with torch.no_grad():
时间: 2024-06-08 18:08:16 浏览: 30
使用`with torch.no_grad():`的作用是停止自动反向计算梯度,并且表明当前计算不需要反向传播。在使用`with torch.no_grad():`语句块内的计算结果不会被记录在计算图中,因此不会进行梯度反传等操作。这样可以有效地减少内存的使用量,尤其是在模型运行时出现CUDA out of memory错误的情况下可以解决该问题。因此,可以将`with torch.no_grad():`用于不需要进行梯度计算的部分,提高代码的效率和性能。
相关问题
def test(): correct = 0 total = 0 with torch.no_grad: for data in test_loader: x, y =data y_pred = model(x) _, predicted = torch.max(y_pred, dim=1) total += y.size(0) correct += (predicted == y).sum().item() print('accuracy on test_data:%d %%' % (100 *correct/total))
这段代码存在一个语法错误,在第 4 行应该调用 `torch.no_grad()` 方法而不是 `torch.no_grad` 属性。`torch.no_grad()` 方法是一个上下文管理器,它使得在该上下文中计算的所有张量都不会被跟踪梯度,从而提高了计算效率。
正确的代码应该是:
```
def test():
correct = 0
total = 0
with torch.no_grad():
for data in test_loader:
x, y = data
y_pred = model(x)
_, predicted = torch.max(y_pred, dim=1)
total += y.size(0)
correct += (predicted == y).sum().item()
print('accuracy on test_data:%d %%' % (100 * correct/total))
```
注意,在 `with` 语句中调用 `torch.no_grad()` 方法之后,所有在该上下文中计算的张量都不会被跟踪梯度,这有助于提高计算效率。然而,如果需要计算梯度,则需要退出该上下文并调用 `backward()` 方法。
with torch.no_grad
`torch.no_grad()`是一个上下文管理器,用于在进行模型推理时禁用梯度计算。在这个上下文中,不会记录梯度信息,从而减少了内存消耗并提高了推理速度。这通常用于测试集上的模型推理,因为在测试集上不需要更新模型的参数。例如:
```
with torch.no_grad():
output = model(input)
```
在这段代码中,`input`是输入数据,`model`是神经网络模型,`output`是模型的输出结果。在`with torch.no_grad()`的上下文中,模型的参数不会被更新,并且梯度计算不会被记录,从而加速了模型的推理过程。
阅读全文
相关推荐
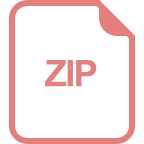
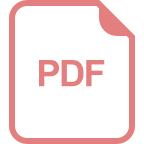
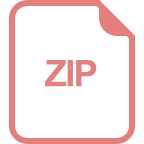
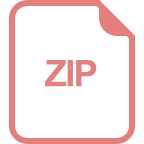
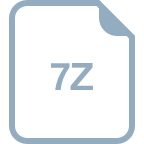
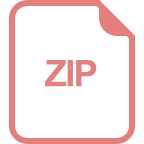
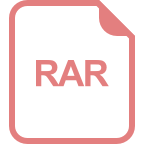
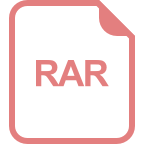
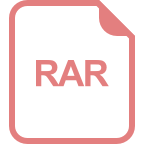
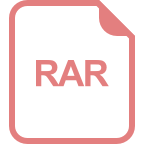