print(f'test error: \n accuracy:{(100*correct):>0.1f}%, ave loss: {test_loss:>8f}')
时间: 2024-04-19 13:27:48 浏览: 56
这是一个使用 f-string 格式化输出的例子。在这个例子中,我们使用了两个变量 correct 和 test_loss。correct 表示正确的数量,test_loss 表示测试损失。这两个变量被插入到字符串中的占位符中。
在占位符中,我们使用了 :>0.1f 和 :>8f。这些是格式化选项,用于指定输出的格式。其中,:>0.1f 表示右对齐,至少占用 0 个字符,并保留一位小数。而 :>8f 表示右对齐,至少占用 8 个字符,并保留八位小数。
最终的输出结果将是类似于下面的形式:
test error:
accuracy: 99.9%, ave loss: 0.12345678
相关问题
为以下代码添加注释def test(dataloader, model, loss_fn): size = len(dataloader.dataset) num_batches = len(dataloader) model.eval() test_loss, correct = 0, 0 with torch.no_grad(): for X, y in dataloader: X, y = X.to(device), y.to(device) pred = model(X) test_loss += loss_fn(pred, y).item() correct += (pred.argmax(1) == y).type(torch.float).sum().item() test_loss /= num_batches correct /= size print(f"Test Error: \n Accuracy: {(100*correct):>0.1f}%, Avg loss: {test_loss:>8f} \n")
# 定义一个测试函数,用于测试模型
def test(dataloader, model, loss_fn):
# 获取数据集大小和批次数
size = len(dataloader.dataset)
num_batches = len(dataloader)
# 将模型设为评估模式
model.eval()
# 初始化测试损失和正确率
test_loss, correct = 0, 0
# 关闭梯度计算
with torch.no_grad():
# 遍历数据集
for X, y in dataloader:
# 将数据和标签移动到设备上
X, y = X.to(device), y.to(device)
# 前向传播计算预测值
pred = model(X)
# 计算损失
test_loss += loss_fn(pred, y).item()
# 统计正确率
correct += (pred.argmax(1) == y).type(torch.float).sum().item()
# 计算平均测试损失和正确率
test_loss /= num_batches
correct /= size
# 输出测试结果
print(f"Test Error: \n Accuracy: {(100*correct):>0.1f}%, Avg loss: {test_loss:>8f} \n")
def val(dataloader, model, loss_fn): size = len(dataloader.dataset) num_batches = len(dataloader) model.eval() val_loss, correct = 0, 0 with torch.no_grad(): for X, y in dataloader: X, y = X.to(device), y.to(device) pred = model(X) val_loss += loss_fn(pred, y).item() correct += (pred.argmax(1) == y).type(torch.float).sum().item() val_loss /= num_batches correct /= size print(f"val Error: \n Accuracy: {(100*correct):>0.1f}%, Avg loss: {val_loss:>8f} \n
这段代码是用于在验证集上评估模型性能的函数。让我来解释一下它的功能:
- `dataloader`:数据加载器,用于迭代验证集数据。
- `model`:模型,即你构建的卷积神经网络模型。
- `loss_fn`:损失函数,用于计算预测值与真实值之间的误差。
以下是函数的主要步骤:
1. 获取验证集的大小和批次数量,并将模型设置为评估模式。
2. 使用`torch.no_grad()`上下文管理器,禁用梯度计算,因为在验证过程中不需要更新模型参数。
3. 迭代数据加载器中的每个批次,其中`X`是输入图像,`y`是对应的标签。
4. 将数据和标签移动到设备(如GPU)上进行计算。
5. 计算模型对输入图像的预测,并使用损失函数计算预测误差。
6. 累积验证集损失和正确预测的数量,以便后续计算平均损失和准确率。
7. 计算平均验证集损失和准确率,并打印出来。
这个函数用于在验证集上评估模型的性能,并打印出验证准确率和平均损失。与训练函数类似,你可以根据需要进行调整和修改。
阅读全文
相关推荐
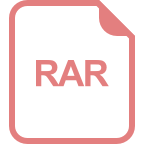
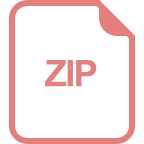
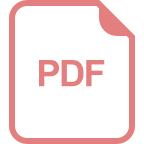





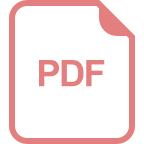
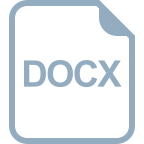
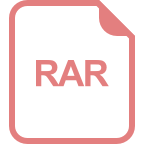
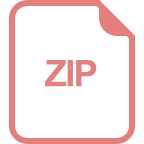



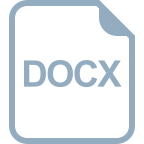