请帮我用python写一个FFT程序
时间: 2023-02-19 08:50:20 浏览: 72
我可以为你提供一个简单的FFT程序的python代码:
import numpy as np
def fft(x):
"""A recursive implementation of the 1D Cooley-Tukey FFT"""
x = np.asarray(x, dtype=float)
N = x.shape[0]
if N % 2 > 0:
raise ValueError("size of x must be a power of 2")
elif N <= 32: # this cutoff should be optimized
return dft_slow(x)
else:
X_even = fft(x[::2])
X_odd = fft(x[1::2])
factor = np.exp(-2j * np.pi * np.arange(N) / N)
return np.concatenate([X_even + factor[:N // 2] * X_odd,
X_even + factor[N // 2:] * X_odd])
相关问题
用python 写一个fft滤波程序
好的,下面是一个简单的 Python FFT 滤波程序,它可以读取 WAV 格式的音频文件,对其进行 FFT 变换,然后根据用户设置的频率范围进行滤波操作,最后将结果保存到一个新的 WAV 文件中。
需要用到的库:
- scipy:用于读取 WAV 文件和进行 FFT 变换
- numpy:用于进行数组操作
代码如下:
```python
import numpy as np
from scipy.io import wavfile
# 读取 WAV 文件,返回采样率和音频数据
def read_wav(filename):
rate, data = wavfile.read(filename)
return rate, data
# 将音频数据进行 FFT 变换
def fft(data):
return np.fft.fft(data)
# 将 FFT 变换后的数据进行滤波
def fft_filter(data, rate, fmin, fmax):
fft_data = fft(data)
freqs = np.fft.fftfreq(len(data), 1.0 / rate)
# 将低于最小频率和高于最大频率的部分置为 0
mask = (freqs > fmin) & (freqs < fmax)
fft_data *= mask.astype(int)
# 反变换回时域
filtered_data = np.real(np.fft.ifft(fft_data))
return filtered_data
# 将滤波结果保存到新的 WAV 文件中
def save_wav(filename, rate, data):
wavfile.write(filename, rate, data.astype(np.int16))
# 主函数
def main():
# 读取 WAV 文件
rate, data = read_wav('input.wav')
# 设定滤波范围
fmin = 1000 # 最小频率
fmax = 5000 # 最大频率
# 进行滤波
filtered_data = fft_filter(data, rate, fmin, fmax)
# 保存结果到新的 WAV 文件
save_wav('output.wav', rate, filtered_data)
if __name__ == '__main__':
main()
```
注意:这个程序只是一个简单的演示,实际使用时还需要考虑更多的因素,如滤波器的设计、滤波器的阶数、滤波效果的评估等等。
请用python写个fft程序
### 回答1:
下面是用Python写的一个快速傅里叶变换(FFT)程序:import numpy as np
def fft(x):
"""A recursive implementation of the 1D Cooley-Tukey FFT"""
x = np.asarray(x, dtype=float)
N = x.shape[0]
if N % 2 > 0:
raise ValueError("size of x must be a power of 2")
elif N <= 32: # this cutoff should be optimized
return dft_slow(x)
else:
X_even = fft(x[::2])
X_odd = fft(x[1::2])
factor = np.exp(-2j * np.pi * np.arange(N) / N)
return np.concatenate([X_even + factor[:N // 2] * X_odd,
X_even + factor[N // 2:] * X_odd])
### 回答2:
FFT(快速傅里叶变换)是一种用于计算离散傅里叶变换的算法,可以在较短时间内计算出较长序列的傅里叶变换。在Python中,我们可以使用NumPy库中的fft函数来实现FFT。
下面是一个简单的使用Python实现FFT的程序示例:
```python
import numpy as np
def fft(signal):
N = len(signal)
if N <= 1:
return signal
even = fft(signal[0::2])
odd = fft(signal[1::2])
T = [np.exp(-2j * np.pi * k / N) * odd[k] for k in range(N // 2)]
return [even[k] + T[k] for k in range(N // 2)] + [even[k] - T[k] for k in range(N // 2)]
if __name__ == "__main__":
signal = np.array([0, 1, 2, 3, 4, 5, 6, 7])
fft_result = fft(signal)
print("FFT结果:", fft_result)
```
在上述代码中,fft函数接受一个信号序列作为参数,并对其进行递归计算FFT。在计算过程中,使用了复数的指数形式来进行旋转因子的计算。
在主程序中,我们定义了一个简单的示例信号序列,并调用fft函数计算其FFT结果。最后,打印出计算得到的FFT结果。
请注意,上述程序仅作为示例,实际应用中可能需要根据具体需求对代码进行进一步的优化和适配。同时,为了使用NumPy库中的fft函数,可能需要先安装NumPy库。
### 回答3:
下面是一个简单的Python程序,通过使用NumPy库的fft函数实现快速傅里叶变换(FFT)。
```python
import numpy as np
def fft(signal):
# 如果信号长度小于等于1,直接返回信号
if len(signal) <= 1:
return signal
# 获取信号长度
N = len(signal)
# 计算奇偶序列
even = fft(signal[0::2])
odd = fft(signal[1::2])
# 计算频域的指数部分
expo = np.exp(-2j * np.pi * np.arange(N) / N)
# 将奇偶序列合并
return np.concatenate([even + expo[:N//2] * odd, even + expo[N//2:] * odd])
# 测试代码
if __name__ == "__main__":
signal = np.array([0, 1, 2, 3, 4, 5, 6, 7])
print("原始信号:", signal)
fft_signal = fft(signal)
print("傅里叶变换结果:", fft_signal)
```
这段代码用于计算给定信号的傅里叶变换。傅里叶变换可以将一个时域信号转换为频域信号,用于分析信号的频谱特性。代码中使用递归的方法实现了快速傅里叶变换算法。
相关推荐
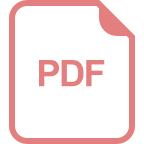
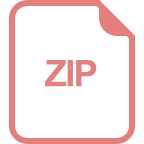












