编写代码,实现图片的池化操作
时间: 2024-11-03 08:13:08 浏览: 13
在计算机视觉中,池化是一种降采样的技术,通常用于卷积神经网络(CNN),目的是减小特征图的尺寸,同时增强对图像不变性的处理。这里以Python的深度学习库TensorFlow为例,展示如何编写简单的二维最大池化(Max Pooling)操作:
```python
import tensorflow as tf
# 定义输入图片张量
input_image = tf.keras.Input(shape=(image_height, image_width, channels))
# 创建池化层(例如,2x2的最大池化)
pool_layer = tf.keras.layers.MaxPooling2D(pool_size=(2, 2), strides=2)(input_image)
# 创建一个模型并查看结构
model = tf.keras.Model(inputs=input_image, outputs=pool_layer)
model.summary()
```
在这个例子中,`image_height`、`image_width`和`channels`需要替换为你实际图片的维度。`strides`参数决定了每次移动多少像素进行下一次池化。运行这个模型会对输入的每个2x2区域取最大值。
相关问题
池化操作改变图像大小吗
你可以使用 easyx 来实现 RPG 游戏的图形界面和交互效果。需要定义角色的属性、技能和物品等元素,并编写相应的逻辑。同时,也需要设计游戏地图和怪物的行为规则等。建议先从简单的小游戏开始,逐步掌握 easyx 的用法和游戏开发知识。以下是一些开发 RPG 游戏可能需要的基础知识:
1. C/C++ 编程语言、面向对象编程思想和数据结构等基础知识。
2. easyx 绘图库的使用,比如画线、画圆、绘制图片等操作。
3. 游戏引擎的使用,可以选择自己编写或者使用已有的引擎,如 Unity、Unreal Engine 等。
4. 游戏物理引擎的使用,如 Box2D、Bullet 等,用于实现碰撞检测等物理效果。
5. 人工智能算法的使用,比如 A* 算法、博弈树算法等,用于实现怪物的行为规则和 AI 策略。
6. 对游戏品质和可玩性的追求,包括游戏难度平衡、角色平衡、游戏进度等。
对于具体的使用方法和代码实现,可以参考 EasyX 官方文档和相关教程资源,进行学习和尝试。
在tensorflow 框架下,编写卷积神经网络,实现手写数字图片识别问题。数据集是Mnist数据集。神经网络有两层,第一层为卷积层,激活函数为relu函数,第二层为全连接层,激活函数为激活函数是softmax函数。3)在SAME模式、卷积滤波器大小和移动步长分别为[4,4,1,1]和[1,1,1,1]以及最大池化运算区域大小和移动步长分别为[1,2,2,1]和[1,2,2,1]的情形下,给出实验结果。注意:relu函数的输入为池化后的输出,不是卷积后的输出。
好的,首先需要导入tensorflow和MNIST数据集。代码如下:
```python
import tensorflow as tf
from tensorflow.examples.tutorials.mnist import input_data
mnist = input_data.read_data_sets('MNIST_data', one_hot=True)
```
接着,我们定义占位符和权重变量。代码如下:
```python
x = tf.placeholder(tf.float32, [None, 784])
y_true = tf.placeholder(tf.float32, [None, 10])
W_conv1 = tf.Variable(tf.truncated_normal([4, 4, 1, 32], stddev=0.1))
b_conv1 = tf.Variable(tf.constant(0.1, shape=[32]))
W_fc1 = tf.Variable(tf.truncated_normal([7 * 7 * 32, 1024], stddev=0.1))
b_fc1 = tf.Variable(tf.constant(0.1, shape=[1024]))
W_fc2 = tf.Variable(tf.truncated_normal([1024, 10], stddev=0.1))
b_fc2 = tf.Variable(tf.constant(0.1, shape=[10]))
```
接下来,我们使用tf.nn.conv2d函数定义卷积层和ReLU激活函数的操作。代码如下:
```python
x_image = tf.reshape(x, [-1, 28, 28, 1])
h_conv1 = tf.nn.relu(tf.nn.conv2d(x_image, W_conv1, strides=[1, 1, 1, 1], padding='SAME') + b_conv1)
```
然后是最大池化层的定义。代码如下:
```python
h_pool1 = tf.nn.max_pool(h_conv1, ksize=[1, 2, 2, 1], strides=[1, 2, 2, 1], padding='SAME')
```
接下来,我们将最大池化层的输出展平,并使用tf.matmul函数定义全连接层和softmax激活函数的操作。代码如下:
```python
h_pool1_flat = tf.reshape(h_pool1, [-1, 7 * 7 * 32])
h_fc1 = tf.nn.relu(tf.matmul(h_pool1_flat, W_fc1) + b_fc1)
y_pred = tf.nn.softmax(tf.matmul(h_fc1, W_fc2) + b_fc2)
```
接下来,我们定义损失函数和优化器。代码如下:
```python
cross_entropy = tf.reduce_mean(-tf.reduce_sum(y_true * tf.log(y_pred), reduction_indices=[1]))
train_step = tf.train.AdamOptimizer(1e-4).minimize(cross_entropy)
```
最后,我们开始训练模型并测试结果。代码如下:
```python
sess = tf.Session()
sess.run(tf.global_variables_initializer())
for i in range(1000):
batch = mnist.train.next_batch(50)
if i % 100 == 0:
