sklearn调用importance的python代码
时间: 2024-10-18 16:18:30 浏览: 23
在Scikit-Learn库中,`importance`通常用于特征选择或者理解模型中各个特征的重要性,比如决策树、随机森林等集成学习模型。这里我们以随机森林为例,因为它们内置了特征重要性的计算。
假设你已经有一个训练好的随机森林模型 `rf_model`,并且数据集有特征名列表 `feature_names`,你可以通过以下Python代码获取特征的重要性:
```python
from sklearn.ensemble import RandomForestClassifier
# 假设已经拟合好了模型
rf_model = RandomForestClassifier()
rf_model.fit(X_train, y_train)
# 获取特征的重要性得分
importances = rf_model.feature_importances_
# 打印特征名称和对应的重要性
for feature, importance in zip(feature_names, importances):
print(f"特征: {feature}, 重要性: {importance}")
# 或者将结果转换成DataFrame方便查看
importance_df = pd.DataFrame({'Features': feature_names, 'Importance': importances})
print(importance_df)
```
相关问题
【实验项目3】 1.学习并调用AdaBoostClassifier处理预测问题,并尝试用于真实数据集(不选择boston波士顿房价数据集),并提供完整的python代码,可适当可视化; 2.学习并调用AdaBoostRegressor处理预测问题,并尝试用于真实数据集(不选择boston波士顿房价数据集),并提供完整的python代码,可适当可视化;
1. AdaBoostClassifier
首先,我们需要导入所需的库和数据集。这里我们选用了sklearn库中的乳腺癌数据集。
```python
from sklearn.datasets import load_breast_cancer
from sklearn.ensemble import AdaBoostClassifier
from sklearn.model_selection import train_test_split
from sklearn.metrics import confusion_matrix, classification_report
import matplotlib.pyplot as plt
import seaborn as sns
cancer = load_breast_cancer()
X = cancer.data
y = cancer.target
```
接下来,我们将数据集划分为训练集和测试集,并用AdaBoostClassifier进行训练和预测。
```python
X_train, X_test, y_train, y_test = train_test_split(X, y, test_size=0.2, random_state=42)
ada_clf = AdaBoostClassifier(n_estimators=100)
ada_clf.fit(X_train, y_train)
y_pred = ada_clf.predict(X_test)
```
最后,我们可以使用混淆矩阵和分类报告来评估模型的性能,并绘制特征的重要性图表。
```python
conf_mat = confusion_matrix(y_test, y_pred)
sns.heatmap(conf_mat, annot=True, cmap='Blues')
plt.xlabel('Predicted')
plt.ylabel('Actual')
plt.show()
print(classification_report(y_test, y_pred))
plt.figure(figsize=(10,6))
plt.bar(range(len(ada_clf.feature_importances_)), ada_clf.feature_importances_)
plt.xlabel('Features')
plt.ylabel('Importance')
plt.show()
```
完整的代码如下:
```python
from sklearn.datasets import load_breast_cancer
from sklearn.ensemble import AdaBoostClassifier
from sklearn.model_selection import train_test_split
from sklearn.metrics import confusion_matrix, classification_report
import matplotlib.pyplot as plt
import seaborn as sns
cancer = load_breast_cancer()
X = cancer.data
y = cancer.target
X_train, X_test, y_train, y_test = train_test_split(X, y, test_size=0.2, random_state=42)
ada_clf = AdaBoostClassifier(n_estimators=100)
ada_clf.fit(X_train, y_train)
y_pred = ada_clf.predict(X_test)
conf_mat = confusion_matrix(y_test, y_pred)
sns.heatmap(conf_mat, annot=True, cmap='Blues')
plt.xlabel('Predicted')
plt.ylabel('Actual')
plt.show()
print(classification_report(y_test, y_pred))
