k-svd算法代码实现
时间: 2023-11-17 14:07:13 浏览: 108
以下是 K-SVD 算法的 MATLAB 代码实现:
```matlab
function [D,X] = ksvd(Y,k,T)
% Y: 数据矩阵, d x n
% k: 字典大小
% T: 迭代次数
[d,n] = size(Y);
% 初始字典
D = normc(randn(d,k));
for t = 1:T
% 稀疏编码
X = omp(D,Y,[],'maxatoms',ceil(d/4));
% 更新字典
for j = 1:k
% 找到使用第 j 列的数据
idx = find(X(j,:));
if isempty(idx)
continue;
end
% 减去使用第 j 列的数据对应的列
E = Y(:,idx) - D*X(:,idx) + D(:,j)*X(j,idx);
% 奇异值分解
[U,S,V] = svd(E,'econ');
% 更新字典和稀疏表示
D(:,j) = U(:,1);
X(j,idx) = S(1,1)*V(:,1)';
end
end
end
```
这里调用了 MATLAB 自带的 `omp` 函数进行稀疏编码,使用了 `svd` 函数进行奇异值分解。需要注意的是,这个实现并不是最优化的,仅供参考。
相关问题
k-svd算法matlab代码
以下是一个简单的 MATLAB 实现 K-SVD 算法的代码:
```matlab
function [D,X] = ksvd(Y,D,X,iterations)
% Y: 数据矩阵, d x n
% D: 初始字典, d x k
% X: 初始稀疏表示, k x n
% iterations: 迭代次数
[d,n] = size(Y);
k = size(D,2);
for iter = 1:iterations
% 更新稀疏表示
for i = 1:n
Xi = X(:,i);
Di = D;
Di(:,Xi~=0) = 0;
residual = Y(:,i) - D*Xi;
active_idx = find(Xi~=0);
if ~isempty(active_idx)
residual = residual + D(:,active_idx)*(Xi(active_idx)*active_idx');
end
[Ci,~,~] = svds(residual,1);
Xi = zeros(k,1);
Xi(active_idx) = X(active_idx,i);
Xi(Xi~=0) = Ci;
X(:,i) = Xi;
end
% 更新字典
for j = 1:k
active_idx = find(X(j,:)~=0);
if ~isempty(active_idx)
E = Y(:,active_idx) - D*X(:,active_idx) + D(:,j)*X(j,active_idx);
[U,S,V] = svds(E,1);
D(:,j) = U;
X(j,active_idx) = S*V';
end
end
end
end
```
这里的 `svds` 函数用来计算矩阵的奇异值分解。需要注意的是,这个实现并不是最优化的,仅供参考。
k-svd字典学习代码
以下是一个简单的 Python 实现 k-svd 字典学习算法的代码:
```python
import numpy as np
from scipy.linalg import svd
def ksvd(Y, K, T):
"""
k-svd 字典学习算法
参数:
Y: (n, m) ndarray,其中 n 是样本的维度,m 是样本的数量
K: 字典的大小
T: 迭代次数
返回值:
D: (n, K) ndarray,学习到的字典
X: (K, m) ndarray,表示每个样本在字典上的表示
"""
# 随机初始化字典
n, m = Y.shape
D = np.random.randn(n, K)
D /= np.linalg.norm(D, axis=0)
for t in range(T):
# 更新 X
X = np.dot(np.linalg.pinv(D), Y)
# 更新 D
for k in range(K):
# 找到使用第 k 列的样本
I = np.nonzero(X[k, :])[0]
if len(I) == 0:
continue
# 计算误差矩阵和奇异值分解
E = Y[:, I] - np.dot(D, X[:, I])
U, S, V = svd(E)
# 更新字典的第 k 列和 X 的第 k 行
D[:, k] = U[:, 0]
X[k, I] = S[0] * V[0, :]
# 记录更新后的误差
Y[:, I] = np.dot(D, X[:, I])
return D, X
```
使用方法:
```python
# 生成一些随机样本
n, m = 50, 100
Y = np.random.randn(n, m)
# 运行 k-svd 算法
K = 10
T = 50
D, X = ksvd(Y, K, T)
# 显示学习到的字典
import matplotlib.pyplot as plt
fig, axs = plt.subplots(1, K, figsize=(10, 2))
for k in range(K):
axs[k].imshow(D[:, k].reshape(5, 10), cmap='gray')
axs[k].axis('off')
plt.show()
```
相关推荐
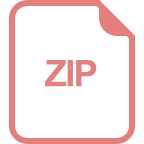












