基于tensorflow的手写数字识别
时间: 2023-04-21 20:06:05 浏览: 88
基于tensorflow的手写数字识别是一种人工智能技术,可以通过训练模型来识别手写数字。使用tensorflow框架可以方便地搭建神经网络模型,并进行训练和测试。该技术在数字识别、自然语言处理等领域有广泛应用。
相关问题
基于tensorflow手写数字识别构建三层神经网络的代码
以下是基于TensorFlow实现手写数字识别的三层神经网络代码,供您参考:
```python
import tensorflow as tf
from tensorflow.examples.tutorials.mnist import input_data
# 读取MNIST数据集
mnist = input_data.read_data_sets("MNIST_data/", one_hot=True)
# 定义网络超参数
learning_rate = 0.01
training_epochs = 100
batch_size = 100
display_step = 1
# 定义网络参数
n_hidden_1 = 256
n_hidden_2 = 256
n_input = 784
n_classes = 10
# 定义占位符
x = tf.placeholder("float", [None, n_input])
y = tf.placeholder("float", [None, n_classes])
# 定义权重和偏置变量
weights = {
'h1': tf.Variable(tf.random_normal([n_input, n_hidden_1])),
'h2': tf.Variable(tf.random_normal([n_hidden_1, n_hidden_2])),
'out': tf.Variable(tf.random_normal([n_hidden_2, n_classes]))
}
biases = {
'b1': tf.Variable(tf.random_normal([n_hidden_1])),
'b2': tf.Variable(tf.random_normal([n_hidden_2])),
'out': tf.Variable(tf.random_normal([n_classes]))
}
# 定义三层神经网络的模型
def multilayer_perceptron(x):
layer_1 = tf.add(tf.matmul(x, weights['h1']), biases['b1'])
layer_1 = tf.nn.relu(layer_1)
layer_2 = tf.add(tf.matmul(layer_1, weights['h2']), biases['b2'])
layer_2 = tf.nn.relu(layer_2)
out_layer = tf.matmul(layer_2, weights['out']) + biases['out']
return out_layer
# 定义损失函数和优化器
pred = multilayer_perceptron(x)
cost = tf.reduce_mean(tf.nn.softmax_cross_entropy_with_logits(logits=pred, labels=y))
optimizer = tf.train.AdamOptimizer(learning_rate=learning_rate).minimize(cost)
# 初始化变量
init = tf.global_variables_initializer()
# 开始训练模型
with tf.Session() as sess:
sess.run(init)
for epoch in range(training_epochs):
avg_cost = 0.
total_batch = int(mnist.train.num_examples/batch_size)
for i in range(total_batch):
batch_x, batch_y = mnist.train.next_batch(batch_size)
_, c = sess.run([optimizer, cost], feed_dict={x: batch_x, y: batch_y})
avg_cost += c / total_batch
if epoch % display_step == 0:
print("Epoch:", '%04d' % (epoch+1), "cost=", "{:.9f}".format(avg_cost))
print("Optimization Finished!")
# 测试模型
correct_prediction = tf.equal(tf.argmax(pred, 1), tf.argmax(y, 1))
accuracy = tf.reduce_mean(tf.cast(correct_prediction, "float"))
print("Accuracy:", accuracy.eval({x: mnist.test.images, y: mnist.test.labels}))
```
代码中首先读取MNIST数据集,并定义网络的超参数和参数,包括输入层784个节点,两个隐藏层分别有256个节点,输出层10个节点等。接着定义了占位符、权重和偏置变量,以及三层神经网络的模型和损失函数、优化器。然后初始化变量,并开始训练模型。最后测试模型,并计算准确率。
基于 TensorFlow的手写体数字识别
TensorFlow是一个广泛用于机器学习和深度学习的开源框架,可以用它来实现手写体数字识别。该任务通常使用卷积神经网络(CNN)来实现,以下是一个基于TensorFlow的手写体数字识别的简单步骤:
1. 数据准备:获取手写数字图像数据集,如MNIST数据集。
2. 数据预处理:将图像数据转换为适合CNN的格式,如将二维图像转换为三维张量。
3. 模型构建:使用TensorFlow构建CNN模型,包括卷积层、池化层和全连接层。
4. 模型训练:使用训练数据集对CNN模型进行训练,包括前向传播、反向传播和参数更新。
5. 模型测试:使用测试数据集对训练好的CNN模型进行测试,并计算准确率等指标。
6. 模型优化:根据测试结果对模型进行优化,如增加/减少神经元数量、调整学习率等。
7. 模型应用:将训练好的模型应用到实际手写数字识别任务中,如通过输入手写数字图像,输出对应数字标签。
以上是基本的手写体数字识别步骤,当然还可以根据具体需求进行更加复杂的处理和优化。
相关推荐
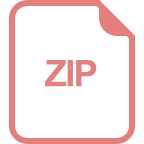
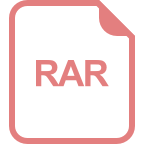
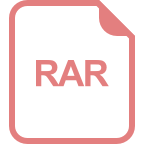
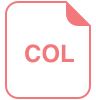
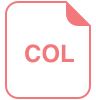
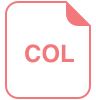

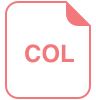







