sklearn.metrics.roc_auc_score
时间: 2023-04-22 21:02:05 浏览: 336
`sklearn.metrics.roc_auc_score` 是 Scikit-learn 中用于计算二分类模型 ROC 曲线下面积(AUC)的函数。
在使用该函数时,你需要提供真实标签 `y_true` 和预测标签 `y_pred`。其中,`y_true` 是一个长度为 `n_samples` 的一维数组,表示每个样本的真实标签;`y_pred` 是一个长度为 `n_samples` 的一维数组,表示模型对每个样本的预测标签。
下面是一个简单的例子:
```python
from sklearn.metrics import roc_auc_score
y_true = [0, 0, 1, 1]
y_pred = [0.1, 0.4, 0.35, 0.8]
roc_auc = roc_auc_score(y_true, y_pred)
print("ROC AUC score:", roc_auc)
```
在这个例子中,`y_true` 表示四个样本的真实标签,分别为 0、0、1、1;`y_pred` 表示模型对这四个样本的预测概率,分别为 0.1、0.4、0.35、0.8。函数的返回值 `roc_auc` 表示模型的 ROC 曲线下面积,即 0.75。
相关问题
Sklearn.metrics.roc_auc_score模块中的源代码
以下是sklearn.metrics.roc_auc_score模块的源代码:
```python
def roc_auc_score(y_true, y_score, average='macro', sample_weight=None,
max_fpr=None, multi_class='raise', labels=None):
"""Compute Area Under the Receiver Operating Characteristic Curve (ROC AUC)
from prediction scores.
Note: this implementation can be used with binary, multiclass and
multilabel classification, but some restrictions apply (see Parameters).
Read more in the :ref:`User Guide <roc_metrics>`.
Parameters
----------
y_true : array-like of shape (n_samples,) or (n_samples, n_classes)
True labels or binary label indicators. The binary and multiclass cases
expect labels with shape (n_samples,) while the multilabel case expects
binary label indicators with shape (n_samples, n_classes).
y_score : array-like of shape (n_samples,) or (n_samples, n_classes)
Target scores. In the binary and multilabel cases, these can be either
probability estimates or non-thresholded decision values (as returned
by `decision_function` on some classifiers). In the multiclass case,
these must be probability estimates which sum to 1. The binary case
expects a shape (n_samples,), and the scores must be the scores of
the class with the greater label. The multiclass and multilabel
cases expect a shape (n_samples, n_classes).
average : {'micro', 'macro', 'samples', 'weighted'} or None, \
default='macro'
If ``None``, the scores for each class are returned. Otherwise,
this determines the type of averaging performed on the data:
``'micro'``:
Calculate metrics globally by counting the total true positives,
false negatives and false positives.
``'macro'``:
Calculate metrics for each label, and find their unweighted
mean. This does not take label imbalance into account.
``'weighted'``:
Calculate metrics for each label, and find their average, weighted
by support (the number of true instances for each label). This
alters 'macro' to account for label imbalance; it can result in an
F-score that is not between precision and recall.
``'samples'``:
Calculate metrics for each instance, and find their average
(only meaningful for multilabel classification).
sample_weight : array-like of shape (n_samples,), default=None
Sample weights.
max_fpr : float or None, default=None
If not ``None``, the standardized partial AUC [2]_ over the range
[0, max_fpr] is returned. For the multiclass case, ``max_fpr``
should be either ``None`` or ``1.0`` as partial AUC makes
sense for binary classification only.
multi_class : {'raise', 'ovr', 'ovo'}, default='raise'
Multiclass only. Determines the type of configuration to use.
The default value raises an error, so either ``'ovr'`` or
``'ovo'`` must be passed explicitly.
``'ovr'``:
Computes ROC curve independently for each class. For each class,
the binary problem y_true == i or not is solved and the
corresponding ROC curve is computed and averaged across
classes. This is a commonly used strategy for multiclass
or multi-label classification problems.
``'ovo'``:
Computes pairwise ROC curve for each pair of classes. For each
pair of classes, the binary problem y_true == i or y_true == j
is solved and the corresponding ROC curve is computed. The
micro-averaged ROC curve is computed from the individual curves
and hence is agnostic to the class balance.
labels : array-like of shape (n_classes,), default=None
Multiclass only. List of labels to index ``y_score`` used for
multiclass. If ``None``, the lexical order of ``y_true`` is used to
index ``y_score``.
