tensorflow >= 1.11.0 # CPU Version of TensorFlow. # tensorflow-gpu >= 1.11.0 # GPU version of TensorFlow.
时间: 2024-09-29 13:02:25 浏览: 71
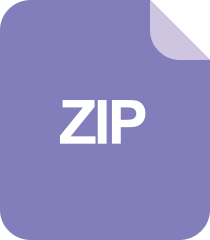
tensorflow-1.11.0-cp36-cp36m-win_amd64.zip
TensorFlow是一个开源的人工智能计算框架,最初由Google开发,用于构建和部署机器学习模型。从版本1.11.0开始,它支持了两个主要的变体:CPU版本和GPU版本。
CPU版本的TensorFlow(tensorflow>=1.11.0)针对没有GPU设备或希望在CPU上运行模型的情况设计,它的核心功能包括数据流图、张量操作和各种预训练模型的API,适合于在普通计算机上进行大规模数据处理和模型训练。
GPU版本的TensorFlow(tensorflow-gpu>=1.11.0),则是专为利用图形处理器的强大并行计算能力优化的。相比CPU,GPU拥有更多的处理单元,可以显著加速深度学习模型的训练速度,特别是对于需要大量矩阵运算的任务,如卷积神经网络(CNN)和循环神经网络(RNN)。
要安装特定版本的TensorFlow,你可以使用包管理工具(如pip)在Python环境中进行安装,例如:
```bash
pip install tensorflow==1.11.0 # 如果是CPU版本
pip install tensorflow-gpu==1.11.0 # 如果是GPU版本,并且已配置好CUDA和cuDNN
```
阅读全文
相关推荐
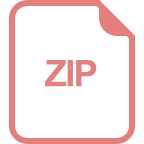

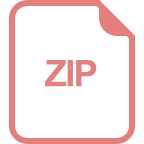
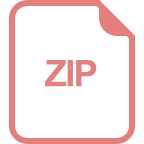
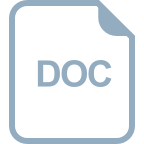
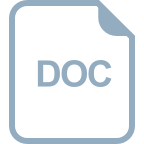
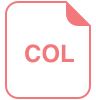


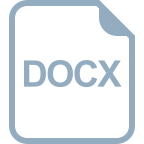
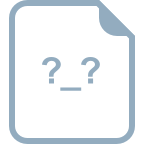
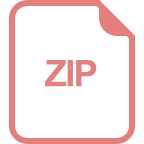