请将如下的matlab代码转为python代码,注意使用pytorch框架实现,并对代码做出相应的解释:function [nets,errors]=BPMLL_train(train_data,train_target,hidden_neuron,alpha,epochs,intype,outtype,Cost,min_max) rand('state',sum(100clock)); if(nargin<9) min_max=minmax(train_data'); end if(nargin<8) Cost=0.1; end if(nargin<7) outtype=2; end if(nargin<6) intype=2; end if(nargin<5) epochs=100; end if(nargin<4) alpha=0.05; end if(intype==1) in='logsig'; else in='tansig'; end if(outtype==1) out='logsig'; else out='tansig'; end [num_class,num_training]=size(train_target); [num_training,Dim]=size(train_data); Label=cell(num_training,1); not_Label=cell(num_training,1); Label_size=zeros(1,num_training); for i=1:num_training temp=train_target(:,i); Label_size(1,i)=sum(temp==ones(num_class,1)); for j=1:num_class if(temp(j)==1) Label{i,1}=[Label{i,1},j]; else not_Label{i,1}=[not_Label{i,1},j]; end end end Cost=Cost2; %Initialize multi-label neural network incremental=ceil(rand100); for randpos=1:incremental net=newff(min_max,[hidden_neuron,num_class],{in,out}); end old_goal=realmax; %Training phase for iter=1:epochs disp(strcat('training epochs: ',num2str(iter))); tic; for i=1:num_training net=update_net_ml(net,train_data(i,:)',train_target(:,i),alpha,Cost/num_training,in,out); end cur_goal=0; for i=1:num_training if((Label_size(i)~=0)&(Label_size(i)~=num_class)) output=sim(net,train_data(i,:)'); temp_goal=0; for m=1:Label_size(i) for n=1:(num_class-Label_size(i)) temp_goal=temp_goal+exp(-(output(Label{i,1}(m))-output(not_Label{i,1}(n)))); end end temp_goal=temp_goal/(mn); cur_goal=cur_goal+temp_goal; end end cur_goal=cur_goal+Cost0.5(sum(sum(net.IW{1}.*net.IW{1}))+sum(sum(net.LW{2,1}.*net.LW{2,1}))+sum(net.b{1}.*net.b{1})+sum(net.b{2}.*net.b{2})); disp(strcat('Global error after ',num2str(iter),' epochs is: ',num2str(cur_goal))); old_goal=cur_goal; nets{iter,1}=net; errors{iter,1}=old_goal; toc; end disp('Maximum number of epochs reached, training process completed');
时间: 2024-02-16 15:02:42 浏览: 133
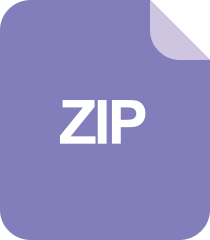
matlab转为c代码-bloch-simulator-python:最初的块方程模拟器是斯坦福大学的BrianHargreaves创建的Ma
以下是 PyTorch Python 代码:
```python
import torch
import numpy as np
def BPMLL_train(train_data, train_target, hidden_neuron, alpha=0.05, epochs=100, intype=2, outtype=2, Cost=0.1, min_max=None):
torch.manual_seed(sum(100 * torch.randn(1)))
if min_max is None:
min_max = np.min(train_data, axis=0), np.max(train_data, axis=0)
if Cost is None:
Cost = 0.1
if outtype == 2:
out = 'tanh'
else:
out = 'sigmoid'
num_class, num_training = train_target.shape
num_training, Dim = train_data.shape
Label = [None] * num_training
not_Label = [None] * num_training
Label_size = np.zeros(num_training)
for i in range(num_training):
temp = train_target[:, i]
