python中DecisionTreeClassifier的max_depth调整示范
时间: 2024-04-07 10:07:28 浏览: 18
决策树分类器 DecisionTreeClassifier 是一种常见的机器学习算法,其参数 max_depth 可以调整决策树的最大深度。下面是一个示范代码,展示如何在 Python 中使用 DecisionTreeClassifier 并调整 max_depth 参数。
首先,我们需要导入必要的库和数据集:
```python
from sklearn.datasets import load_iris
from sklearn.tree import DecisionTreeClassifier
from sklearn.model_selection import train_test_split
from sklearn.metrics import accuracy_score
iris = load_iris()
X = iris.data
y = iris.target
```
然后,我们将数据集分为训练集和测试集,使用 DecisionTreeClassifier 创建分类器,并在训练集上训练模型:
```python
X_train, X_test, y_train, y_test = train_test_split(X, y, test_size=0.2, random_state=42)
clf = DecisionTreeClassifier(max_depth=3, random_state=42)
clf.fit(X_train, y_train)
```
在这里,我们将 max_depth 设置为 3。接下来,我们使用测试集评估模型的性能:
```python
y_pred = clf.predict(X_test)
accuracy = accuracy_score(y_test, y_pred)
print("Accuracy:", accuracy)
```
最后,我们可以使用不同的 max_depth 值进行实验,并比较模型的准确性。例如,我们可以将 max_depth 设置为 4:
```python
clf = DecisionTreeClassifier(max_depth=4, random_state=42)
clf.fit(X_train, y_train)
y_pred = clf.predict(X_test)
accuracy = accuracy_score(y_test, y_pred)
print("Accuracy:", accuracy)
```
通过比较不同 max_depth 值下的准确性,我们可以找到一个最佳值来优化模型性能。
相关推荐
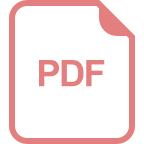
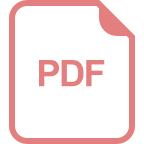
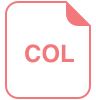
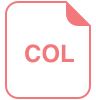
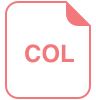
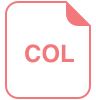
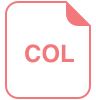









