VS 部署openvino
时间: 2024-10-12 13:02:44 浏览: 29
在Visual Studio (VS) 中部署OpenVINO(Intel OpenVINO Toolkit),你需要完成以下几个步骤:
1. 安装 prerequisites:确保已经安装了Visual Studio版本(推荐使用最新版支持C++的版本),以及 Intel C++ Compiler 和 Visual C++ Redistributable。
2. 下载OpenVINO:从Intel官网上下载适合你环境的OpenVINO Toolkit,包含模型优化工具、推理引擎等组件。
3. 设置环境变量:配置环境变量,包括INTEL_CVSDK_DIR和INTEL_OPENVINO_DIR,以便VS能找到相关的库和头文件。
4. 添加项目依赖:在VS中创建新的C++项目,然后添加对OpenVINO库的引用。这通常涉及到添加头文件路径到项目属性,并链接相应的库。
5. 编写代码:使用OpenVINO提供的API(如InferenceEngine API)编写推理代码,处理图像数据并执行模型预测。
6. 构建和调试:在VS中构建项目并进行调试,确保程序能正常运行并且与OpenVINO集成无误。
7. 发布和打包:如果需要,你可以将编译后的应用程序打包成可执行文件或DLL,以便于部署。
相关问题
openvino部署yolo
OpenVINO是一个深度学习推理框架,可用于将训练好的神经网络模型部署到各种硬件平台上进行推理。下面是在OpenVINO上部署Yolo的一些步骤:
1. 下载和安装OpenVINO Toolkit。在OpenVINO官网下载并安装适合您操作系统的版本。
2. 下载Yolo的权重文件和配置文件。在Yolo官网上下载权重文件和配置文件。
3. 将Yolo模型转换为OpenVINO格式。使用OpenVINO提供的Model Optimizer工具将Yolo模型转换为OpenVINO格式。您需要使用以下命令:
```
python mo_tf.py --input_model yolov3.weights --tensorflow_use_custom_operations_config extensions/front/tf/yolo_v3.json --input_shape [1,416,416,3] --data_type FP32 --output_dir yolov3_openvino --model_name yolov3
```
其中,yolov3.weights是您下载的权重文件,yolo_v3.json是一个自定义操作文件,用于告诉Model Optimizer如何处理Yolo模型。--input_shape参数指定输入张量的形状,--data_type参数指定数据类型,--output_dir参数指定输出文件夹,--model_name参数指定模型名称。
4. 运行OpenVINO推理引擎。使用OpenVINO Inference Engine API加载和推理转换后的模型。您需要编写一个Python脚本来完成这一步骤。以下是一个简单的示例:
```
import cv2
import numpy as np
from openvino.inference_engine import IECore
# Load the OpenVINO model
model_xml = 'yolov3_openvino/yolov3.xml'
model_bin = 'yolov3_openvino/yolov3.bin'
ie = IECore()
net = ie.read_network(model=model_xml, weights=model_bin)
# Load the input image
img = cv2.imread('input.jpg')
# Preprocess the input image
input_blob = next(iter(net.inputs))
n, c, h, w = net.inputs[input_blob].shape
img = cv2.resize(img, (w, h))
img = img.transpose((2, 0, 1))
img = img.reshape((n, c, h, w))
# Run inference
exec_net = ie.load_network(network=net, device_name='CPU')
output = exec_net.infer(inputs={input_blob: img})
# Process the output
output_blob = next(iter(net.outputs))
output = output[output_blob][0]
boxes = output[:, 0:4]
confs = output[:, 4]
class_ids = output[:, 5:].argmax(axis=-1)
# Draw the predicted boxes on the input image
for i in range(len(boxes)):
if confs[i] > 0.5:
x, y, w, h = boxes[i]
cv2.rectangle(img, (int(x), int(y)), (int(x+w), int(y+h)), (0, 255, 0), 2)