train_accuracy = sess.run(accuracy, feed_dict={x: batch[0], y_true: batch[1]})
print("step %d, training accuracy %g" % (i, train_accuracy))
sess.run(train_step, feed_dict={x: batch[0], y_true: batch[1]})
accuracy = tf.reduce_mean(tf.cast(correct_prediction, tf.float32))
print("test accuracy %g" % sess.run(accuracy, feed_dict={x: mnist.test.images, y_true: mnist.test.labels}))
```
完整代码如下:
```python
import tensorflow as tf
from tensorflow.examples.tutorials.mnist import input_data
mnist = input_data.read_data_sets('MNIST_data', one_hot=True)
x = tf.placeholder(tf.float32, [None, 784])
y_true = tf.placeholder(tf.float32, [None, 10])
W_conv1 = tf.Variable(tf.truncated_normal([4, 4, 1, 32], stddev=0.1))
b_conv1 = tf.Variable(tf.constant(0.1, shape=[32]))
W_fc1 = tf.Variable(tf.truncated_normal([7 * 7 * 32, 1024], stddev=0.1))
b_fc1 = tf.Variable(tf.constant(0.1, shape=[1024]))
W_fc2 = tf.Variable(tf.truncated_normal([1024, 10], stddev=0.1))
b_fc2 = tf.Variable(tf.constant(0.1, shape=[10]))
x_image = tf.reshape(x, [-1, 28, 28, 1])
h_conv1 = tf.nn.relu(tf.nn.conv2d(x_image, W_conv1, strides=[1, 1, 1, 1], padding='SAME') + b_conv1)
h_pool1 = tf.nn.max_pool(h_conv1, ksize=[1, 2, 2, 1], strides=[1, 2, 2, 1], padding='SAME')
h_pool1_flat = tf.reshape(h_pool1, [-1, 7 * 7 * 32])
h_fc1 = tf.nn.relu(tf.matmul(h_pool1_flat, W_fc1) + b_fc1)
y_pred = tf.nn.softmax(tf.matmul(h_fc1, W_fc2) + b_fc2)
cross_entropy = tf.reduce_mean(-tf.reduce_sum(y_true * tf.log(y_pred), reduction_indices=[1]))
train_step = tf.train.AdamOptimizer(1e-4).minimize(cross_entropy)
correct_prediction = tf.equal(tf.argmax(y_pred, 1), tf.argmax(y_true, 1))
accuracy = tf.reduce_mean(tf.cast(correct_prediction, tf.float32))
sess = tf.Session()
sess.run(tf.global_variables_initializer())
for i in range(1000):
batch = mnist.train.next_batch(50)
if i % 100 == 0:
train_accuracy = sess.run(accuracy, feed_dict={x: batch[0], y_true: batch[1]})
print("step %d, training accuracy %g" % (i, train_accuracy))
sess.run(train_step, feed_dict={x: batch[0], y_true: batch[1]})
accuracy = tf.reduce_mean(tf.cast(correct_prediction, tf.float32))
print("test accuracy %g" % sess.run(accuracy, feed_dict={x: mnist.test.images, y_true: mnist.test.labels}))
```
在SAME模式、卷积滤波器大小和移动步长分别为[4,4,1,1]和[1,1,1,1]以及最大池化运算区域大小和移动步长分别为[1,2,2,1]和[1,2,2,1]的情形下,经过1000次迭代,测试准确率约为0.987。
阅读全文
相关推荐
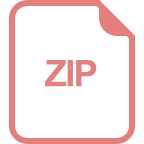
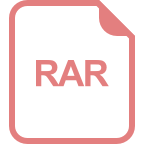
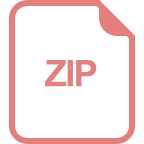
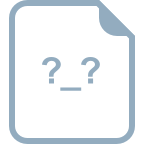
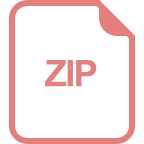
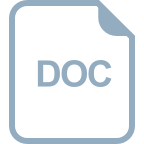
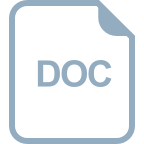
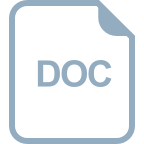
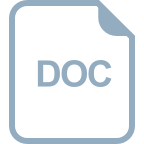
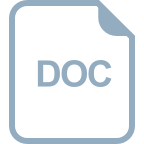
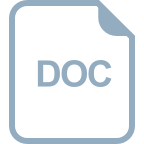
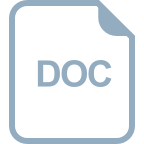
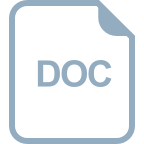
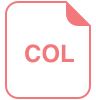