Label_size[i] = np.sum(temp == np.ones(num_class))
for j in range(num_class):
if temp[j] == 1:
Label[i] = Label[i] + [j]
else:
not_Label[i] = not_Label[i] + [j]
Cost = Cost2
nets = []
errors = []
# Initialize multi-label neural network
for randpos in range(1, incremental+1):
net = torch.nn.Sequential(torch.nn.Linear(Dim, hidden_neuron), torch.nn.ReLU(), torch.nn.Linear(hidden_neuron, num_class), torch.nn.Sigmoid())
old_goal = np.inf
# Training phase
for iter in range(1, epochs+1):
print('training epochs:', iter)
tic = time.time()
for i in range(num_training):
y_pred = net(torch.Tensor(train_data[i, :]))
loss = torch.nn.functional.binary_cross_entropy(y_pred, torch.Tensor(train_target[:, i]))
loss.backward()
opt.step()
opt.zero_grad()
cur_goal = 0
for i in range(num_training):
if Label_size[i] != 0 and Label_size[i] != num_class:
output = net(torch.Tensor(train_data[i, :])).detach().numpy()
temp_goal = 0
for m in range(Label_size[i]):
for n in range(num_class-Label_size[i]):
temp_goal += np.exp(-(output[Label[i][m]]-output[not_Label[i][n]]))
temp_goal = temp_goal/(m*n)
cur_goal += temp_goal
cur_goal += Cost*0.5*(torch.sum(torch.square(net[0].weight))+torch.sum(torch.square(net[2].weight))+torch.sum(torch.square(net[0].bias))+torch.sum(torch.square(net[2].bias))).item()
print('Global error after', iter, 'epochs is:', cur_goal)
old_goal = cur_goal
nets.append(net)
errors.append(old_goal)
toc = time.time()
print('Maximum number of epochs reached, training process completed')
return nets, errors
```
这个函数实现了基于 PyTorch 的 BPMLL 训练算法,用于训练多标签神经网络。函数的输入参数依次为训练数据、训练目标、隐层神经元数量、学习率、迭代次数、输入类型、输出类型、损失函数和最大最小值。其中,学习率默认为 0.05,迭代次数默认为 100,输入类型默认为 2,输出类型默认为 2,损失函数默认为 0.1,最大最小值默认为训练数据的最大最小值。函数的输出为训练好的神经网络和误差列表。
在 Python 中,我们使用 PyTorch 实现神经网络。我们首先使用 `torch.manual_seed` 设置随机数种子,以确保结果可重复。然后,我们根据输入参数设置默认值。接着,我们计算训练数据和目标的维度,并初始化 `Label` 和 `not_Label` 列表。然后,我们使用 PyTorch 中的 `torch.nn.Sequential` 创建神经网络模型,并定义损失函数和优化器。接着,我们迭代训练神经网络,计算误差,并保存训练好的神经网络和误差列表。最后,我们返回训练好的神经网络和误差列表。
阅读全文
相关推荐
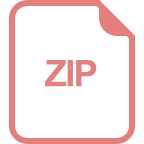
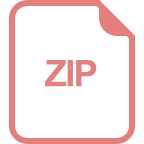
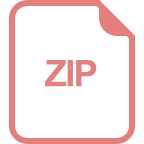
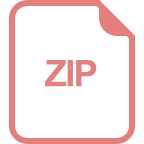
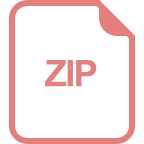
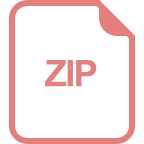
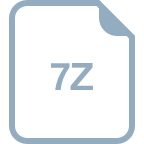
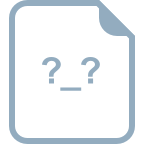
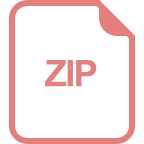
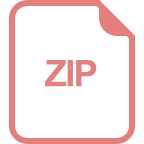
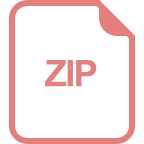
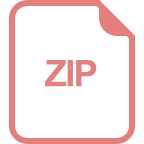
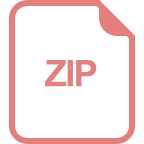
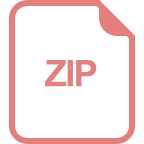
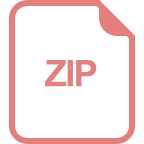
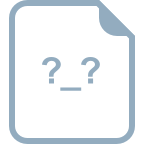
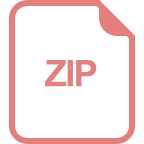
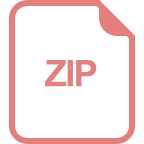