# Save the output image
cv2.imwrite('output.jpg', img.transpose((1, 2, 0)))
```
这个脚本加载了转换后的Yolo模型,读取了输入图像,对图像进行预处理,运行了推理,处理了输出并将结果绘制在输入图像上,最后保存了输出图像。
这些步骤仅仅是一个简单的例子,如果您需要更复杂的操作,您需要仔细阅读OpenVINO文档,并参考OpenVINO的示例代码。
openvino部署yolov5
部署 YOLOv5 到 OpenVINO 可以通过以下步骤完成:
1. 下载 YOLOv5 模型:从 https://github.com/ultralytics/yolov5/releases 下载预训练权重文件,例如 yolov5s.pt。
2. 安装 OpenVINO:请确保已经安装了 OpenVINO 工具包,并设置了正确的环境变量。
3. 转换模型:使用 OpenVINO 的 Model Optimizer 工具将 YOLOv5 模型转换为 OpenVINO 可以使用的 IR 格式。在转换模型之前,您需要准备一个 .yaml 配置文件,该文件包含有关模型的信息。例如,以下是一个针对 yolov5s.pt 的配置文件示例:
```
model:
name: yolov5s
input_shape: [1,3,640,640]
output_names: ['output']
input_names: ['input']
precision: FP32
mean_values: [0,0,0]
scale_values: [1,1,1]
```
这个配置文件中指定了模型的名称、输入形状、输出名称、输入名称、精度、均值和标准差。
然后,您可以运行以下命令将模型转换为 IR 格式:
```
python <path_to_openvino>/deployment_tools/model_optimizer/mo.py --input_model <path_to_yolov5s.pt> --transformations_config <path_to_yolo_v5s.yaml>
```
这会生成一个 IR 文件,例如 yolov5s.xml 和 yolov5s.bin。
4. 运行模型:使用 OpenVINO 的 Inference Engine 运行模型。以下是一个 Python 脚本示例,演示如何加载并运行 IR 文件:
```python
import cv2
import numpy as np
from openvino.inference_engine import IECore
# 加载 IR 文件
model_xml = 'yolov5s.xml'
model_bin = 'yolov5s.bin'
ie = IECore()
net = ie.read_network(model=model_xml, weights=model_bin)
# 获取输入和输出信息
input_blob = next(iter(net.input_info))
output_blob = next(iter(net.outputs))
# 加载图像
image = cv2.imread('image.jpg')
# 预处理输入图像
input_shape = net.input_info[input_blob].input_data.shape
image = cv2.resize(image, (input_shape[3], input_shape[2]))
image = image.transpose((2, 0, 1))
image = image.reshape((1,) + image.shape)
# 运行推理
exec_net = ie.load_network(network=net, device_name='CPU')
output = exec_net.infer(inputs={input_blob: image})
# 后处理输出
output = output[output_blob]
output = np.squeeze(output)
detections = []
for det in output:
if det[4] > 0.5:
detections.append(det)
# 打印检测结果
print(detections)
```
这个脚本将图像输入模型,运行推理,并输出检测结果。
请注意,这只是一个示例脚本,您需要根据您的实际应用程序对其进行修改。例如,您可能需要更改输入和输出名称、设备名称和推理参数。
阅读全文
相关推荐
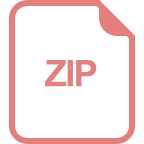
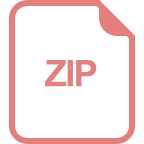
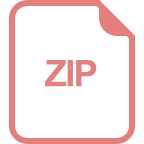
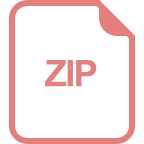
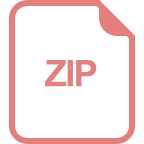
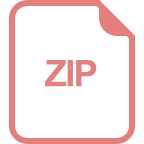
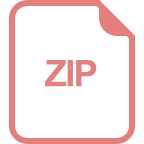
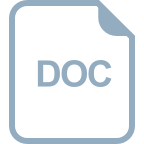