Returns
-------
auc : float or dict (if ``multi_class`` is ``'ovo'`` or ``'ovr'``)
AUC of the ROC curves.
If ``multi_class`` is ``'ovr'``, then returns an array of shape
``(n_classes,)`` such that each element corresponds to the AUC of
the ROC curve for a specific class.
If ``multi_class`` is ``'ovo'``, then returns a dict where the keys
are ``(i, j)`` tuples and the values are the AUCs of the ROC curve
for the binary problem of predicting class ``i`` vs. class ``j``.
See also
--------
roc_curve : Compute Receiver operating characteristic (ROC) curve.
roc_auc : Compute Area Under the Receiver Operating Characteristic Curve
(ROC AUC) from prediction scores
Examples
--------
>>> import numpy as np
>>> from sklearn.metrics import roc_auc_score
>>> y_true = np.array([0, 0, 1, 1])
>>> y_scores = np.array([0.1, 0.4, 0.35, 0.8])
>>> roc_auc_score(y_true, y_scores)
0.75
>>> y_true = np.array([0, 0, 1, 1])
>>> y_scores = np.array([[0.1, 0.9], [0.4, 0.6], [0.35, 0.65], [0.8, 0.2]])
>>> roc_auc_score(y_true, y_scores, multi_class='ovo')
0.6666666666666667
>>> roc_auc_score(y_true, y_scores[:, 1])
0.75
"""
# validation of the input y_score
if not (y_true.shape == y_score.shape):
raise ValueError("y_true and y_score have different shape.")
if (not is_multilabel(y_true) and not is_multiclass(y_true)):
# roc_auc_score only supports binary and multiclass classification
# for the time being
if len(np.unique(y_true)) == 2:
# Only one class present in y_true. ROC AUC score is not defined
# in that case. Note that raising an error is consistent with the
# deprecated roc_auc_score behavior.
raise ValueError(
"ROC AUC score is not defined in that case: "
"y_true contains only one label ({0}).".format(
format_label(y_true[0])
)
)
else:
raise ValueError(
"ROC AUC score is not defined in that case: "
"y_true has {0} unique labels. ".format(len(np.unique(y_true))) +
"ROC AUC score is defined only for binary or multiclass "
"classification where the number of classes is greater than "
"one."
)
if multi_class == 'raise':
raise ValueError("multi_class must be in ('ovo', 'ovr')")
elif multi_class == 'ovo':
if is_multilabel(y_true):
# check if max_fpr is valid in this case
if max_fpr is not None and (max_fpr == 0 or max_fpr > 1):
raise ValueError("Expected max_fpr in range (0, 1], got: %f" %
max_fpr)
return _multiclass_roc_auc_score_ovr(y_true, y_score,
average, sample_weight,
max_fpr=max_fpr)
else:
return _binary_roc_auc_score(y_true, y_score, average,
sample_weight, max_fpr=max_fpr)
elif multi_class == 'ovr':
if is_multilabel(y_true):
return _multilabel_roc_auc_score_ovr(y_true, y_score,
average, sample_weight)
else:
return _multiclass_roc_auc_score_ovr(y_true, y_score,
average, sample_weight,
labels=labels)
else:
raise ValueError("Invalid multi_class parameter: {0}".format(multi_class))
```
