生成python代码,对Excel表格中A列相同的行,验证B列是否只有一个数值“1”,并将不符合条件的行返回。
时间: 2024-10-18 20:11:12 浏览: 40
为了实现这个功能,我们可以使用Python的pandas库来操作Excel数据。下面是一个示例代码片段,它读取Excel文件,检查A列是否有重复值,并在B列找到所有"1"的位置,然后筛选出那些B列不是唯一的"1"的行:
```python
import pandas as pd
def check_excel(file_path):
# 读取Excel文件
df = pd.read_excel(file_path)
# 验证A列是否有重复
dup_rows = df[df['A'].duplicated()]
# 检查B列是否只有一个1
def has_unique_one(row):
return len(row[row['B'] == 1]) == 1
# 应用函数到DataFrame并筛选结果
filtered_rows = dup_rows[dup_rows.apply(has_unique_one, axis=1)]
return filtered_rows
# 使用函数并打印结果
result = check_excel('your_file.xlsx')
print(result)
```
在这个代码中,`your_file.xlsx`需要替换为你实际的Excel文件路径。运行这段代码后,你会得到一个包含A列中有重复值并且B列不只包含一个"1"的行的数据框。
相关问题
生成python代码,对Excel表格中A列相同的行,验证B列是否只有一个数值“1”,并将错误的行信息返回。
你可以使用pandas库来处理这个问题。首先,你需要安装这个库(如果你还没有安装),可以使用`pip install pandas`命令。下面是一个简单的Python脚本,它会读取Excel文件,检查A列是否有重复值,如果B列对应这些重复的A值有多个"1",则返回相应的行索引:
```python
import pandas as pd
def check_excel_file(file_path):
# 读取Excel文件
df = pd.read_excel(file_path)
# 验证每个唯一 A 列值对应的 B 列
for group_name, group in df.groupby('A'):
if (group['B'] != 1).sum() > 1:
# 如果 B 列中有超过一个非 1 的值,返回该组的行索引
error_rows = group.index.tolist()
yield error_rows
# 使用函数并打印错误行
file_path = "your_excel_file.xlsx" # 替换为你需要检查的Excel文件路径
for error_rows in check_excel_file(file_path):
print(f"发现错误行:{error_rows}")
#
使用python导入excel表格数据,根据y=wx+b,产生数据y,并用np.random.rand()添加随机噪声,y=wx+b+噪声,得到数据集(x,y)
在Python中,我们可以使用pandas库读取Excel表格的数据,NumPy库处理数值计算,以及matplotlib或seaborn库可视化结果。首先,你需要安装`pandas`, `numpy`和`openpyxl`这三个库,如果还没有安装可以使用pip进行安装:
```bash
pip install pandas numpy openpyxl
```
下面是一个步骤来完成你的需求:
1. **导入必要的库**:
```python
import pandas as pd
import numpy as np
from sklearn.model_selection import train_test_split # 用于划分训练集和测试集
```
2. **加载Excel数据**:
```python
# 假设你的Excel文件名为'data.xlsx',并且数据在Sheet1的第一列是x,第二列是w,第三列是b
df = pd.read_excel('data.xlsx', sheet_name='Sheet1')
x_data = df.iloc[:, :-1] # 剔除最后一列(即b)
y_data = df.iloc[:, -1]
```
3. **生成数据并添加随机噪声**:
```python
def add_noise(y, noise_level=0.1):
return y + noise_level * np.random.randn(len(y)) # np.random.randn生成标准正态分布的随机数
y_with_noise = add_noise(y_data)
```
4. **将数据划分为训练集和测试集**:
```python
x_train, x_test, y_train, y_test = train_test_split(x_data, y_with_noise, test_size=0.2, random_state=42)
```
5. **整理成适合模型训练的形式**:
```python
x_train = x_train.values.reshape(-1, 1) # 将一维数组转换为二维
x_test = x_test.values.reshape(-1, 1)
# 对于线性回归模型,通常不会用到加法项(b),因为它是模型参数的一部分
# 如果需要拟合,可以创建一个全零向量作为b的初始值
# b = np.zeros((1,))
# x_train = np.concatenate((x_train, np.ones_like(x_train)), axis=1) # 添加常数列(ones)
```
现在你已经有了一个带随机噪声的数据集(x_train, y_train)和验证集(x_test, y_test),可以根据需求建立简单的线性回归模型或者深度学习模型。
阅读全文
相关推荐


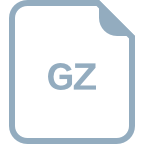