plt.figure(figsize=(10,6))
plt.bar(range(len(ada_clf.feature_importances_)), ada_clf.feature_importances_)
plt.xlabel('Features')
plt.ylabel('Importance')
plt.show()
```
2. AdaBoostRegressor
同样地,我们需要导入所需的库和数据集。这里我们选用了sklearn库中的波士顿房价数据集。
```python
from sklearn.datasets import load_boston
from sklearn.ensemble import AdaBoostRegressor
from sklearn.model_selection import train_test_split
from sklearn.metrics import mean_squared_error
import matplotlib.pyplot as plt
import seaborn as sns
import numpy as np
boston = load_boston()
X = boston.data
y = boston.target
```
接下来,我们将数据集划分为训练集和测试集,并用AdaBoostRegressor进行训练和预测。
```python
X_train, X_test, y_train, y_test = train_test_split(X, y, test_size=0.2, random_state=42)
ada_reg = AdaBoostRegressor(n_estimators=100)
ada_reg.fit(X_train, y_train)
y_pred = ada_reg.predict(X_test)
```
最后,我们可以使用均方误差来评估模型的性能,并绘制特征的重要性图表。
```python
print('Mean Squared Error: ', mean_squared_error(y_test, y_pred))
plt.figure(figsize=(10,6))
plt.bar(range(len(ada_reg.feature_importances_)), ada_reg.feature_importances_)
plt.xlabel('Features')
plt.ylabel('Importance')
plt.show()
```
完整的代码如下:
```python
from sklearn.datasets import load_boston
from sklearn.ensemble import AdaBoostRegressor
from sklearn.model_selection import train_test_split
from sklearn.metrics import mean_squared_error
import matplotlib.pyplot as plt
import seaborn as sns
import numpy as np
boston = load_boston()
X = boston.data
y = boston.target
X_train, X_test, y_train, y_test = train_test_split(X, y, test_size=0.2, random_state=42)
ada_reg = AdaBoostRegressor(n_estimators=100)
ada_reg.fit(X_train, y_train)
y_pred = ada_reg.predict(X_test)
print('Mean Squared Error: ', mean_squared_error(y_test, y_pred))
plt.figure(figsize=(10,6))
plt.bar(range(len(ada_reg.feature_importances_)), ada_reg.feature_importances_)
plt.xlabel('Features')
plt.ylabel('Importance')
plt.show()
```
随机森林进行特征选择python代码
### 回答1:
随机森林是决策树算法的一种集成算法,可以用于特征选择和分类问题。在这里,我们使用Python的scikit-learn库来实现随机森林进行特征选择的代码。
首先,我们需要载入数据集和必要的库:
```python
from sklearn.datasets import load_iris
from sklearn.ensemble import RandomForestClassifier
import numpy as np
iris = load_iris() # 载入数据集
X = iris.data
y = iris.target
```
然后我们可以定义一个随机森林分类器并训练它:
```python
rf = RandomForestClassifier(n_estimators=100) # 定义一个包含100棵树的随机森林分类器
rf.fit(X, y) # 训练随机森林分类器
```
接下来,我们可以调用feature_importances_属性来获取每个特征对预测结果的重要性:
```python
importances = rf.feature_importances_ # 获取特征重要性
indices = np.argsort(importances)[::-1] # 将重要性从高到低排序
```
最后,我们可以输出每个特征的重要性排名和重要性指标:
```python
for f in range(X.shape[1]):
print("%2d) %-*s %f" % (f + 1, 30, iris.feature_names[indices[f]], importances[indices[f]]))
```
