st-dbscan算法原理代码
时间: 2023-09-06 20:12:42 浏览: 94
ST-DBSCAN算法是基于DBSCAN算法的扩展,用于空间数据中的聚类。它在DBSCAN的基础上增加了时间维度,使得可以识别和处理时空数据中的聚类。其算法流程如下:
1. 对于每一个数据点p,找到与其距离在ε内的所有点,并将这些点放入集合N(p)中。
2. 如果p是核心点,即N(p)中包含至少MinPts个点,则p成为一个种子点,将其放入当前聚类集合C中,并将N(p)中的所有点都加入C中。
3. 对于N(p)中的每个点q,如果q是一个核心点并且还没有被分配到任何一个聚类集合中,则将其加入C中,并且将N(q)中的所有点都加入C中。
4. 重复步骤2和3,直到C中的所有点都被分配到一个聚类集合中。
5. 对于下一个未被分配到任何聚类集合中的点,重复步骤2-4。
ST-DBSCAN算法可以简单地扩展为将时间维度考虑进去。具体地,我们可以将每个数据点p表示为(p.x, p.y, p.t),其中p.x和p.y表示空间坐标,p.t表示时间坐标。我们可以根据空间距离和时间距离来计算点之间的距离,进而进行聚类。
下面是ST-DBSCAN算法的Python实现代码:
```python
from collections import defaultdict
from typing import List, Tuple
def st_dbscan(points: List[Tuple[float, float, float]], epsilon: float, min_pts: int, tau: float) -> List[List[Tuple[float, float, float]]]:
"""
ST-DBSCAN algorithm implementation.
:param points: A list of points, where each point is a tuple of (x, y, t).
:param epsilon: The maximum distance between two points to be considered as neighbors.
:param min_pts: The minimum number of points required to form a dense region.
:param tau: The maximum time difference between two points to be considered as neighbors.
:return: A list of clusters, where each cluster is a list of points.
"""
clusters = []
visited = set()
core_points = set()
neighbor_points = defaultdict(set)
for i, point1 in enumerate(points):
if point1 in visited:
continue
visited.add(point1)
neighbor_points[i] = set()
neighbors = get_neighbors(points, i, epsilon, tau)
if len(neighbors) >= min_pts:
core_points.add(i)
for j in neighbors:
neighbor_points[i].add(j)
neighbor_points[j].add(i)
for i in core_points:
if i not in visited:
cluster = set()
visited.add(i)
cluster.add(points[i])
neighbors = neighbor_points[i]
while neighbors:
j = neighbors.pop()
if j not in visited:
visited.add(j)
cluster.add(points[j])
if j in core_points:
neighbors |= neighbor_points[j]
clusters.append(list(cluster))
return clusters
def get_neighbors(points: List[Tuple[float, float, float]], i: int, epsilon: float, tau: float) -> List[int]:
"""
Get all the neighbors of point i.
:param points: A list of points, where each point is a tuple of (x, y, t).
:param i: The index of the point.
:param epsilon: The maximum distance between two points to be considered as neighbors.
:param tau: The maximum time difference between two points to be considered as neighbors.
:return: A list of indices of the neighbors.
"""
neighbors = []
for j, point2 in enumerate(points):
if i == j:
continue
distance = ((point2[0] - points[i][0]) ** 2 +
(point2[1] - points[i][1]) ** 2) ** 0.5
time_diff = abs(point2[2] - points[i][2])
if distance <= epsilon and time_diff <= tau:
neighbors.append(j)
return neighbors
```
其中,输入参数points是一个由元组组成的列表,每个元组表示一个点的坐标和时间;epsilon和min_pts分别是DBSCAN算法中的两个参数;tau表示时间距离的阈值。输出是一个列表,其中每个元素都是一个列表,表示一个聚类集合中的所有点。
相关推荐
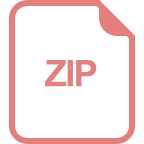
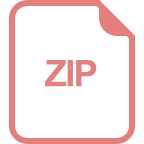
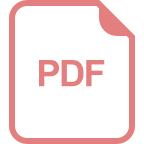
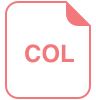
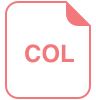
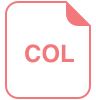
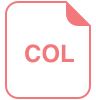










