adabn算法pytorch实现
时间: 2023-07-07 07:16:00 浏览: 99
下面是一个简单的基于PyTorch实现AdaBoost算法的示例代码:
```
import torch
from torch.utils.data import DataLoader, Dataset
class AdaBoost:
def __init__(self, weak_classifier, n_estimators):
self.weak_classifier = weak_classifier
self.n_estimators = n_estimators
self.alpha = []
self.classifiers = []
def fit(self, X, y):
n_samples = len(X)
w = torch.ones(n_samples) / n_samples
for i in range(self.n_estimators):
h = self.weak_classifier()
h.fit(X, y, w)
y_pred = h.predict(X)
error = torch.dot(w, (y_pred != y).float())
alpha = 0.5 * torch.log((1 - error) / error)
w = w * torch.exp(-alpha * y * y_pred)
w = w / torch.sum(w)
self.alpha.append(alpha)
self.classifiers.append(h)
def predict(self, X):
y_pred = torch.zeros(len(X))
for alpha, h in zip(self.alpha, self.classifiers):
y_pred += alpha * h.predict(X)
return torch.sign(y_pred)
class DecisionStump:
def __init__(self):
self.polarity = 1
self.threshold = None
self.feature_index = None
def fit(self, X, y, w):
n_samples, n_features = X.shape
best_error = float('inf')
for feature_idx in range(n_features):
feature_values = X[:, feature_idx]
thresholds = torch.unique(feature_values)
for threshold in thresholds:
p = 1
y_pred = torch.ones(n_samples)
y_pred[feature_values < threshold] = -1
error = torch.dot(w, (y_pred != y).float())
if error > 0.5:
error = 1 - error
p = -1
if error < best_error:
self.polarity = p
self.threshold = threshold
self.feature_index = feature_idx
best_error = error
def predict(self, X):
n_samples = X.shape[0]
y_pred = torch.ones(n_samples)
feature_values = X[:, self.feature_index]
y_pred[self.polarity * feature_values < self.polarity * self.threshold] = -1
return y_pred
class ToyDataset(Dataset):
def __init__(self, X, y):
self.X = X
self.y = y
def __len__(self):
return len(self.X)
def __getitem__(self, idx):
return self.X[idx], self.y[idx]
X = torch.tensor([[1, 2], [2, 1], [2, 3], [4, 5], [5, 4], [5, 6], [7, 8], [8, 7], [8, 9], [10, 11], [11, 10], [11, 12], [13, 14], [14, 13], [14, 15], [16, 17], [17, 16], [17, 18]])
y = torch.tensor([-1, -1, -1, -1, -1, -1, 1, 1, 1, 1, 1, 1, 1, 1, 1, 1, 1, 1])
dataset = ToyDataset(X, y)
dataloader = DataLoader(dataset, batch_size=len(X), shuffle=True)
ada = AdaBoost(weak_classifier=DecisionStump, n_estimators=10)
for X_batch, y_batch in dataloader:
ada.fit(X_batch, y_batch)
print(ada.predict(X))
```
在这个示例中,我们首先定义了一个AdaBoost类,它包含了一些成员变量和方法:
- weak_classifier:弱分类器,这里我们使用了决策树桩(Decision Stump);
- n_estimators:基分类器的数量;
- alpha:每个基分类器的权重;
- classifiers:基分类器的列表;
- fit:训练模型的方法;
- predict:使用训练好的模型进行预测的方法。
接下来,我们定义了一个DecisionStump类作为弱分类器,它包含了以下成员变量和方法:
- polarity:分类器的方向;
- threshold:分类器的阈值;
- feature_index:分类器选择的特征索引;
- fit:训练模型的方法;
- predict:使用训练好的模型进行预测的方法。
最后,我们定义了一个ToyDataset类作为数据集,它包含了以下方法:
- \_\_len\_\_:返回数据集的大小;
- \_\_getitem\_\_:根据索引返回对应的数据。
在实际使用中,我们可以根据需要修改这些类和方法,以适应不同的数据集和模型。
相关推荐
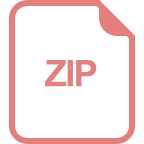
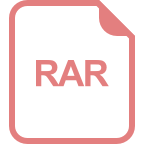
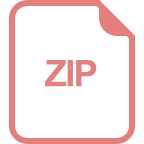















