as-exploits
时间: 2024-01-28 08:01:40 浏览: 100
as-exploits是指针对计算机系统中存在的漏洞或弱点进行攻击和利用的行为。这种行为可能会导致系统崩溃、信息泄漏、数据丢失甚至系统被恶意控制。通常情况下,攻击者会利用find漏洞或弱点,编写特定的代码或程序来利用这些漏洞,然后用这些程序对系统进行攻击。
as-exploits可能会造成严重的安全问题,因为被攻击者通常无法察觉到这种攻击。一旦系统被攻击成功,攻击者可能会窃取敏感信息,篡改或破坏数据,甚至植入恶意软件。因此,as-exploits对于保护计算机系统的安全构成了威胁。
为了减少as-exploits的发生,用户和管理员应该定期更新系统和软件,及时修补已知漏洞。此外,还需要加强对系统的监控和安全防护,以及对用户进行安全意识教育,避免点击恶意链接或下载未经验证的软件。
总之,as-exploits是一种危害计算机系统安全的行为,需要采取有效的措施来预防和应对这种威胁。只有通过大家共同努力,才能有效地保护计算机系统的安全。
相关问题
Abstract— Image nonlocal self-similarity (NSS) property has been widely exploited via various sparsity models such as joint sparsity (JS) and group sparse coding (GSC). However, the existing NSS-based sparsity models are either too restrictive, e.g., JS enforces the sparse codes to share the same support, or too general, e.g., GSC imposes only plain sparsity on the group coefficients, which limit their effectiveness for modeling real images. In this paper, we propose a novel NSS-based sparsity model, namely, low-rank regularized group sparse coding (LR-GSC), to bridge the gap between the popular GSC and JS. The proposed LR-GSC model simultaneously exploits the sparsity and low-rankness of the dictionary-domain coefficients for each group of similar patches. An alternating minimization with an adaptive adjusted parameter strategy is developed to solve the proposed optimization problem for different image restoration tasks, including image denoising, image deblocking, image inpainting, and image compressive sensing. Extensive experimental results demonstrate that the proposed LR-GSC algorithm outperforms many popular or state-of-the-art methods in terms of objective and perceptual metrics.翻译
摘要—图像的非局部自相似性(NSS)属性已经被广泛应用于各种稀疏模型中,例如联合稀疏(JS)和群组稀疏编码(GSC)。然而,现有的基于NSS的稀疏模型要么太过严格,例如JS强制使稀疏编码共享相同的支持,要么太过通用,例如GSC仅对群组系数施加简单的稀疏性,这限制了它们对实际图像建模的有效性。本文提出了一种新的NSS-based稀疏模型,即低秩正则化群组稀疏编码(LR-GSC),以弥合流行的GSC和JS之间的差距。所提出的LR-GSC模型同时利用了每组相似补丁的字典域系数的稀疏性和低秩性。开发了一种带有自适应调整参数策略的交替最小化方法,用于解决不同的图像恢复任务,包括图像去噪、图像去块、图像修复和图像压缩感知。广泛的实验结果表明,所提出的LR-GSC算法在客观和感知度量方面优于许多流行或最先进的方法。
The RGB images have three color channels (24-bit image), but the grayscale image had only a single grayscale image (8-bit image). In our previous experiment, we normalized the images of the pre- trained network by each color channel separately. This approach lost the information provided by the other two channels. Here, we used the same grayscale LDCT image for each channel to make it somewhat analogous to an RGB image for the CNN. Doing so engages all the weights and exploits all the learned knowledge when extracting features from the pretrained net- work. Since the images experimented with were smaller than the required input size of the pretrained CNN (224 × 224), we used bicubic interpolation for resizing. The dimension of a deep feature vector extracted for each image was 4096. The features were the output of the last fully connected layer (the full 2 layer as shown in Table 2) before the output layer in an ImageNet pretrained CNN. The architectures and para 解释
这段话主要是关于一个实验中的图像处理和深度学习网络的使用。该实验中使用了一个预训练的卷积神经网络(CNN)来提取图像的特征,以用于后续的任务。由于原始图像是RGB图像,而预训练网络是对彩色图像进行训练的,因此将灰度图像复制三份,以便处理成类似于RGB图像的形式,以便充分利用预训练网络的知识。因为图像的大小比预训练网络的输入大小要小,所以使用了双三次插值的方法进行图像的缩放。最后,提取了每个图像的深度特征向量,其维度为4096,这些特征是在ImageNet预训练CNN的输出层之前的最后一个全连接层(即表2中的完整2层)的输出结果。
阅读全文
相关推荐
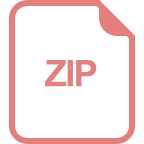
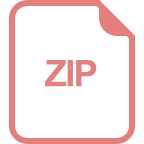
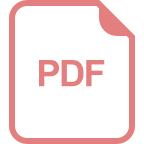
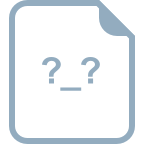
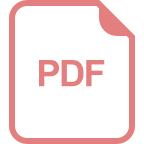
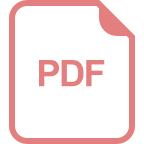
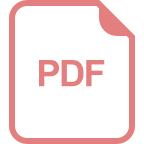
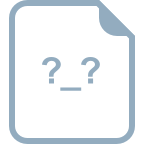
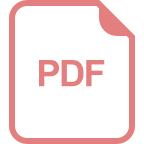
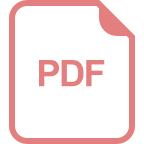
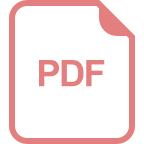
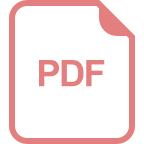
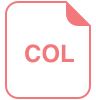