上述代码将按照从最重要到最不重要的顺序输出每个特征的贡献百分比。我们可以根据正向选择、反向选择或者一个自定义的模型选择特征。
值得注意的是,随机森林是一种自带特征选择能力的算法,因此在特征选择时不需要手动选择特征。如果把随机森林用于分类问题,它也可以自动选择最优特征,并把其它无用的特征剔除掉,从而提高模型的精度和效率。
### 回答2:
随机森林是一种常用的机器学习算法,可以用于分类和回归问题。在实际应用中,我们需要从大量的特征中选择出最为关键的特征,这时候可以使用随机森林进行特征选择。
在Python中,可以使用scikit-learn库中的随机森林算法进行特征选择。具体代码如下:
首先导入必要的库:
import numpy as np
import pandas as pd
from sklearn.ensemble import RandomForestClassifier
接着导入数据并进行预处理:
# 导入数据
data = pd.read_csv('data.csv')
# 将数据分为特征和标签
X = data.drop('label', axis=1) # 特征
y = data['label'] # 标签
# 将标签编码为数字
y = pd.factorize(y)[0]
# 将数据划分为训练集和测试集
from sklearn.model_selection import train_test_split
X_train, X_test, y_train, y_test = train_test_split(X, y, test_size=0.2)
接着使用随机森林进行特征选择:
# 创建随机森林分类器
rf = RandomForestClassifier(n_estimators=100)
# 训练模型
rf.fit(X_train, y_train)
# 提取特征的重要性得分
feature_importances = rf.feature_importances_
# 将得分与特征名一一对应
features = X.columns.tolist()
feature_importances = pd.DataFrame({'feature': features, 'importance': feature_importances})
# 根据重要性得分排序
feature_importances = feature_importances.sort_values('importance', ascending=False).reset_index(drop=True)
# 输出排序后的特征重要性得分
print(feature_importances)
根据特征的重要性得分可以判断出哪些特征对于分类更为重要,这样可以帮助我们选择最为关键的特征来进行分析和建模。
### 回答3:
随机森林是常用的机器学习算法之一,可以用于分类和回归问题。特征选择是机器学习中非常重要的一个步骤,它可以在不影响模型性能的情况下,提高模型的训练效率和精度。下面是关于随机森林进行特征选择的Python代码。
首先需要导入所需的库:
```
import numpy as np
import pandas as pd
from sklearn.ensemble import RandomForestClassifier
```
然后加载数据,获取特征和标签:
```
# 加载数据
data = pd.read_csv('data.csv')
# 获取特征和标签
X = data.drop(['label'], axis=1)
y = data['label']
```
接着将数据集分为训练集和测试集:
```
from sklearn.model_selection import train_test_split
X_train, X_test, y_train, y_test = train_test_split(X, y, test_size=0.3)
```
随机森林需要设置一些参数,不同的数据集可能需要不同的参数值。这里我们设置n_estimators为100,表示森林中有100棵树。
```
# 设置随机森林分类器参数
rf = RandomForestClassifier(n_estimators=100, random_state=1)
```
然后使用fit方法训练随机森林模型:
```
# 训练随机森林模型
rf.fit(X_train, y_train)
```
随机森林在训练过程中会计算每个特征的重要性,并将其存储在feature_importances_属性中。为了查看每个特征的重要性,可以使用如下代码:
```
# 查看特征重要性
importances = rf.feature_importances_
indices = np.argsort(importances)[::-1]
for f in range(X_train.shape[1]):
print("%2d) %-*s %f" % (f + 1, 30, X_train.columns[indices[f]], importances[indices[f]]))
```
该代码会输出每个特征的重要性,越重要的特征排名越靠前。
另外,也可以使用SelectFromModel类来选择重要特征:
```
from sklearn.feature_selection import SelectFromModel
sfm = SelectFromModel(rf, threshold=0.1)
sfm.fit(X_train, y_train)
X_important_train = sfm.transform(X_train)
X_important_test = sfm.transform(X_test)
```
以上代码会根据重要性阈值选择重要特征,并将其存储在新的变量中。之后可以使用X_important_train和y_train来训练模型。
总之,随机森林是一种有效的特征选择方法,通过计算每个特征的重要性,可以选择重要特征提高模型的准确度和效率。
阅读全文
相关推荐
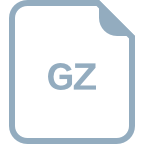
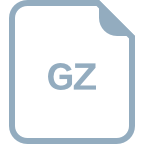















