pinky is not in the sudoers file. This incident will be reported.
时间: 2023-11-16 13:49:11 浏览: 87
根据提供的引用内容,"pinky is not in the sudoers file. This incident will be reported." 是一条错误消息,通常是在Linux系统中使用sudo命令时出现的。这个错误消息意味着用户"Pinky"没有被授权执行sudo命令的权限。该错误消息将被记录并报告给系统管理员。
相关问题
mediapipe怎么检测是左手还是右手,请给出示例代码
以下是一个简单的示例代码,展示了如何使用MediaPipe的Hands模型检测手的左右:
```python
import cv2
import mediapipe as mp
mp_drawing = mp.solutions.drawing_utils
mp_hands = mp.solutions.hands
cap = cv2.VideoCapture(0)
with mp_hands.Hands(
min_detection_confidence=0.5,
min_tracking_confidence=0.5) as hands:
while cap.isOpened():
success, image = cap.read()
if not success:
print("Ignoring empty camera frame.")
continue
# Convert the BGR image to RGB before processing.
image = cv2.cvtColor(image, cv2.COLOR_BGR2RGB)
# To improve performance, optionally mark the image as not writeable to pass by reference.
image.flags.writeable = False
results = hands.process(image)
# Draw the hand annotations on the image.
image.flags.writeable = True
image = cv2.cvtColor(image, cv2.COLOR_RGB2BGR)
if results.multi_hand_landmarks:
for hand_landmarks in results.multi_hand_landmarks:
mp_drawing.draw_landmarks(
image, hand_landmarks, mp_hands.HAND_CONNECTIONS)
# Check if the hand is left or right
if hand_landmarks.landmark[mp_hands.HandLandmark.INDEX_FINGER_TIP].x < hand_landmarks.landmark[mp_hands.HandLandmark.PINKY_TIP].x:
print("Right hand detected.")
else:
print("Left hand detected.")
cv2.imshow('MediaPipe Hands', image)
if cv2.waitKey(5) & 0xFF == 27:
break
cap.release()
```
在上面的代码中,我们首先创建了一个 `cv2.VideoCapture` 对象,用于捕获摄像头的视频流。然后,我们创建了一个 `mp_hands.Hands` 对象,用于加载MediaPipe的Hands模型。在循环中,我们从摄像头中读取一帧图像,并将其转换为RGB格式。然后,我们调用 `hands.process` 方法,传入图像,以便使用Hands模型检测手部关键点。如果检测到手部关键点,我们会遍历所有检测到的手,并在图像中绘制出手部关键点的位置。最后,我们判断手的左右位置,并输出结果。
需要注意的是,上述代码中的判断手的左右位置的方法仅适用于手掌朝下的情况。如果手掌朝上,需要修改判断条件。此外,还可以使用更复杂的方法来判断手的左右位置,例如使用机器学习模型来分类左右手。
mediapipe简单手势判断
Mediapipe是一个Google开源的跨平台机器学习框架,可以用于构建各种视觉和媒体处理应用程序。Mediapipe中提供了手部关键点检测模型,可以用于手势识别。具体实现步骤如下:
1. 使用Mediapipe中的HandLandmark模型检测手部关键点。
2. 根据手部关键点的位置计算手指的弯曲角度。
3. 根据手指的弯曲角度判断手势类型。
以下是一个简单的手势判断代码示例:
```python
import cv2
import mediapipe as mp
import math
mp_drawing = mp.solutions.drawing_utils
mp_hands = mp.solutions.hands
# 初始化HandLandmark模型
hands = mp_hands.Hands(static_image_mode=False, max_num_hands=2, min_detection_confidence=0.5)
# 定义手势类型
GESTURES = {
0: 'Fist',
1: 'Five',
2: 'Ok',
3: 'Peace',
4: 'Thumbs up'
}
# 计算两个点之间的距离
def distance(p1, p2):
return math.sqrt((p1.x - p2.x) ** 2 + (p1.y - p2.y) ** 2)
# 计算三个点之间的夹角
def angle(p1, p2, p3):
a = distance(p2, p3)
b = distance(p1, p2)
c = distance(p1, p3)
return math.degrees(math.acos((a ** 2 + b ** 2 - c ** 2) / (2 * a * b)))
cap = cv2.VideoCapture(0)
while cap.isOpened():
