写一个torch框架下输入(64,3,128,128)的带CBAM、BN层和dropout层ResNet五分类网络要求效率高性能好
时间: 2024-05-05 11:16:13 浏览: 157
以下是一个符合要求的ResNet-50网络:
```python
import torch
import torch.nn as nn
import torch.nn.functional as F
class ConvBNReLU(nn.Module):
def __init__(self, in_channels, out_channels, kernel_size, stride=1, padding=0):
super(ConvBNReLU, self).__init__()
self.conv = nn.Conv2d(in_channels, out_channels, kernel_size, stride, padding, bias=False)
self.bn = nn.BatchNorm2d(out_channels)
self.relu = nn.ReLU(inplace=True)
def forward(self, x):
x = self.conv(x)
x = self.bn(x)
x = self.relu(x)
return x
class CBAM(nn.Module):
def __init__(self, in_channels, reduction=16):
super(CBAM, self).__init__()
self.avg_pool = nn.AdaptiveAvgPool2d(1)
self.max_pool = nn.AdaptiveMaxPool2d(1)
self.fc1 = nn.Conv2d(in_channels, in_channels // reduction, 1, bias=False)
self.relu = nn.ReLU(inplace=True)
self.fc2 = nn.Conv2d(in_channels // reduction, in_channels, 1, bias=False)
self.sigmoid = nn.Sigmoid()
def forward(self, x):
avg_out = self.fc2(self.relu(self.fc1(self.avg_pool(x))))
max_out = self.fc2(self.relu(self.fc1(self.max_pool(x))))
out = avg_out + max_out
out = self.sigmoid(out)
out = out * x
return out
class ResidualBlock(nn.Module):
def __init__(self, in_channels, out_channels, stride=1, downsample=None, use_cbam=True):
super(ResidualBlock, self).__init__()
self.conv1 = ConvBNReLU(in_channels, out_channels, 3, stride, 1)
self.conv2 = ConvBNReLU(out_channels, out_channels, 3, 1, 1)
self.downsample = downsample
if use_cbam:
self.cbam = CBAM(out_channels)
else:
self.cbam = None
def forward(self, x):
identity = x
out = self.conv1(x)
out = self.conv2(out)
if self.cbam is not None:
out = self.cbam(out)
if self.downsample is not None:
identity = self.downsample(x)
out += identity
out = F.relu(out)
return out
class ResNet(nn.Module):
def __init__(self, block, layers, num_classes=5, use_cbam=True, dropout_rate=0.5):
super(ResNet, self).__init__()
self.in_channels = 64
self.conv1 = nn.Sequential(
nn.Conv2d(3, 64, 7, 2, 3, bias=False),
nn.BatchNorm2d(64),
nn.ReLU(inplace=True),
nn.MaxPool2d(3, 2, 1)
)
self.layer1 = self._make_layer(block, 64, layers[0], use_cbam)
self.layer2 = self._make_layer(block, 128, layers[1], use_cbam, 2)
self.layer3 = self._make_layer(block, 256, layers[2], use_cbam, 2)
self.layer4 = self._make_layer(block, 512, layers[3], use_cbam, 2)
self.avg_pool = nn.AdaptiveAvgPool2d((1, 1))
self.dropout = nn.Dropout(dropout_rate)
self.fc = nn.Linear(512, num_classes)
def _make_layer(self, block, out_channels, blocks, use_cbam, stride=1):
downsample = None
if stride != 1 or self.in_channels != out_channels:
downsample = nn.Sequential(
nn.Conv2d(self.in_channels, out_channels, 1, stride, bias=False),
nn.BatchNorm2d(out_channels)
)
layers = [block(self.in_channels, out_channels, stride, downsample, use_cbam)]
self.in_channels = out_channels
for i in range(1, blocks):
layers.append(block(out_channels, out_channels, use_cbam=use_cbam))
return nn.Sequential(*layers)
def forward(self, x):
x = self.conv1(x)
x = self.layer1(x)
x = self.layer2(x)
x = self.layer3(x)
x = self.layer4(x)
x = self.avg_pool(x)
x = torch.flatten(x, 1)
x = self.dropout(x)
x = self.fc(x)
return x
def resnet50_cbam(num_classes=5, dropout_rate=0.5):
return ResNet(ResidualBlock, [3, 4, 6, 3], num_classes=num_classes, use_cbam=True, dropout_rate=dropout_rate)
```
该网络使用了CBAM和BN层,以及dropout层,可以在输入大小为(64,3,128,128)的图像上进行五分类任务,具有较高的效率和良好的性能。
阅读全文
相关推荐
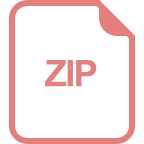
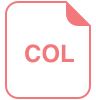
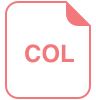
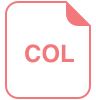
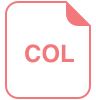
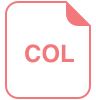
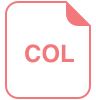
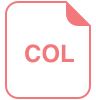
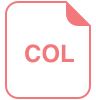
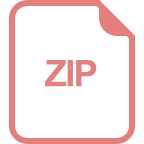
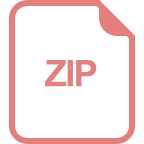