这段代码实现了计算ROC AUC的功能,支持二元、多类和多标签分类。其中,分为'ovo'和'ovr'两种多类模式,'ovo'表示一对一,'ovr'表示一对多。
sklearn.metrics.roc_auc_score和sklearn.metrics.roc_curve怎么用,参数都有哪些,举个例子应用一下说明
首先我们需要了解什么是ROC曲线以及AUC,ROC曲线指的是接收者操作特征曲线(Receiver Operating Characteristic Curve),常用于二分类问题中评价模型的性能。ROC曲线的横轴是假正率(False Positive Rate, FPR),纵轴是真正率(True Positive Rate, TPR),而AUC(Area Under Curve)指的是ROC曲线下的面积,用于衡量模型预测准确性的指标。AUC的取值范围在0.5到1之间,AUC越大,模型的性能越好。
接下来我们来看看如何使用`sklearn.metrics`中的`roc_auc_score`和`roc_curve`函数。
`roc_auc_score`函数的参数说明:
```python
sklearn.metrics.roc_auc_score(y_true, y_score, average='macro', sample_weight=None, max_fpr=None)
```
- `y_true`:真实标签,可以是一个一维数组或列表。
- `y_score`:模型预测的得分,与`y_true`的长度相同。
- `average`:指定AUC计算的方式。默认为`'macro'`,即计算每个类别的AUC值并求平均值。如果设为`'micro'`,则将所有数据合并计算一个AUC值。如果设为`None`,则返回所有类别的AUC值。
- `sample_weight`:样本权重,可以是一个一维数组或列表,长度与`y_true`相同。
- `max_fpr`:最大假正率,用于计算部分AUC值。默认为`None`,即计算完整的ROC曲线下的AUC值。
`roc_curve`函数的参数说明:
```python
sklearn.metrics.roc_curve(y_true, y_score, pos_label=None, sample_weight=None, drop_intermediate=True)
```
- `y_true`:真实标签,可以是一个一维数组或列表。
- `y_score`:模型预测的得分,与`y_true`的长度相同。
- `pos_label`:指定正例的标签值。默认为`None`,即将最大的标签设为正例。
- `sample_weight`:样本权重,可以是一个一维数组或列表,长度与`y_true`相同。
- `drop_intermediate`:是否在计算过程中舍弃中间结果。默认为`True`,即只返回阈值和对应的FPR和TPR值,且只包括第一个和最后一个元素。
下面给出一个例子,使用`sklearn.metrics`中的`roc_auc_score`和`roc_curve`函数来评价一个二分类模型的性能:
```python
from sklearn.datasets import make_classification
from sklearn.linear_model import LogisticRegression
from sklearn.model_selection import train_test_split
from sklearn.metrics import roc_auc_score, roc_curve
import matplotlib.pyplot as plt
# 生成随机数据
X, y = make_classification(n_samples=1000, n_features=10, n_classes=2, random_state=1)
# 划分训练集和测试集
X_train, X_test, y_train, y_test = train_test_split(X, y, test_size=0.3, random_state=1)
# 训练模型
model = LogisticRegression()
model.fit(X_train, y_train)
# 预测测试集
y_pred = model.predict(X_test)
y_score = model.predict_proba(X_test)[:, 1]
# 计算AUC
auc = roc_auc_score(y_test, y_score)
print('AUC:', auc)
# 计算ROC曲线
fpr, tpr, thresholds = roc_curve(y_test, y_score)
# 绘制ROC曲线
plt.plot(fpr, tpr, label='ROC curve (area = %0.2f)' % auc)
plt.plot([0, 1], [0, 1], 'k--')
plt.xlim([0.0, 1.0])
plt.ylim([0.0, 1.05])
plt.xlabel('False Positive Rate')
plt.ylabel('True Positive Rate')
plt.title('Receiver operating characteristic example')
plt.legend(loc="lower right")
plt.show()
```
在上面的例子中,我们使用`make_classification`函数生成了1000个样本,10个特征和2个类别。然后使用`train_test_split`函数将数据划分为训练集和测试集。接着训练一个逻辑回归模型,并预测测试集的标签和得分。最后使用`roc_auc_score`函数计算AUC值,使用`roc_curve`函数计算ROC曲线的FPR和TPR,并绘制ROC曲线图。
运行上述代码,可以得到以下输出:
```
AUC: 0.9311666666666667
```
同时还会出现一个ROC曲线的图像。可以看到该模型的AUC值较高,ROC曲线也比较靠近左上角,说明该模型的预测准确性较好。
阅读全文
相关推荐
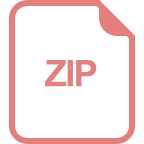
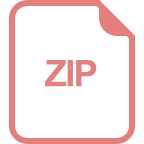
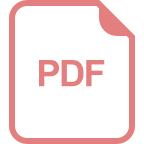









