python face_check
时间: 2023-05-12 14:01:32 浏览: 65
Python是一门强大的编程语言,可以用来编写多种应用。其中最受欢迎的是Python人脸识别技术。Python face_check是使用Python编写的人脸识别程序,可以用于图像识别、视频监控等场景。
Python face_check使用深度学习算法进行人脸检测,通过检测输入图像或视频帧中的人脸位置和特征,然后将其与数据集中的预定义特征进行比对,从而实现人脸验证、识别和比对等功能。
此外,Python face_check还可以集成其他技术,如活体检测和防照片攻击技术,从而提高精度和安全性。因此,Python face_check在物联网、安防和金融等领域应用广泛。
总之,Python face_check是一种快速、准确、可靠的人脸识别工具,可以满足多种业务场景需求。
相关问题
File "compare.py", line 23, in <module> is_same = face_recognition.compare_faces([image_1], image_2)[0] File "/home/zhxk/.local/lib/python3.8/site-packages/face_recognition/api.py", line 226, in compare_faces return list(face_distance(known_face_encodings, face_encoding_to_check) <= tolerance) File "/home/zhxk/.local/lib/python3.8/site-packages/face_recognition/api.py", line 75, in face_distance return np.linalg.norm(face_encodings - face_to_compare, axis=1) TypeError: unsupported operand type(s) for -: 'list' and 'NoneType'
这个错误提示说的是 face_encodings 和 face_to_compare 两个变量相减时,一个是列表类型,一个是 NoneType 类型,导致无法进行减法运算。可能是因为其中一个变量的值为 None,导致出现了这个错误。
建议在这段代码之前,检查一下 image_1 和 image_2 是否都被正确地读取和编码。另外,你可以在 `face_recognition.compare_faces([image_1], image_2)` 这行代码之前,加入以下语句来打印一下 image_1 和 image_2 的值,以及它们的类型,以便更好地排查问题:
```
print(type(image_1), type(image_2))
print(image_1, image_2)
```
希望这些能够帮助你找到问题所在。
face_recognition的参数介绍
face_recognition是一个用于人脸识别的Python库,其主要参数介绍如下:
1. face_recognition.face_locations(image, model='hog')
- image: 待识别的图片,可以是文件路径或者numpy数组
- model: 人脸检测模型,可以选择'hog'或者'cnn',默认为'hog'
2. face_recognition.face_encodings(face_image, known_face_locations=None, num_jitters=1, model='small')
- face_image: 待编码的人脸图片,可以是文件路径或者numpy数组
- known_face_locations: 已知的人脸位置,可以通过face_locations函数获取
- num_jitters: 编码时对人脸进行的随机扰动次数,可以提高编码的准确性,默认为1
- model: 人脸编码模型,可以选择'small'或者'large',默认为'small'
3. face_recognition.compare_faces(known_face_encodings, face_encoding_to_check, tolerance=0.6)
- known_face_encodings: 已知的人脸编码列表
- face_encoding_to_check: 待比较的人脸编码
- tolerance: 人脸编码之间的容差值,越小则匹配越严格,默认为0.6
4. face_recognition.load_image_file(file, mode='RGB')
- file: 待加载的图片文件路径
- mode: 图片加载模式,可以选择'RGB'或者'L',默认为'RGB'
5. face_recognition.face_distance(face_encodings, face_to_compare)
- face_encodings: 已知的人脸编码列表
- face_to_compare: 待比较的人脸编码
6. face_recognition.face_landmarks(face_image, face_locations=None, model='large')
- face_image: 待检测的人脸图片,可以是文件路径或者numpy数组
- face_locations: 已知的人脸位置,可以通过face_locations函数获取
- model: 人脸关键点检测模型,可以选择'large'或者'small',默认为'large'
7. face_recognition.face_encodings_batch(face_images, known_face_locations=None, num_jitters=1, model='small')
- face_images: 待编码的人脸图片列表,每个元素可以是文件路径或者numpy数组
- known_face_locations: 已知的人脸位置列表,可以通过face_locations函数获取
- num_jitters: 编码时对人脸进行的随机扰动次数,可以提高编码的准确性,默认为1
- model: 人脸编码模型,可以选择'small'或者'large',默认为'small'
相关推荐
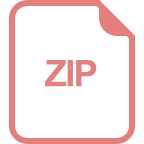
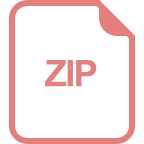
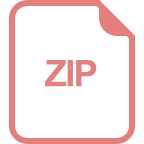













