To do multiple steps, we implement a function the_loop that iterates over a dataloader. It should do a training step per batch for epochs. After one epoch, the loss on the validation set should be calculated.帮我根据这个要求补充完整下面代码中。。。的部分。 def the_loop(net, optimizer, train_loader, val_loader=None, epochs=None, swa_model=None, swa_start=5): if epochs is None: raise Exception("a training duration must be given: set epochs") log_iterval = 1 running_mean = 0. loss = Tensor([0.]).cuda() losses = [] val_losses = [] states = [] i, j = 0, 0 pbar = tqdm(train_loader, desc=f"epoch {i}", postfix={"loss": loss.item(), "step": j}) for i in range(epochs): running_mean = 0. j = 0 pbar.set_description(f"epoch {i}") pbar.refresh() pbar.reset() for j, batch in enumerate(train_loader): # implement training step by # - appending the current states to `states` # - doing a training_step # - appending the current loss to the `losses` list # - update the running_mean for logging ... if j % log_iterval == 0 and j != 0: pbar.set_postfix({"loss": running_mean.item(), "step": j}) running_mean = 0. pbar.update() if i > swa_start and swa_model is not None: swa_model.update_parameters(net) if val_loader is not None: # evaluate the current net on the validation data loader and # collect all losses in the ´val_loss´ list ... pbar.refresh() if val_loader is not None: return losses, states, val_losses return losses, states
时间: 2024-02-10 14:16:51 浏览: 80
Here is the completed code:
```python
def the_loop(net, optimizer, train_loader, val_loader=None, epochs=None, swa_model=None, swa_start=5):
if epochs is None:
raise Exception("a training duration must be given: set epochs")
log_iterval = 1
running_mean = 0.
loss = torch.Tensor([0.]).cuda()
losses = []
val_losses = []
states = []
i, j = 0, 0
pbar = tqdm(train_loader, desc=f"epoch {i}", postfix={"loss": loss.item(), "step": j})
for i in range(epochs):
running_mean = 0.
j = 0
pbar.set_description(f"epoch {i}")
pbar.refresh()
pbar.reset()
for j, batch in enumerate(train_loader):
# implement training step by
# - appending the current states to `states`
# - doing a training_step
# - appending the current loss to the `losses` list
# - update the running_mean for logging
states.append(net.state_dict())
optimizer.zero_grad()
output = net(batch)
batch_loss = loss_function(output, batch.target)
batch_loss.backward()
optimizer.step()
losses.append(batch_loss.item())
running_mean = (running_mean * j + batch_loss.item()) / (j + 1)
if j % log_iterval == 0 and j != 0:
pbar.set_postfix({"loss": running_mean, "step": j})
running_mean = 0.
pbar.update()
if i > swa_start and swa_model is not None:
swa_model.update_parameters(net)
if val_loader is not None:
val_loss = 0.
with torch.no_grad():
for val_batch in val_loader:
val_output = net(val_batch)
val_loss += loss_function(val_output, val_batch.target).item()
val_loss /= len(val_loader)
val_losses.append(val_loss)
pbar.refresh()
if val_loader is not None:
return losses, states, val_losses
return losses, states
```
Note that the training step implementation assumes that you have already defined a `loss_function` and that the batch data has an attribute named `target` that contains the ground truth labels.
阅读全文
相关推荐
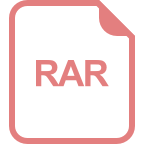
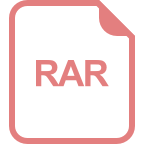
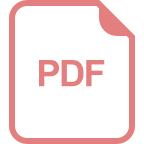
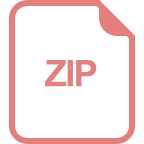
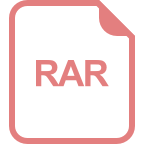
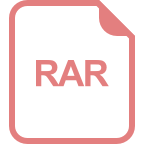
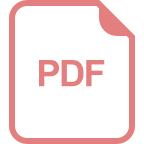
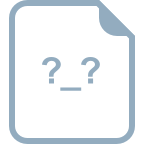
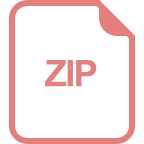
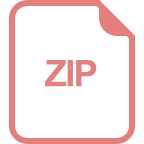
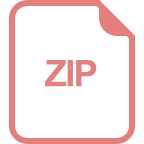
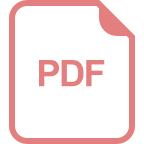
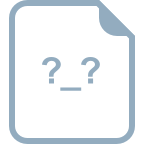
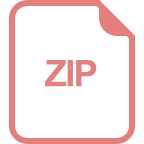
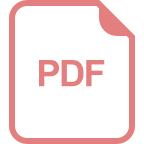
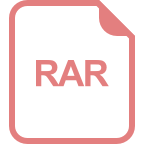