success, image = cap.read()
if not success:
break
# 转换为RGB格式
image = cv2.cvtColor(image, cv2.COLOR_BGR2RGB)
# 检测手部关键点
results = hands.process(image)
# 绘制手部关键点
if results.multi_hand_landmarks:
for hand_landmarks in results.multi_hand_landmarks:
mp_drawing.draw_landmarks(image, hand_landmarks, mp_hands.HAND_CONNECTIONS)
# 计算手指的弯曲角度
thumb_angle = angle(hand_landmarks.landmark[mp_hands.HandLandmark.THUMB_TIP],
hand_landmarks.landmark[mp_hands.HandLandmark.THUMB_IP],
hand_landmarks.landmark[mp_hands.HandLandmark.THUMB_MCP])
index_angle = angle(hand_landmarks.landmark[mp_hands.HandLandmark.INDEX_FINGER_TIP],
hand_landmarks.landmark[mp_hands.HandLandmark.INDEX_FINGER_PIP],
hand_landmarks.landmark[mp_hands.HandLandmark.INDEX_FINGER_MCP])
middle_angle = angle(hand_landmarks.landmark[mp_hands.HandLandmark.MIDDLE_FINGER_TIP],
hand_landmarks.landmark[mp_hands.HandLandmark.MIDDLE_FINGER_PIP],
hand_landmarks.landmark[mp_hands.HandLandmark.MIDDLE_FINGER_MCP])
ring_angle = angle(hand_landmarks.landmark[mp_hands.HandLandmark.RING_FINGER_TIP],
hand_landmarks.landmark[mp_hands.HandLandmark.RING_FINGER_PIP],
hand_landmarks.landmark[mp_hands.HandLandmark.RING_FINGER_MCP])
pinky_angle = angle(hand_landmarks.landmark[mp_hands.HandLandmark.PINKY_TIP],
hand_landmarks.landmark[mp_hands.HandLandmark.PINKY_PIP],
hand_landmarks.landmark[mp_hands.HandLandmark.PINKY_MCP])
# 判断手势类型
if thumb_angle < 90 and index_angle < 90 and middle_angle < 90 and ring_angle < 90 and pinky_angle < 90:
gesture = GESTURES[0]
elif thumb_angle > 90 and index_angle > 90 and middle_angle > 90 and ring_angle > 90 and pinky_angle > 90:
gesture = GESTURES[1]
elif thumb_angle < 90 and index_angle < 90 and middle_angle < 90 and ring_angle < 90 and pinky_angle > 90:
gesture = GESTURES[2]
elif thumb_angle > 90 and index_angle < 90 and middle_angle < 90 and ring_angle > 90 and pinky_angle > 90:
gesture = GESTURES[3]
elif thumb_angle > 90 and index_angle > 90 and middle_angle < 90 and ring_angle < 90 and pinky_angle < 90:
gesture = GESTURES[4]
else:
gesture = 'Unknown'
# 在图像上显示手势类型
cv2.putText(image, gesture, (50, 50), cv2.FONT_HERSHEY_SIMPLEX, 1, (0, 255, 0), 2)
# 显示图像
cv2.imshow('Hand Gesture Recognition', image)
if cv2.waitKey(5) & 0xFF == 27:
break
# 释放资源
hands.close()
cap.release()
cv2.destroyAllWindows()
```
相关推荐
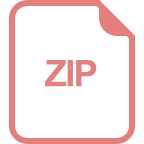
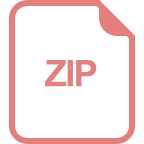
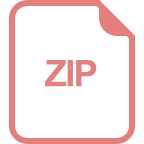






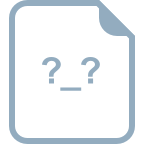
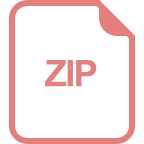
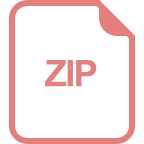
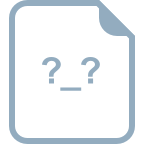
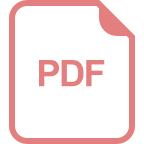
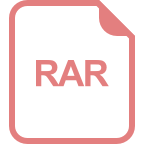
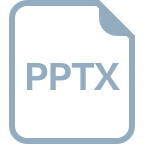