python把array of float64中的空值替换为0
时间: 2024-03-16 09:47:17 浏览: 118
你可以使用NumPy库中的`nan_to_num()`函数将数组中的空值(NaN)替换为0。以下是一个示例代码:
```python
import numpy as np
my_array = np.array([1.0, np.nan, 2.0, np.nan, 3.0, np.nan])
my_array = np.nan_to_num(my_array)
print(my_array)
```
输出结果:
```
[1. 0. 2. 0. 3. 0.]
```
这将替换数组中的空值为0。如果你想替换为其他的值,只需要将`nan_to_num()`函数的第二个参数设置为你想要的值即可。例如,如果你想将空值替换为-1,可以使用以下代码:
```python
my_array = np.array([1.0, np.nan, 2.0, np.nan, 3.0, np.nan])
my_array = np.nan_to_num(my_array, nan=-1)
print(my_array)
```
输出结果:
```
[ 1. -1. 2. -1. 3. -1.]
```
这将替换数组中的空值为-1。
相关问题
D:\pythonProject_ecg_analyzation\GetBeats.py:32: VisibleDeprecationWarning: Creating an ndarray from ragged nested sequences (which is a list-or-tuple of lists-or-tuples-or ndarrays with different lengths or shapes) is deprecated. If you meant to do this, you must specify 'dtype=object' when creating the ndarray. beat = np.array([beat]).astype(float) TypeError: only size-1 arrays can be converted to Python scalars The above exception was the direct cause of the following exception: Traceback (most recent call last): File "D:\pythonProject_ecg_analyzation\main.py", line 22, in <module> GetBeats.getbeats(r_peaks, ecg_voltage, user_number) File "D:\pythonProject_ecg_analyzation\GetBeats.py", line 32, in getbeats beat = np.array([beat]).astype(float) ValueError: setting an array element with a sequence.怎么改
这个错误提示是因为在将一个列表转换为 ndarray 时,列表中有嵌套的子列表,而这些子列表的长度不同,导致无法转换。解决方法是将所有的子列表长度都设置为相同的长度,可以在短的子列表后面加上一些空值或者删除多余的数据。
另外,如果你只是想将一个列表转换为 ndarray,可以使用 np.asarray() 函数,它可以自动处理嵌套列表的情况。例如:
```
beat = np.asarray(beat, dtype=float)
```
这行代码会将列表 beat 转换为 ndarray,并将数据类型设置为 float。如果列表中包含非数字的元素,转换时会抛出异常。
对点云数据体素化降采样,黑色体素至少包含一个点,值是该体素中所有点的平均位置;白色体素表示空值,值为0,然后再对体素化后的点云数据实施细化算法获得骨架。用python代码实现
要实现点云数据的体素化降采样,并且对降采样后的体素化数据进行细化算法以获取骨架,您可以使用以下Python代码:
```python
import numpy as np
from scipy.ndimage import morphology
def voxel_downsampling(point_cloud, voxel_size):
# 计算点云数据在每个维度上的最小和最大值
min_coords = np.min(point_cloud, axis=0)
max_coords = np.max(point_cloud, axis=0)
# 计算体素网格的尺寸
voxel_grid_size = np.ceil((max_coords - min_coords) / voxel_size).astype(int)
# 创建空的体素网格
voxel_grid = np.zeros(voxel_grid_size, dtype=bool)
# 将点云数据映射到体素网格中
voxel_coords = ((point_cloud - min_coords) / voxel_size).astype(int)
voxel_grid[tuple(voxel_coords.T)] = True
return voxel_grid
def skeletonize_voxel(voxel_data):
# 定义细化算法的核心函数
def thinning(image):
skeleton = image.copy()
done = False
while not done:
eroded = morphology.binary_erosion(image)
temp = morphology.binary_dilation(eroded)
temp = np.subtract(image, temp, out=image)
np.logical_or(skeleton, temp, out=skeleton)
image = eroded.copy()
if np.sum(image) == 0:
done = True
return skeleton
# 应用细化算法
skeleton_voxel = thinning(voxel_data)
return skeleton_voxel
def calculate_voxel_average_position(point_cloud, voxel_grid, voxel_size):
# 计算每个体素中点的平均位置
voxel_average_positions = np.zeros_like(voxel_grid, dtype=float)
for voxel_idx in np.ndindex(*voxel_grid.shape):
if voxel_grid[voxel_idx]:
voxel_points = point_cloud[(point_cloud / voxel_size).astype(int) == voxel_idx]
voxel_average_positions[voxel_idx] = np.mean(voxel_points, axis=0)
return voxel_average_positions
# 示例用法
point_cloud = np.array([[1, 2, 3], [4, 5, 6], [7, 8, 9]]) # 假设这是您的点云数据
voxel_size = 1.0 # 体素大小
# 进行体素化降采样
voxel_grid = voxel_downsampling(point_cloud, voxel_size)
# 计算体素中点的平均位置
voxel_average_positions = calculate_voxel_average_position(point_cloud, voxel_grid, voxel_size)
# 进行细化算法
skeleton = skeletonize_voxel(voxel_grid)
print("Voxel Average Positions:")
print(voxel_average_positions)
print("Skeleton Voxel:")
print(skeleton)
```
在上面的代码中,`voxel_downsampling` 函数用于将点云数据进行体素化降采样,`calculate_voxel_average_position` 函数用于计算每个体素中点的平均位置,`skeletonize_voxel` 函数用于对体素化数据进行细化算法。
希望这能帮助到您!如果您有任何其他问题,请随时提问。
阅读全文
相关推荐
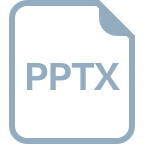
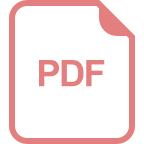
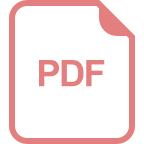
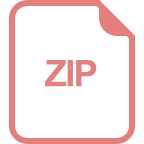
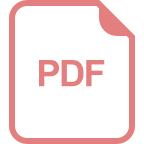
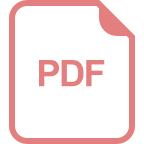
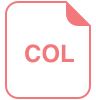
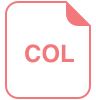
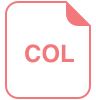
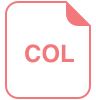
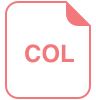
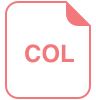
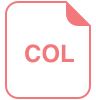
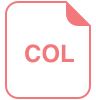

