for param, param_g in zip(params[:-self.layer_idx], params_g[:-self.layer_idx]): param.data = param_g.data.clone()啥意思
时间: 2024-01-18 10:02:36 浏览: 122
这段代码是用来将梯度更新到模型参数中的。具体来说,`params`列表中存储了模型的参数,`params_g`列表中存储了相应参数的梯度。代码中的`zip`函数用于将这两个列表中对应位置的元素一一对应起来,然后遍历这个对应的元素对。在遍历过程中,`param`表示模型参数,`param_g`表示该参数的梯度。`param.data`是参数的值,用`param_g.data.clone()`表示该参数梯度的值,即先将梯度的值克隆一份,然后再更新到参数的值中。这样,就完成了将梯度更新到参数的操作。
相关问题
def __init__(self, sess, state_dim, learning_rate): self.sess = sess self.s_dim = state_dim self.lr_rate = learning_rate # Create the critic network self.inputs, self.out = self.create_critic_network() # Get all network parameters self.network_params = \ tf.compat.v1.get_collection(tf.compat.v1.GraphKeys.TRAINABLE_VARIABLES, scope='critic') # Set all network parameters self.input_network_params = [] for param in self.network_params: self.input_network_params.append( tf.compat.v1.placeholder(tf.float32, shape=param.get_shape())) self.set_network_params_op = [] for idx, param in enumerate(self.input_network_params): self.set_network_params_op.append(self.network_params[idx].assign(param)) # Network target目标 V(s) self.td_target = tf.compat.v1.placeholder(tf.float32, [None, 1]) # Temporal Difference, will also be weights for actor_gradients时间差异,也将是actor_gradients的权重 self.td = tf.subtract(self.td_target, self.out) # Mean square error均方误差 self.loss = tflearn.mean_square(self.td_target, self.out) # Compute critic gradient计算临界梯度 self.critic_gradients = tf.gradients(self.loss, self.network_params) # Optimization Op self.optimize = tf.compat.v1.train.RMSPropOptimizer(self.lr_rate). \ apply_gradients(zip(self.critic_gradients, self.network_params))请对这段代码每句进行注释
# 定义一个类,表示 Critic 网络
class CriticNetwork(object):
def __init__(self, sess, state_dim, learning_rate):
# 初始化 Critic 网络的一些参数
self.sess = sess
self.s_dim = state_dim
self.lr_rate = learning_rate
# 创建 Critic 网络
self.inputs, self.out = self.create_critic_network()
# 获取 Critic 网络中所有的参数
self.network_params = tf.compat.v1.get_collection(tf.compat.v1.GraphKeys.TRAINABLE_VARIABLES, scope='critic')
# 定义一个占位符,表示 Critic 网络的输入参数
self.input_network_params = []
for param in self.network_params:
self.input_network_params.append(tf.compat.v1.placeholder(tf.float32, shape=param.get_shape()))
# 定义一个操作,用于设置 Critic 网络的所有参数
self.set_network_params_op = []
for idx, param in enumerate(self.input_network_params):
self.set_network_params_op.append(self.network_params[idx].assign(param))
# 定义一个占位符,表示 Critic 网络的目标输出
self.td_target = tf.compat.v1.placeholder(tf.float32, [None, 1])
# 计算 Critic 网络的 Temporal Difference
self.td = tf.subtract(self.td_target, self.out)
# 定义 Critic 网络的损失函数,使用均方误差
self.loss = tflearn.mean_square(self.td_target, self.out)
# 计算 Critic 网络的梯度
self.critic_gradients = tf.gradients(self.loss, self.network_params)
# 定义 Critic 网络的优化器
self.optimize = tf.compat.v1.train.RMSPropOptimizer(self.lr_rate).apply_gradients(zip(self.critic_gradients, self.network_params))
Nesterov Accelerated Gradient Descent A variant of Stochastic Gradient Descent also considers the penultimate update to include more statistics for optimization: 𝜃̃ =𝜃+𝑣old⋅𝑚𝑡𝑚 𝑣=𝑣old⋅𝑚𝑡𝑚+𝜂⋅(−∂𝐿(𝜃̃ )∂𝜃) 𝜃=𝜃̃ Help me complete the following code based on the above question class NAGD(Optimizer): def __init__(self, params, lr=0.2, momentum=0.9) -> None: super().__init__(params, {'lr': lr, 'momentum': momentum}) self.lr = lr # maybe you need some more code here def step(self): with torch.no_grad(): for i, p in enumerate(self.param_groups[0]['params']): if p.grad is not None: # update parameters ...
Here is the completed code for Nesterov Accelerated Gradient Descent:
```
class NAGD(Optimizer):
def __init__(self, params, lr=0.2, momentum=0.9) -> None:
super().__init__(params, {'lr': lr, 'momentum': momentum})
self.lr = lr
self.momentum = momentum
self.v = {} # initialize velocity as an empty dictionary
for param in self.param_groups[0]['params']:
self.v[param] = torch.zeros_like(param.data) # initialize velocity for each parameter as a tensor of zeros
def step(self):
with torch.no_grad():
for i, p in enumerate(self.param_groups[0]['params']):
if p.grad is not None:
# update velocity
self.v[p] = self.momentum * self.v[p] + self.lr * (-p.grad)
# compute Nesterov update
p_nesterov = p.data - self.momentum * self.v[p]
# update parameters
p.data.copy_(p_nesterov)
```
In the above code, we initialize the velocity `self.v` for each parameter as a tensor of zeros in the constructor. Then, in the `step()` method, we first update the velocity using the gradient of the current parameter value. Next, we compute the Nesterov update by subtracting the momentum-scaled velocity from the current parameter value. Finally, we update the parameter with the Nesterov update.
阅读全文
相关推荐









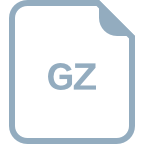






