使用k近邻回归算法,对波士顿房价数据进行预测,并画出真实值与预测值的图像对比图python
时间: 2024-02-24 10:59:35 浏览: 21
好的,我可以为您提供代码实现。首先,我们需要加载波士顿房价数据集,并将其分为训练集和测试集。然后,我们使用K近邻回归算法进行预测,并计算其均方误差(MSE)和决定系数(R2)来评估模型的性能。最后,我们将预测值和真实值绘制在一个图像上进行比较。
下面是实现代码:
```python
# 导入需要的库
from sklearn.datasets import load_boston
from sklearn.model_selection import train_test_split
from sklearn.neighbors import KNeighborsRegressor
from sklearn.metrics import mean_squared_error, r2_score
import matplotlib.pyplot as plt
# 加载波士顿房价数据集
boston = load_boston()
# 将数据集分为训练集和测试集
X_train, X_test, y_train, y_test = train_test_split(boston.data, boston.target, test_size=0.2, random_state=42)
# 定义K近邻回归模型
knn = KNeighborsRegressor(n_neighbors=5)
# 训练模型
knn.fit(X_train, y_train)
# 预测测试集的房价
y_pred = knn.predict(X_test)
# 计算均方误差和决定系数
mse = mean_squared_error(y_test, y_pred)
r2 = r2_score(y_test, y_pred)
print("MSE:", mse)
print("R2:", r2)
# 绘制真实值和预测值的比较图像
plt.scatter(y_test, y_pred)
plt.plot([y_test.min(), y_test.max()], [y_test.min(), y_test.max()], '--', color='red')
plt.xlabel('True Values')
plt.ylabel('Predictions')
plt.show()
```
运行代码后,会输出MSE和R2的值,并显示真实值和预测值的比较图像。您可以根据自己的需要进行调整和优化。
相关推荐
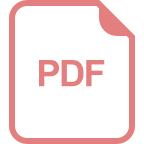
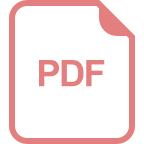
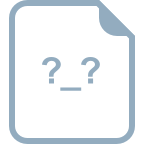














