上文中data_load函数
时间: 2024-05-14 21:15:12 浏览: 8
的实现如下:
```python
def data_load(file_path):
data = []
with open(file_path, 'r') as f:
for line in f:
line = line.strip().split(',')
data.append(line)
return data
```
这个函数的作用是从指定的文件中读取数据,并返回一个列表,其中每个元素是一条数据,以列表形式存储。在函数内部,我们打开文件,并循环读取每一行。每一行的数据是一个字符串,我们先去掉两端的空格,然后使用`split()`方法将其按照逗号分隔,得到一个列表,将其添加到`data`列表中。最后,我们返回`data`列表。
相关问题
上文中kube_node_labels的值是从哪里取得的
kube_node_labels是Kubernetes集群中节点的标签(labels)。这些标签可以在创建节点时指定,也可以在以后的时间添加或修改。在Kubernetes中,标签用于标识节点的特征,例如硬件配置、地理位置、业务用途等等。您可以使用kubectl命令行工具来查看和管理节点标签。例如,使用以下命令可以查看节点的标签:
```
kubectl get nodes --show-labels
```
这将显示所有节点及其标签列表。您可以使用标签选择器来筛选具有特定标签的节点,并将它们用于Kubernetes中的不同操作,例如调度Pod到特定的节点。
上文提到的__init__()方法是什么
在Python中,`__init__()`是一种特殊的方法,也称为构造方法或初始化方法,用于在创建对象时初始化对象的属性。当创建一个类的实例时,Python会自动调用该类的`__init__()`方法,从而完成对象的初始化过程。
在Platypus库中,`Problem`类中定义了`__init__()`方法,用于初始化多目标优化问题的属性。在这个方法中,用户需要指定决策变量个数、目标函数个数、约束条件个数等问题相关的参数,同时还需要定义决策变量和约束条件的类型和取值范围等信息。
例如,在上述代码中,我们通过继承`Problem`类并重写`__init__()`方法来定义自己的多目标优化问题。在`__init__()`方法中,我们调用了父类的`__init__()`方法,并传递了参数`2`和`2`,表示我们的问题有两个决策变量和两个目标函数。然后,我们使用`self.types[:] = Real(0, 10)`来定义决策变量的取值范围为`[0,10]`,这里使用了`Real`类型表示实数型变量。最后,我们可以使用`self.constraints[:] = ">=0"`来定义约束条件的范围,这里表示所有的约束条件都应该大于等于0。
总之,`__init__()`方法是一个非常重要的方法,在Platypus库中,用户需要根据自己的问题需求和算法实现来进行调整和优化。
相关推荐
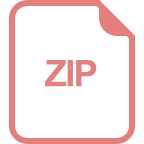
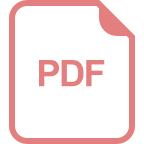
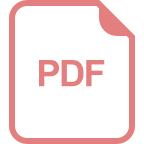












