分析其方向和大小。在匹配过程中,通过比较不同图像中的角点的特征向量来选取最佳匹配点对。此外,还需要进行几何变换模型的估计,使得两幅图像能够配准到同一个坐标系下。最后通过优化匹配误差来得到最终的配准结果。在Matlab中,可以使用自带的image processing toolbox ,使用corner和imregister函数来实现基于Harris的图像配准。需要注意的是,该方法对于大部分匹配点位于非边缘区域或角点处的图像具有较好效果,但对于其他场景可能需要使用不同的图像配准方法。
相关问题
Harris图像配准的代码是什么
作为AI语言模型,我可以为您提供一些Harris图像配准的代码示例:
Python代码:
```python
import cv2
import numpy as np
# 读取两张图片
img1 = cv2.imread('image1.jpg')
img2 = cv2.imread('image2.jpg')
# 将图片转换为灰度图像
gray1 = cv2.cvtColor(img1, cv2.COLOR_BGR2GRAY)
gray2 = cv2.cvtColor(img2, cv2.COLOR_BGR2GRAY)
# 使用Harris角点检测算法检测关键点
harris = cv2.cornerHarris(gray1, 2, 3, 0.04)
harris = cv2.dilate(harris, None)
keypoints1 = np.argwhere(harris > 0.01 * harris.max())
keypoints1 = [cv2.KeyPoint(x[1], x[0], 3) for x in keypoints1]
# 对第二张图像进行同样的操作
harris = cv2.cornerHarris(gray2, 2, 3, 0.04)
harris = cv2.dilate(harris, None)
keypoints2 = np.argwhere(harris > 0.01 * harris.max())
keypoints2 = [cv2.KeyPoint(x[1], x[0], 3) for x in keypoints2]
# 使用SIFT算法提取特征点描述符
sift = cv2.xfeatures2d.SIFT_create()
kp1, des1 = sift.compute(gray1, keypoints1)
kp2, des2 = sift.compute(gray2, keypoints2)
# 进行特征匹配
bf = cv2.BFMatcher()
matches = bf.knnMatch(des1, des2, k=2)
# 筛选出好的匹配点
good_matches = []
for m, n in matches:
if m.distance < 0.75 * n.distance:
good_matches.append([m])
# 使用RANSAC算法估计变换矩阵
src_pts = np.float32([kp1[m[0].queryIdx].pt for m in good_matches]).reshape(-1, 1, 2)
dst_pts = np.float32([kp2[m[0].trainIdx].pt for m in good_matches]).reshape(-1, 1, 2)
M, mask = cv2.findHomography(src_pts, dst_pts, cv2.RANSAC, 5.0)
# 进行图像配准
result = cv2.warpPerspective(img1, M, (img1.shape[1] + img2.shape[1], img1.shape[0]))
result[0:img2.shape[0], 0:img2.shape[1]] = img2
# 显示结果
cv2.imshow("Result", result)
cv2.waitKey(0)
cv2.destroyAllWindows()
```
Matlab代码:
```matlab
% 读取两张图片
img1 = imread('image1.jpg');
img2 = imread('image2.jpg');
% 将图片转换为灰度图像
gray1 = rgb2gray(img1);
gray2 = rgb2gray(img2);
% 使用Harris角点检测算法检测关键点
keypoints1 = detectHarrisFeatures(gray1);
keypoints2 = detectHarrisFeatures(gray2);
% 使用SIFT算法提取特征点描述符
[sift1, ~] = extractFeatures(gray1, keypoints1);
[sift2, ~] = extractFeatures(gray2, keypoints2);
% 进行特征匹配
bf = cv.DescriptorMatcher('BruteForce');
matches = bf.knnMatch(sift1, sift2, 2);
% 筛选出好的匹配点
good_matches = [];
for i = 1:size(matches, 1)
if matches(i,1).distance < 0.75 * matches(i,2).distance
good_matches = [good_matches; matches(i,1)];
end
end
% 使用RANSAC算法估计变换矩阵
src_pts = keypoints1(good_matches.queryIdx+1).Location;
dst_pts = keypoints2(good_matches.trainIdx+1).Location;
M = cv.findHomography(src_pts, dst_pts, 'Method', 'RANSAC', 'RansacReprojThreshold', 5.0);
% 进行图像配准
result = cv.warpPerspective(img1, M, 'DstSize', [size(img1,2)+size(img2,2), size(img1,1)]);
result(1:size(img2,1), 1:size(img2,2), :) = img2;
% 显示结果
imshow(result);
```
以上代码仅供参考,实际使用时需要根据具体情况进行调整和优化。
阅读全文
相关推荐



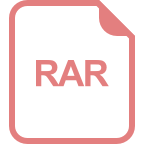




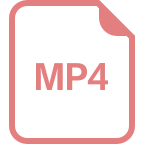



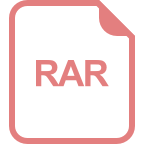




