pytorch实现OhemCrossEntropyLoss
时间: 2023-11-12 17:05:45 浏览: 29
实现 OhemCrossEntropyLoss 可以参考以下步骤:
1. 定义一个继承 Pytorch 自带的 CrossEntropyLoss 的类 OhemCrossEntropyLoss
2. 在 OhemCrossEntropyLoss 类的构造函数中,设置好需要使用的参数,例如阈值 threshold、忽略的类别数 num_ignore、权重因子 alpha 等
3. 在 forward 方法中,计算交叉熵损失,并根据阈值选择保留的样本,即保留 top-k 的概率较大样本和所有正确分类的样本,忽略预测错误的概率较大样本和已经被忽略的类别
4. 根据保留的样本重新计算损失,并乘以权重因子 alpha
5. 返回 OhemCrossEntropyLoss 的结果
具体的实现代码可以参考以下示例:
```python
import torch
import torch.nn as nn
import torch.nn.functional as F
class OhemCrossEntropyLoss(nn.Module):
def __init__(self, threshold, num_ignore, alpha):
super(OhemCrossEntropyLoss, self).__init__()
self.threshold = threshold
self.num_ignore = num_ignore
self.alpha = alpha
self.loss = nn.CrossEntropyLoss(ignore_index=-1, reduction='none')
def forward(self, predict, target):
loss = self.loss(predict, target).view(-1)
sorted_loss, _ = torch.sort(loss, descending=True)
threshold_index = min(len(sorted_loss), int(len(sorted_loss) * self.threshold))
threshold = sorted_loss[threshold_index]
keep_index = loss >= threshold
keep_index &= (target != self.num_ignore)
keep_index |= (target == target.gather(1, predict.argmax(1).unsqueeze(1)).squeeze())
target = target[keep_index]
predict = predict[keep_index]
valid_num = keep_index.sum().item()
if valid_num == 0:
return torch.zeros(1, device=predict.device)
loss = self.loss(predict, target)
label_num = loss.size(0)
weight = torch.zeros(label_num, device=predict.device)
count = torch.zeros(self.num_ignore + 1, device=predict.device)
for idx in range(label_num):
t = target[idx].int().item()
if t != self.num_ignore:
count[t] += 1
for idx in range(label_num):
t = target[idx].int().item()
if t != self.num_ignore:
weight[idx] = 1.0 / (count[t] * self.num_ignore)
weight = (weight * self.alpha + 1) / 2.0
loss = weight * loss
return torch.mean(loss)
```
在使用过程中,可以直接将 OhemCrossEntropyLoss 作为损失函数使用,例如:
```python
criterion = OhemCrossEntropyLoss(threshold=0.7, num_ignore=255, alpha=0.75)
optimizer = torch.optim.Adam(model.parameters(), lr=0.0001)
for epoch in range(100):
for inputs, labels in dataloader:
optimizer.zero_grad()
outputs = model(inputs)
loss = criterion(outputs, labels)
loss.backward()
optimizer.step()
```
这样就可以使用定义好的 OhemCrossEntropyLoss 进行训练了。
相关推荐
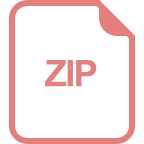














